Artificial Neural Networks for Geothermal Reservoirs: Implications for Oil and Gas Reservoirs
Day 2 Tue, August 02, 2022(2022)
摘要
Abstract Subsurface numerical models take a significant time to build and run. For this reason, the energy industry has been looking towards proxy models that could reduce model computational time. With the advancement of artificial neural network algorithms, building proxy models has become more efficient, and has enabled quick forecasting and quick reservoir management decision-making. In this study, we used a geothermal reservoir to evaluate the suitability of two deep learning algorithms, feed forward neural network and convolutional neural network, for proxy modeling. We used metrics such as the mean square error, losses, number of parameters for the model, and time to run, to compare the two deep learning algorithms. From our study, we determined that the convolutional neural network resulted in less error than the feed forward network and used less hyperparameters. However, the feed forward network was significantly faster than the convolutional neural network. The process of building the proxy model shows how a similar approach can be followed for oil and gas reservoir modeling and demonstrates the feasibility of neural networks in subsurface reservoir modeling and forecasting.
更多查看译文
AI 理解论文
溯源树
样例
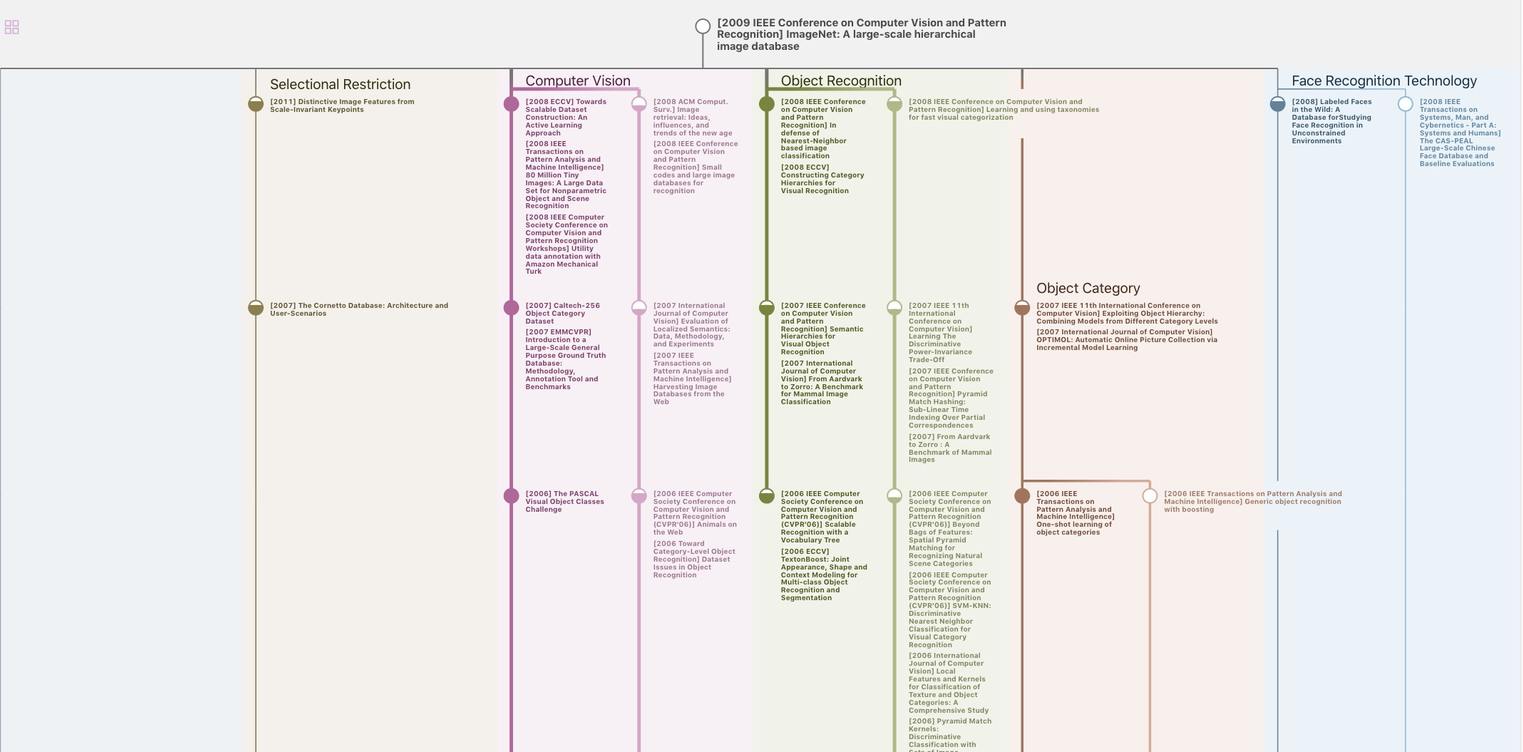
生成溯源树,研究论文发展脉络
Chat Paper
正在生成论文摘要