Understanding the Relation of User and News Representations in Content-Based Neural News Recommendation
arxiv(2022)
摘要
A number of models for neural content-based news recommendation have been proposed. However, there is limited understanding of the relative importances of the three main components of such systems (news encoder, user encoder, and scoring function) and the trade-offs involved. In this paper, we assess the hypothesis that the most widely used means of matching user and candidate news representations is not expressive enough. We allow our system to model more complex relations between the two by assessing more expressive scoring functions. Across a wide range of baseline and established systems this results in consistent improvements of around 6 points in AUC. Our results also indicate a trade-off between the complexity of news encoder and scoring function: A fairly simple baseline model scores well above 68% AUC on the MIND dataset and comes within 2 points of the published state-of-the-art, while requiring a fraction of the computational costs.
更多查看译文
关键词
neural news recommendation,news representations,content-based
AI 理解论文
溯源树
样例
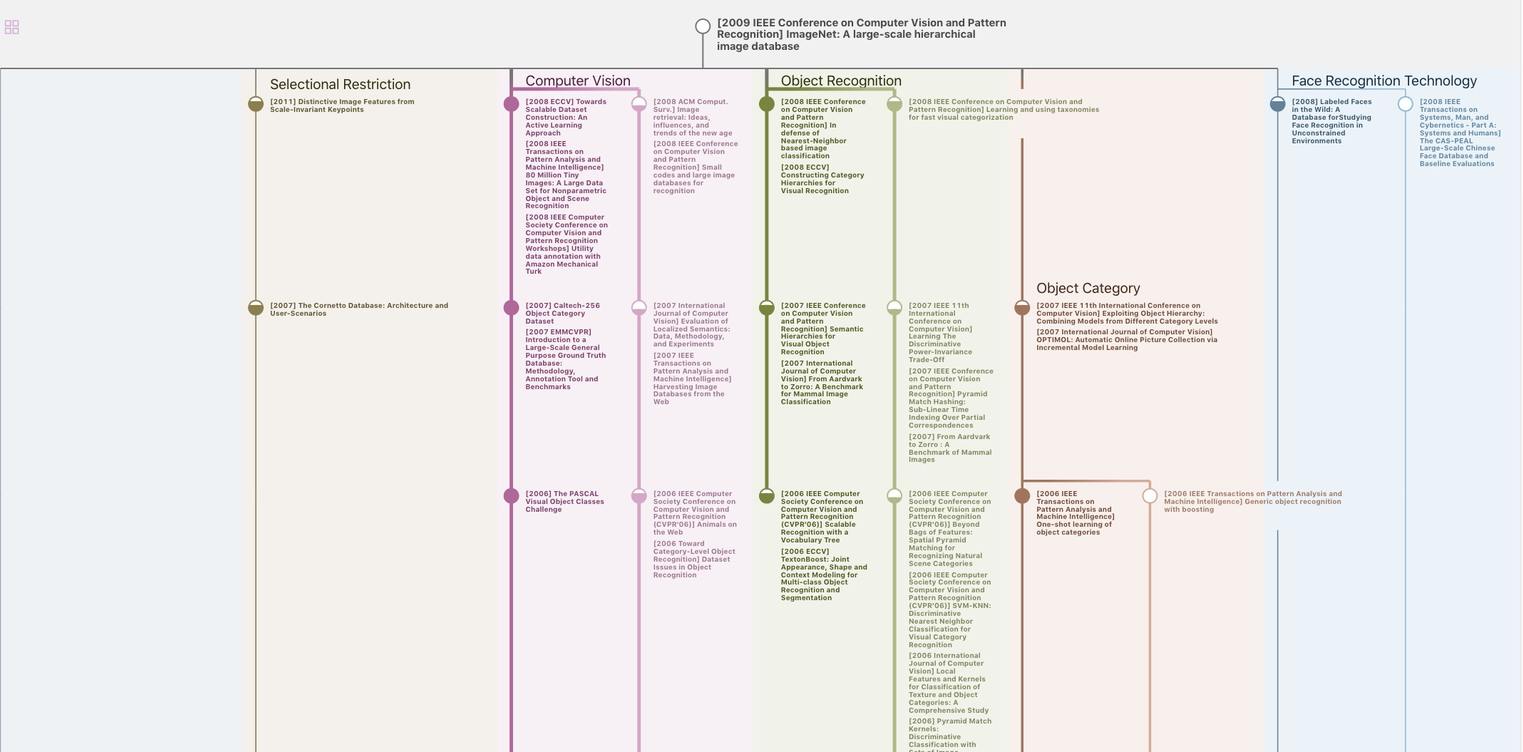
生成溯源树,研究论文发展脉络
Chat Paper
正在生成论文摘要