Detection and classification of pipe defects based on pipe-extended feature pyramid network
Automation in Construction(2022)
摘要
In image-based pipe defect detection research, the effective utilization of the information in the two-dimension (2D) image is directly related to the sampling of the image. The existing inspection methods do not analyze the pipeline imaging but rather directly use the object detection method for defect detection, resulting in a bottleneck problem for the accuracy. In this study, the pipeline imaging was analyzed. It was found that effective sampling of the defect texture within the edge region of the image could improve defect detection accuracy. An image sampling framework, pipe-extended feature pyramid network (P-EFPN), was constructed, and the super-resolution (SR) module was embedded for texture extraction to obtain rich defect texture information and provide image sampling support for pipe defect detection. The defect dataset contains deformation, corrosion, and crack. In the faster region-convolutional neural network (R-CNN) model with Resnet-101 as the backbone, the mean average precision (mAP) of the P-EFPN model was improved by 8.64% compared to the state-of-the-art feature pyramid network (FPN) model. The proposed method improves the accuracy of defect detection by capturing more textures in the edge regions of the image. Compared with existing image sampling methods, the proposed sampling method is more suitable for pipe defect detection.
更多查看译文
关键词
Pipe defects,Underground infrastructure,Textural features,Defects detection,Defects classification
AI 理解论文
溯源树
样例
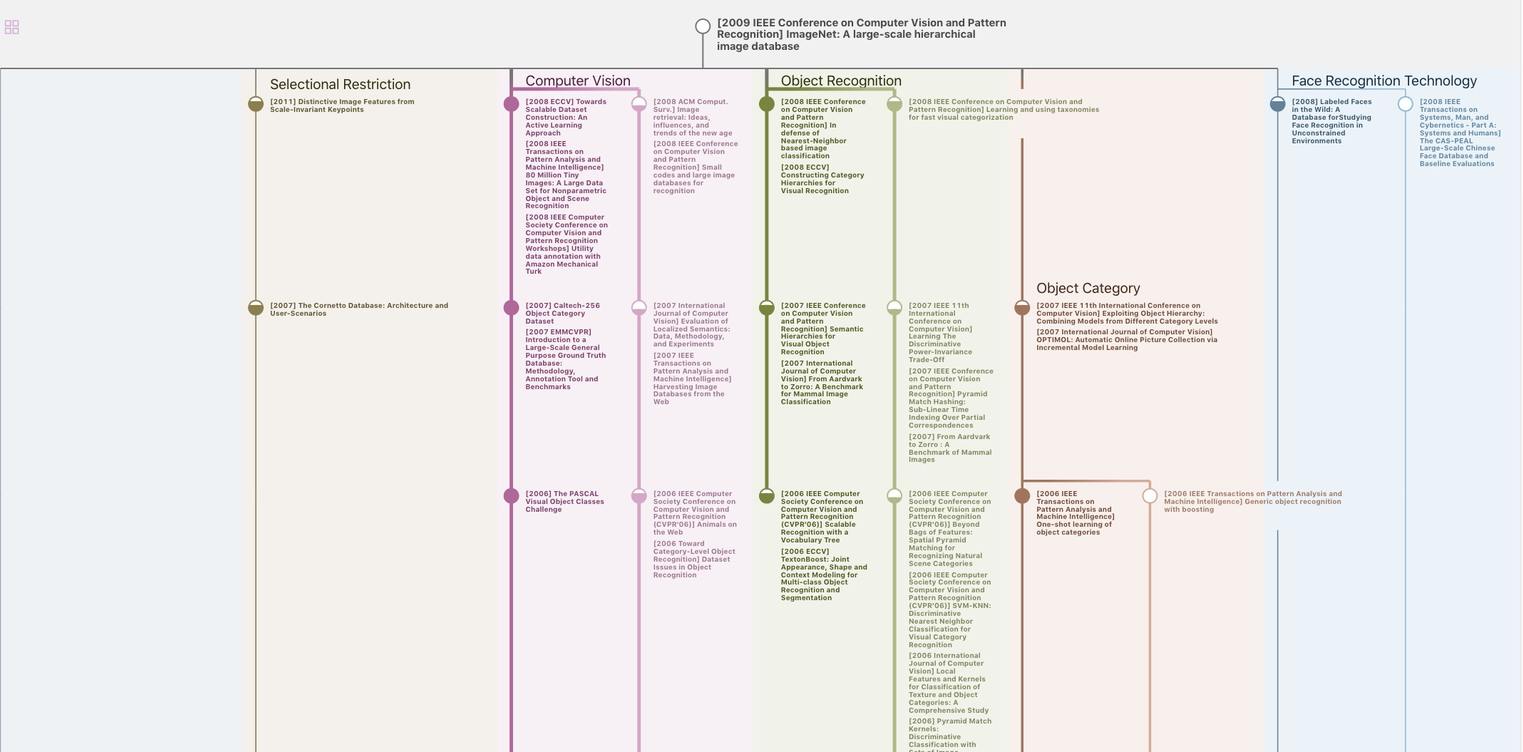
生成溯源树,研究论文发展脉络
Chat Paper
正在生成论文摘要