Convergence analysis on the deterministic mini-batch learning algorithm for noise resilient radial basis function networks
International Journal of Machine Learning and Cybernetics(2022)
摘要
This paper gives a formal convergence analysis on the mini-batch training algorithm for noise resilient radial basis function (RBF) networks. Unlike the conventional analysis which assumes that the mini-batch process is operated in a stochastic manner, we consider that the mini-batch training process is operated in a deterministic manner. The deterministic process divides the training samples into a number of fixed mini-batches, and the mini-batches are presented in a fixed order. This paper first states the noise resilient objective function for weight noise and weight fault. We then derive the mini-batch training algorithm for this noise resilient objective function. Our main contribution is the convergence analysis on the mini-batch training algorithm. We show that under the deterministic setting, the mini-batch training algorithm converges. The converged weight vector is asymptotically close to the optimal batch mode solution. Also, we derive the sufficient conditions (the learning rate range) for convergence. Our theoretical results can be applied to not only the noise resilient objective function but also a large class of objective functions.
更多查看译文
关键词
Convergence analysis, Noise resilience, Weight noise, Weight fault, Fault tolerance, Mini-batch training, Online training, Radial basis function networks
AI 理解论文
溯源树
样例
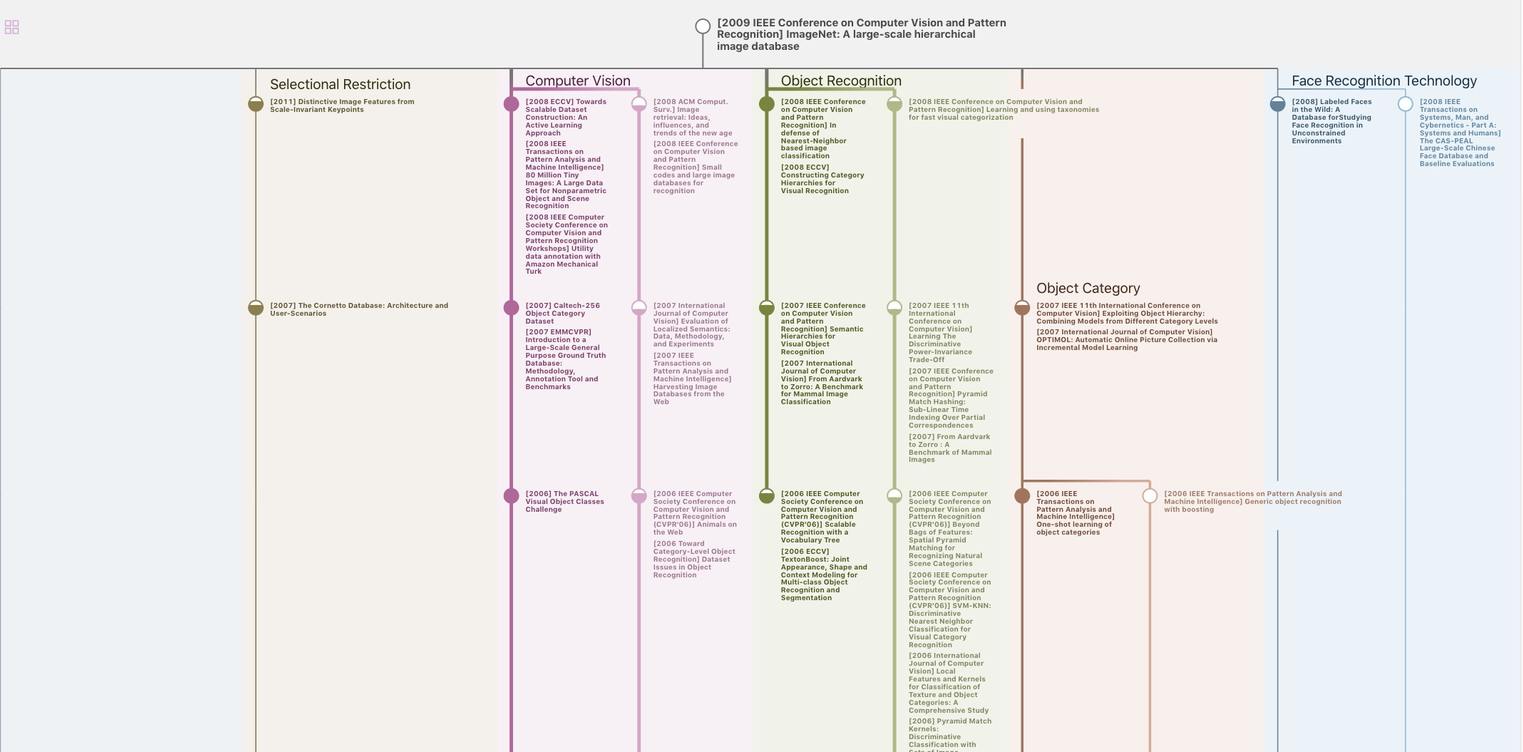
生成溯源树,研究论文发展脉络
Chat Paper
正在生成论文摘要