Towards Unconstrained Audio Splicing Detection and Localization with Neural Networks
arxiv(2023)
摘要
Freely available and easy-to-use audio editing tools make it straightforward to perform audio splicing. Convincing forgeries can be created by combining various speech samples from the same person. Detection of such splices is important both in the public sector when considering misinformation, and in a legal context to verify the integrity of evidence. Unfortunately, most existing detection algorithms for audio splicing use handcrafted features and make specific assumptions. However, criminal investigators are often faced with audio samples from unconstrained sources with unknown characteristics, which raises the need for more generally applicable methods. With this work, we aim to take a first step towards unconstrained audio splicing detection to address this need. We simulate various attack scenarios in the form of post-processing operations that may disguise splicing. We propose a Transformer sequence-to-sequence (seq2seq) network for splicing detection and localization. Our extensive evaluation shows that the proposed method outperforms existing dedicated approaches for splicing detection [3, 10] as well as the general-purpose networks EfficientNet [28] and RegNet [25].
更多查看译文
关键词
unconstrained audio splicing detection,neural networks
AI 理解论文
溯源树
样例
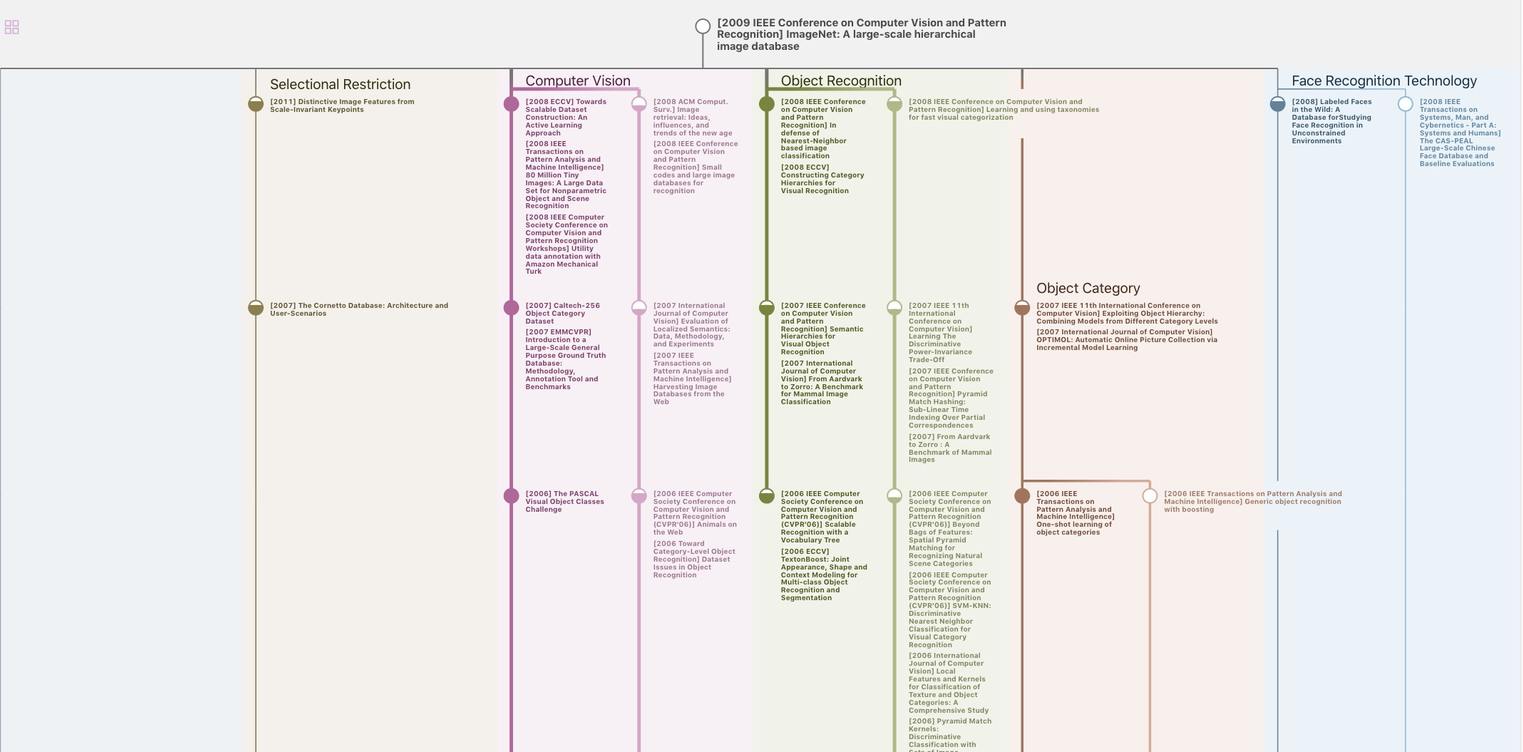
生成溯源树,研究论文发展脉络
Chat Paper
正在生成论文摘要