Ensemble Capsule Network with an Attention Mechanism for the Fault Diagnosis of Bearings from Imbalanced Data Samples
SENSORS(2022)
摘要
In order to solve the problem of imbalanced and noisy data samples for the fault diagnosis of rolling bearings, a novel ensemble capsule network (Capsnet) with a convolutional block attention module (CBAM) that is based on a weighted majority voting method is proposed in this study. Firstly, the complete ensemble empirical mode decomposition with adaptive noise (CEEMDAN) method was used to decompose the raw vibration signal into different IMF signals, which are noise reduction signals. Secondly, the IMF signals were input into the Capsnet with CBAM in order to diagnose the fault category preliminarily. Finally, the weighted majority voting method was utilized so as to fuse all of the preliminary diagnosis results in order to obtain the final diagnostic decision. In order to verify the effectiveness of the proposed ensemble of Capsnet with CBAM, this method was applied to the fault diagnosis of rolling bearings with imbalanced and different SNR data samples. The diagnostic results show that the proposed diagnostic method can achieve higher levels of accuracy than other methods, such as single CNN, single Capsnet, ensemble CNN and an ensemble capsule network without CBAM and that it has stronger immunity to noise than an ensemble capsule network without CBAM.
更多查看译文
关键词
ensemble capsule network, imbalanced dataset, fault diagnosis
AI 理解论文
溯源树
样例
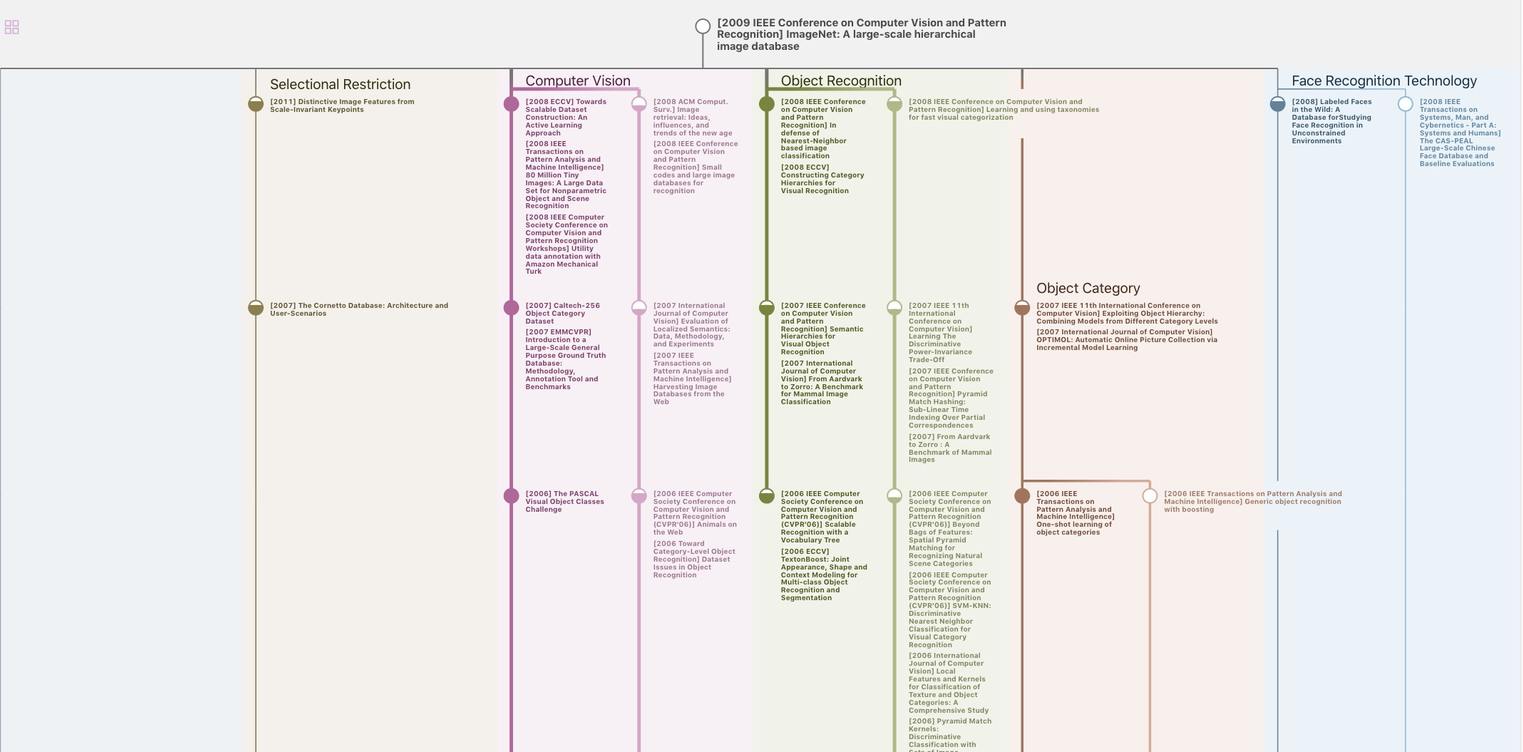
生成溯源树,研究论文发展脉络
Chat Paper
正在生成论文摘要