Evolutionary Computational Intelligence-Based Multi-Objective Sensor Management for Multi-Target Tracking
REMOTE SENSING(2022)
摘要
In multi-sensor systems (MSSs), sensor selection is a critical technique for obtaining high-quality sensing data. However, when the number of sensors to be selected is unknown in advance, sensor selection is essentially non-deterministic polynomial-hard (NP-hard), and finding the optimal solution is computationally unacceptable. To alleviate these issues, we propose a novel sensor selection approach based on evolutionary computational intelligence for tracking multiple targets in the MSSs. The sensor selection problem is formulated in a partially observed Markov decision process framework by modeling multi-target states as labeled multi-Bernoulli random finite sets. Two conflicting task-driven objectives are considered: minimization of the uncertainty in posterior cardinality estimates and minimization of the number of selected sensors. By modeling sensor selection as a multi-objective optimization problem, we develop a binary constrained evolutionary multi-objective algorithm based on non-dominating sorting and dynamically select a subset of sensors at each time step. Numerical studies are used to evaluate the performance of the proposed approach, where the MSS tracks multiple moving targets with nonlinear/linear dynamic models and nonlinear measurements. The results show that our method not only significantly reduces the number of selected sensors but also provides superior tracking accuracy compared to generic sensor selection methods.
更多查看译文
关键词
computational intelligence,intelligent sensing technique,multi-sensor systems,multi-target tracking,random finite set,sensor selection
AI 理解论文
溯源树
样例
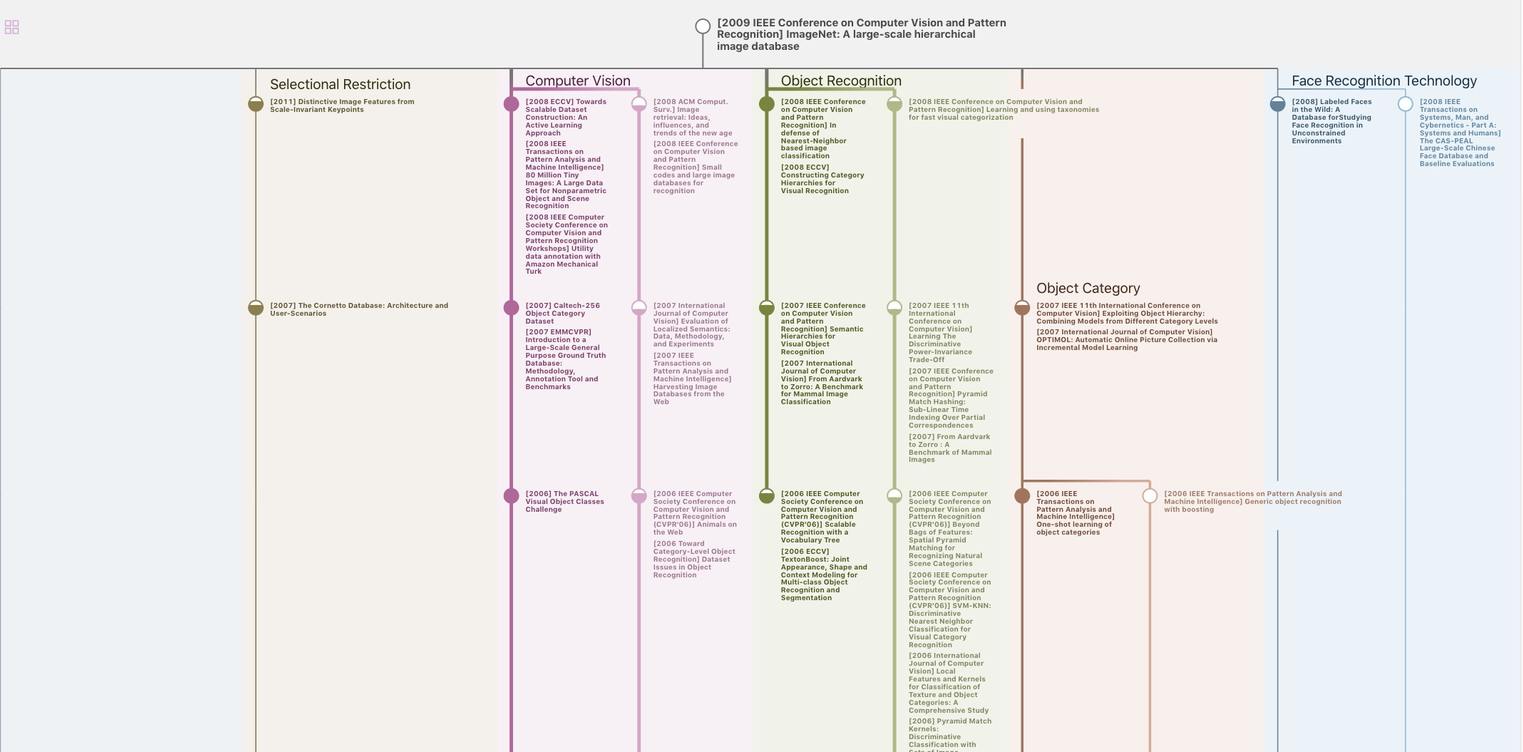
生成溯源树,研究论文发展脉络
Chat Paper
正在生成论文摘要