Visual correspondence-based explanations improve AI robustness and human-AI team accuracy
arxiv(2022)
摘要
Explaining artificial intelligence (AI) predictions is increasingly important and even imperative in many high-stakes applications where humans are the ultimate decision-makers. In this work, we propose two novel architectures of self-interpretable image classifiers that first explain, and then predict (as opposed to post-hoc explanations) by harnessing the visual correspondences between a query image and exemplars. Our models consistently improve (by 1 to 4 points) on out-of-distribution (OOD) datasets while performing marginally worse (by 1 to 2 points) on in-distribution tests than ResNet-50 and a $k$-nearest neighbor classifier (kNN). Via a large-scale, human study on ImageNet and CUB, our correspondence-based explanations are found to be more useful to users than kNN explanations. Our explanations help users more accurately reject AI's wrong decisions than all other tested methods. Interestingly, for the first time, we show that it is possible to achieve complementary human-AI team accuracy (i.e., that is higher than either AI-alone or human-alone), in ImageNet and CUB image classification tasks.
更多查看译文
关键词
visual correspondence,nearest neighbors,optimal transport,explainable AI,xai,human AI team
AI 理解论文
溯源树
样例
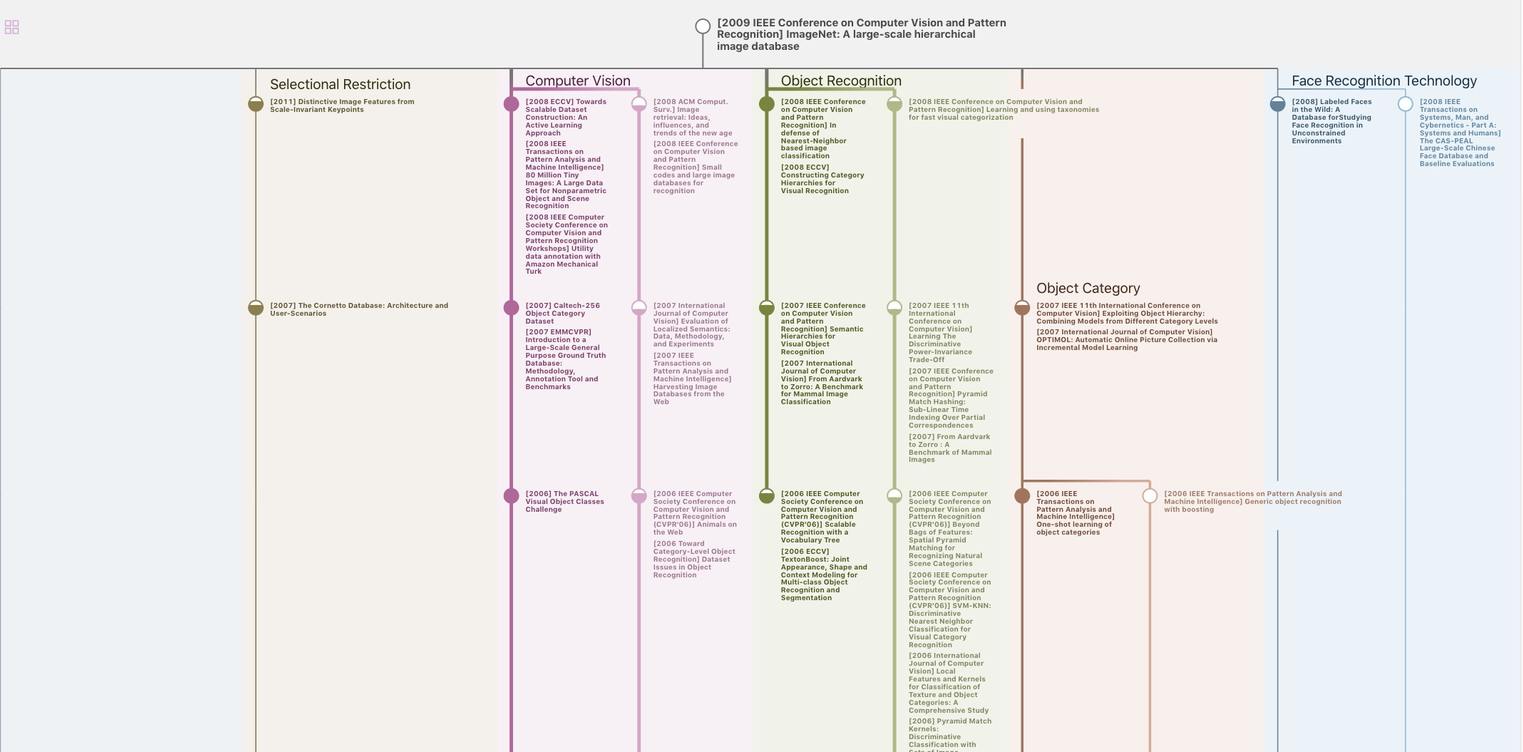
生成溯源树,研究论文发展脉络
Chat Paper
正在生成论文摘要