Evaluation of echosounder data preparation strategies for modern machine learning models
Fisheries Research(2022)
摘要
Fish stock assessment and management requires accurate estimates of fish abundance, which are typically derived from echosounder observations using acoustic target classification (ATC). Skilled operators are regularly assisted in classifying acoustic targets by software and there has been an increasing interest toward using machine learning to create improved tools. Recent studies have applied deep learning approaches to acoustic data, however, algorithm data-preparation strategies (influencing model output) are presently poorly understood and standardization is needed to enable collaborative research and management. For example, a common pre-processing technique is to resample backscatter data coming from echosounder measurements from the original resolution to a coarser resolution in the horizontal (time) and vertical (range) directions. Using data values derived from the volume backscattering coefficient obtained during the Norwegian sandeel survey, we investigate which resampling resolutions are suitable for ATC using a convolutional neural network trained to classify single values of backscatter data. This process is known as pixel-level semantic segmentation. Our results indicate that it is possible to downsample the data if important information related to acoustic characteristics is not smoothed out. We also show that the classification performance is improved when providing the network with contextual information relating to range. These findings will provide input to fisheries acoustic data standards and contribute to the on-going development of automated ATC methods.
更多查看译文
关键词
Acoustic target classification,Multi-frequency echograms,Deep learning,Semantic segmentation,U-Net,Resampling,Sandeel
AI 理解论文
溯源树
样例
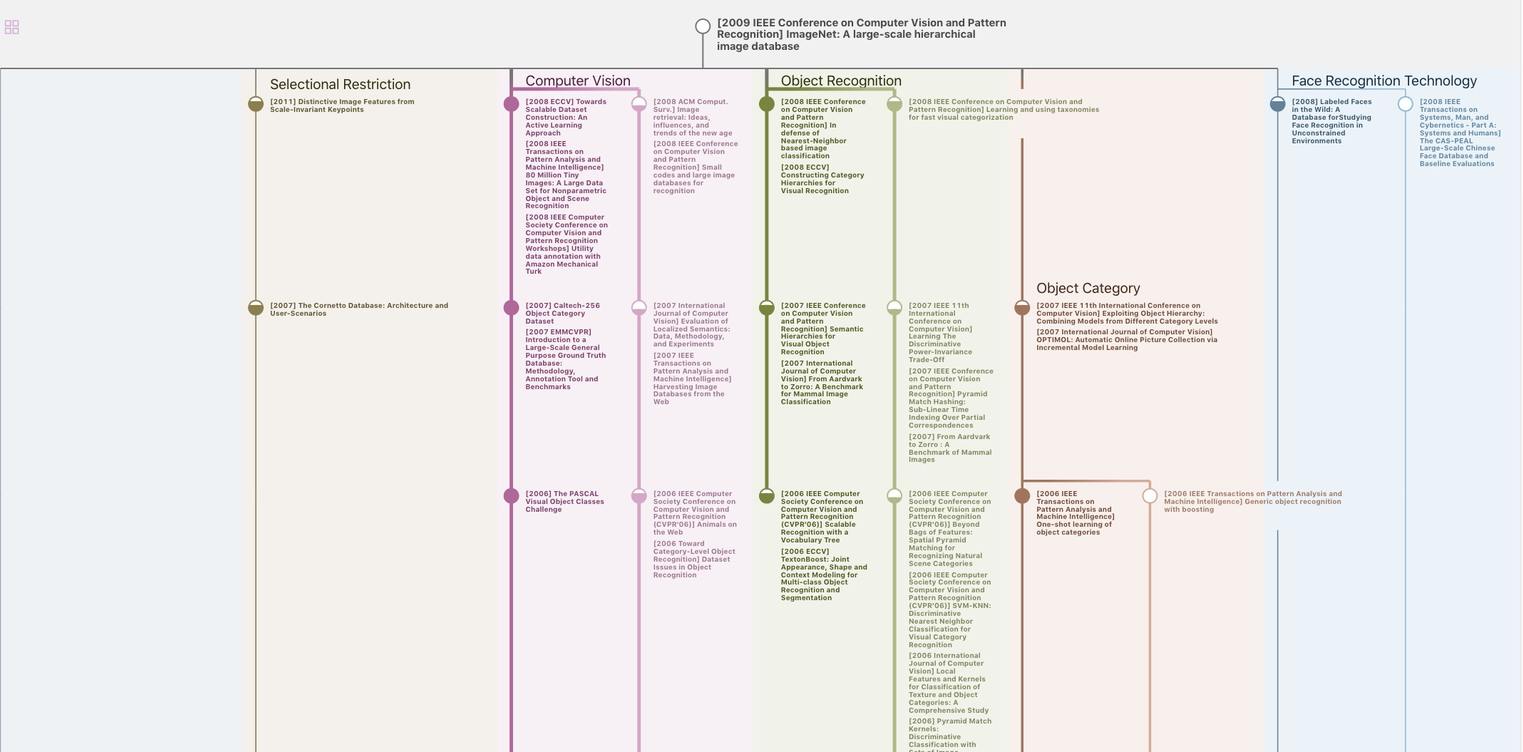
生成溯源树,研究论文发展脉络
Chat Paper
正在生成论文摘要