A Pipeline for the Implementation and Visualization of Explainable Machine Learning for Medical Imaging Using Radiomics Features
SENSORS(2022)
摘要
Machine learning (ML) models have been shown to predict the presence of clinical factors from medical imaging with remarkable accuracy. However, these complex models can be difficult to interpret and are often criticized as "black boxes". Prediction models that provide no insight into how their predictions are obtained are difficult to trust for making important clinical decisions, such as medical diagnoses or treatment. Explainable machine learning (XML) methods, such as Shapley values, have made it possible to explain the behavior of ML algorithms and to identify which predictors contribute most to a prediction. Incorporating XML methods into medical software tools has the potential to increase trust in ML-powered predictions and aid physicians in making medical decisions. Specifically, in the field of medical imaging analysis the most used methods for explaining deep learning-based model predictions are saliency maps that highlight important areas of an image. However, they do not provide a straightforward interpretation of which qualities of an image area are important. Here, we describe a novel pipeline for XML imaging that uses radiomics data and Shapley values as tools to explain outcome predictions from complex prediction models built with medical imaging with well-defined predictors. We present a visualization of XML imaging results in a clinician-focused dashboard that can be generalized to various settings. We demonstrate the use of this workflow for developing and explaining a prediction model using MRI data from glioma patients to predict a genetic mutation.
更多查看译文
关键词
explainable machine learning, medical imaging, information visualization, radiomics
AI 理解论文
溯源树
样例
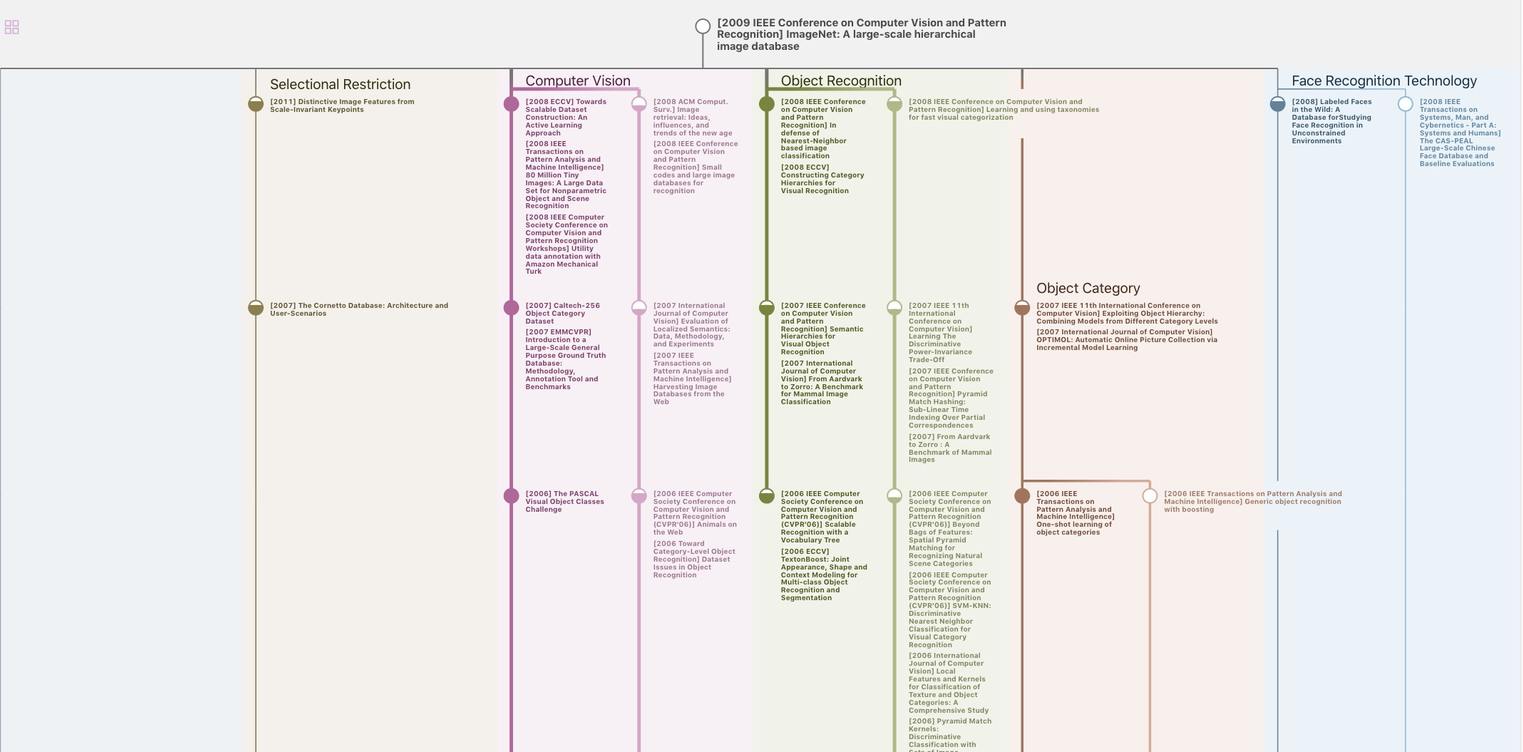
生成溯源树,研究论文发展脉络
Chat Paper
正在生成论文摘要