SYNTA: A novel approach for deep learning-based image analysis in muscle histopathology using photo-realistic synthetic data
arxiv(2022)
摘要
Artificial intelligence (AI), machine learning, and deep learning (DL)
methods are becoming increasingly important in the field of biomedical image
analysis. However, to exploit the full potential of such methods, a
representative number of experimentally acquired images containing a
significant number of manually annotated objects is needed as training data.
Here we introduce SYNTA (synthetic data) as a novel approach for the generation
of synthetic, photo-realistic, and highly complex biomedical images as training
data for DL systems. We show the versatility of our approach in the context of
muscle fiber and connective tissue analysis in histological sections. We
demonstrate that it is possible to perform robust and expert-level segmentation
tasks on previously unseen real-world data, without the need for manual
annotations using synthetic training data alone. Being a fully parametric
technique, our approach poses an interpretable and controllable alternative to
Generative Adversarial Networks (GANs) and has the potential to significantly
accelerate quantitative image analysis in a variety of biomedical applications
in microscopy and beyond.
更多查看译文
关键词
muscle
AI 理解论文
溯源树
样例
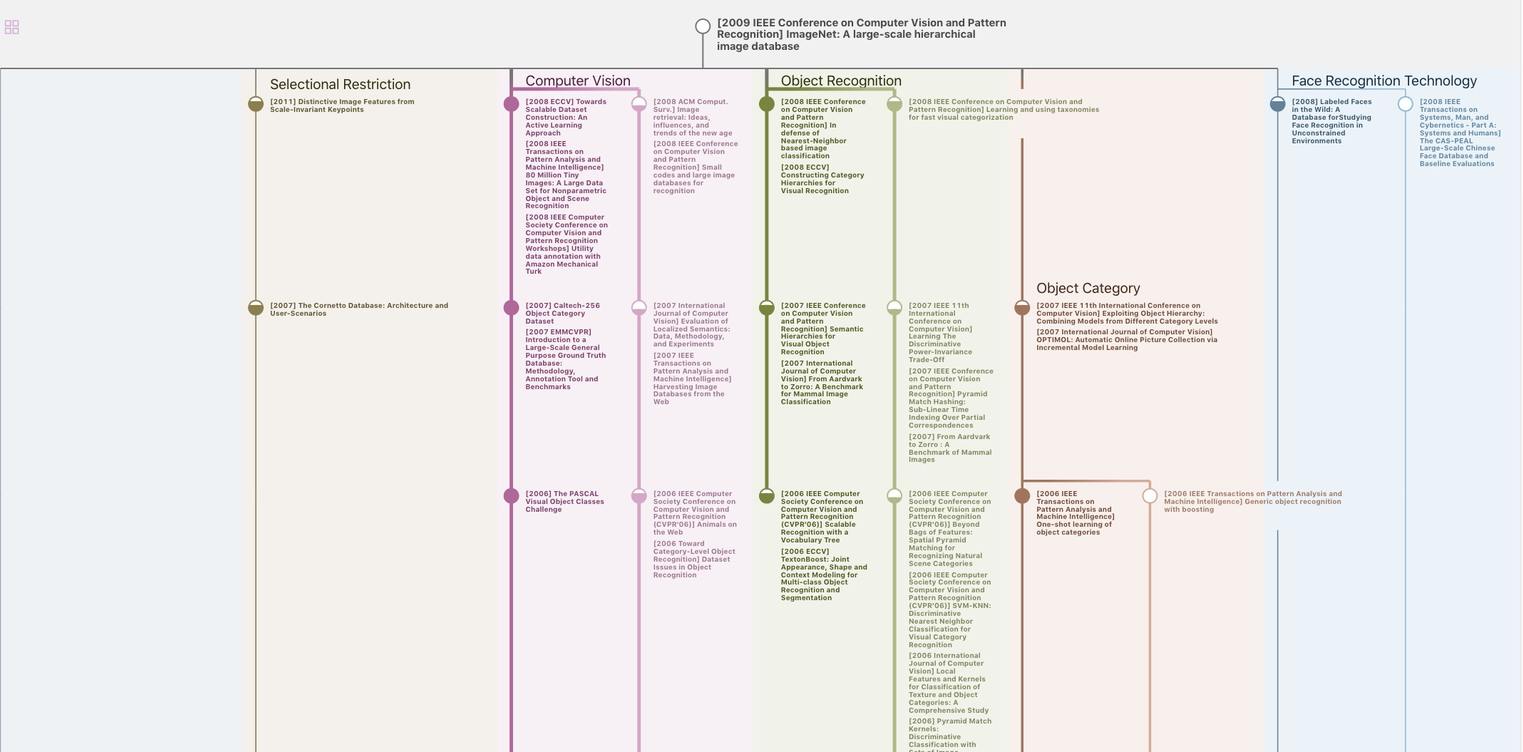
生成溯源树,研究论文发展脉络
Chat Paper
正在生成论文摘要