Developing deep learning algorithms for inferring upstream separatrix density at JET
Nuclear Materials and Energy(2023)
摘要
Predictive and real-time inference capability for the upstream separatrix electron density, ne, sep, is essential for design and control of core-edge integrated plasma scenarios. In this study, both supervised and semi-supervised machine learning algorithms are explored to establish direct mapping as well as indirect compressed representation of the pedestal profiles for predictions and inference of ne, sep. Based on the EUROfusion pedestal database for JET (Frassinetti et al., 2021), a tabular dataset was created, consisting of machine parameters, fraction of ELM cycle, high resolution Thomson scattering profiles of electron density and temperature, and ne, sep for 608 JET shots. Using the tabular dataset, the direct mapping approach provides a mapping of machine parameters and ELM percentage to ne, sep. Through representation learning, a compressed representation of the experimental pedestal electron density and temperature profiles is established. By conditioning the representation with machine control parameters, a probabilistic generative predictive model is established. For prediction, the machine parameters can be used to establish a conditional distribution of the compressed pedestal profiles, and the decoder that is trained as part of the algorithm can be used to decode the compressed representation back to full pedestal profiles. Although, in this work, a proof-of-principle for predicting and inferring ne, sep is given, such a representation learning can be used also for many other applications as the full pedestal profile is predicted. An implementation of this work can be found at https://github.com/fusionby2030/psi_2022.
更多查看译文
关键词
Separatrix,Machine learning,JET,Representation learning
AI 理解论文
溯源树
样例
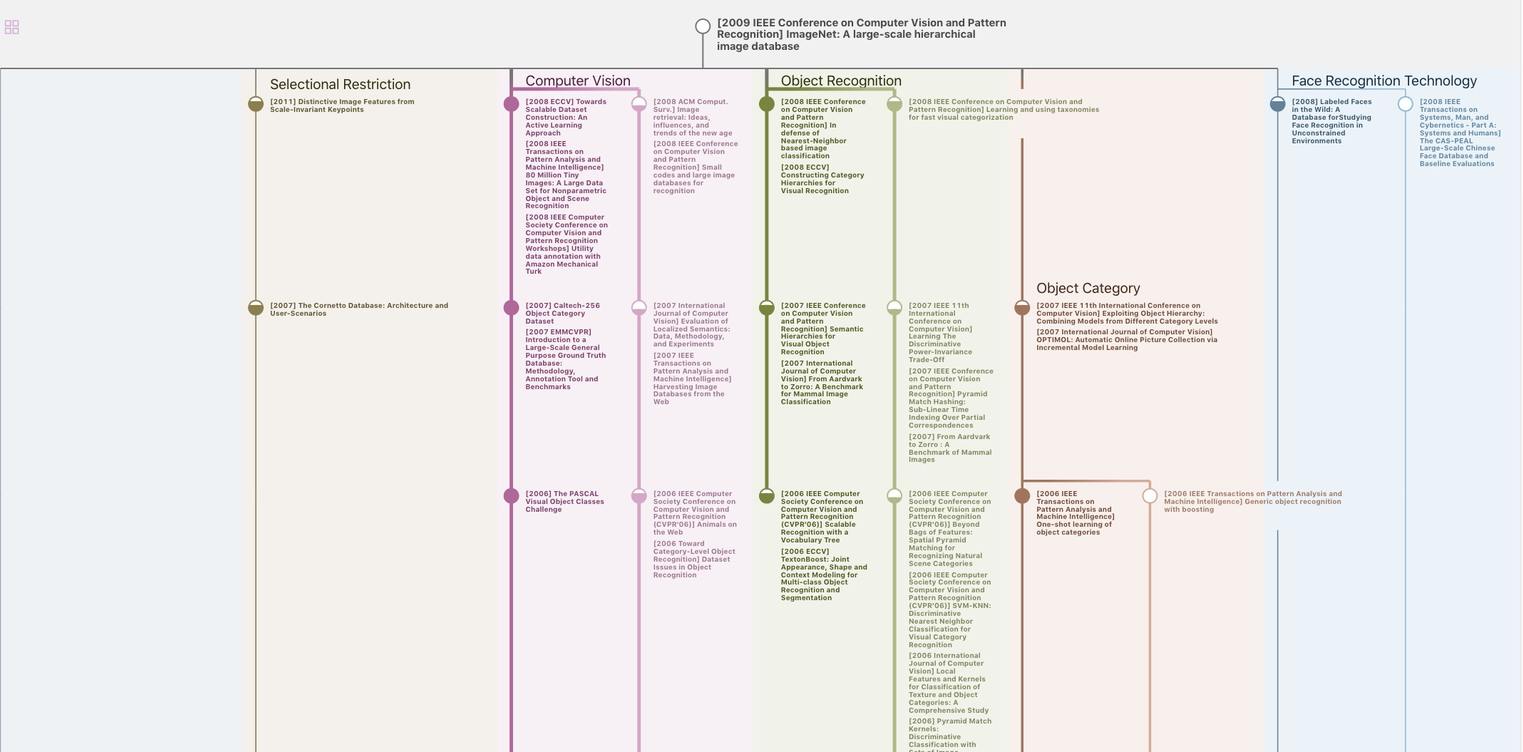
生成溯源树,研究论文发展脉络
Chat Paper
正在生成论文摘要