ObjectFusion: Accurate object-level SLAM with neural object priors
Graphical Models(2022)
摘要
Previous object-level Simultaneous Localization and Mapping (SLAM) approaches still fail to create high quality object-oriented 3D map in an efficient way. The main challenges come from how to represent the object shape effectively and how to apply such object representation to accurate online camera tracking efficiently. In this paper, we provide ObjectFusion as a novel object-level SLAM in static scenes which efficiently creates object-oriented 3D map with high-quality object reconstruction, by leveraging neural object priors. We propose a neural object representation with only a single encoder–decoder network to effectively express the object shape across various categories, which benefits high quality reconstruction of object instance. More importantly, we propose to convert such neural object representation as precise measurements to jointly optimize the object shape, object pose and camera pose for the final accurate 3D object reconstruction. With extensive evaluations on synthetic and real-world RGB-D datasets, we show that our ObjectFusion outperforms previous approaches, with better object reconstruction quality, using much less memory footprint, and in a more efficient way, especially at the object level.
更多查看译文
关键词
Object-Level SLAM,Online Reconstruction,Deep 3D Representation and Reconstruction
AI 理解论文
溯源树
样例
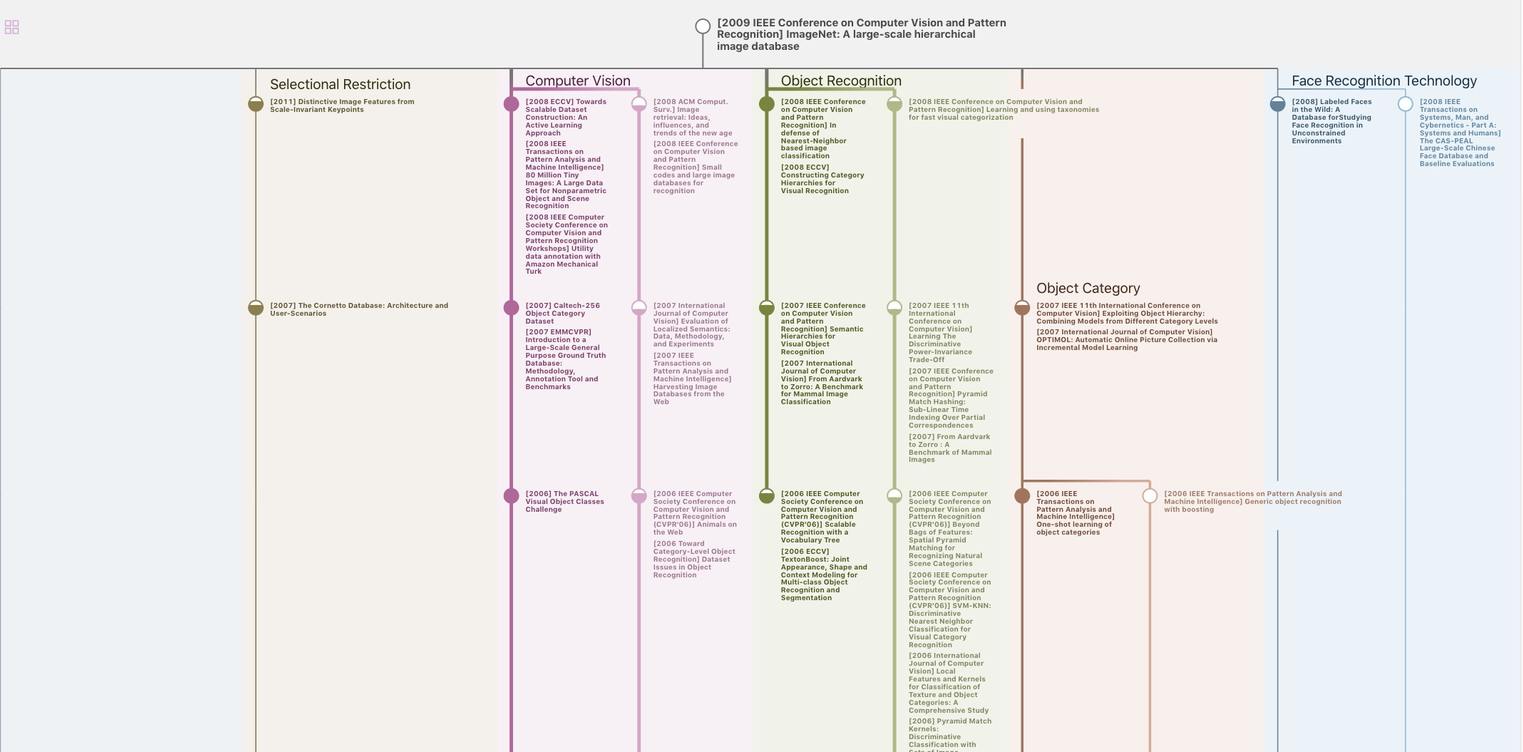
生成溯源树,研究论文发展脉络
Chat Paper
正在生成论文摘要