Blockchain Assisted Intrusion Detection System Using Differential Flower Pollination Model
Computers, Materials & Continua(2022)
摘要
Cyberattacks are developing gradually sophisticated, requiring effective intrusion detection systems (IDSs) for monitoring computer resources and creating reports on anomalous or suspicious actions. With the popularity of Internet of Things (IoT) technology, the security of IoT networks is developing a vital problem. Because of the huge number and varied kinds of IoT devices, it can be challenging task for protecting the IoT framework utilizing a typical IDS. The typical IDSs have their restrictions once executed to IoT networks because of resource constraints and complexity. Therefore, this paper presents a new Blockchain Assisted Intrusion Detection System using Differential Flower Pollination with Deep Learning (BAIDS-DFPDL) model in IoT Environment. The presented BAIDS-DFPDL model mainly focuses on the identification and classification of intrusions in the IoT environment. To accomplish this, the presented BAIDS-DFPDL model follows blockchain (BC) technology for effective and secure data transmission among the agents. Besides, the presented BAIDS-DFPDLmodel designs Differential Flower Pollination based feature selection (DFPFS) technique to elect features. Finally, sailfish optimization (SFO) with Restricted Boltzmann Machine (RBM) model is applied for effectual recognition of intrusions. The simulation results on benchmark dataset exhibit the enhanced performance of the BAIDS-DFPDL model over other models on the recognition of intrusions.
更多查看译文
关键词
Internet of things, feature selection, intrusion detection, blockchain, security, deep learning
AI 理解论文
溯源树
样例
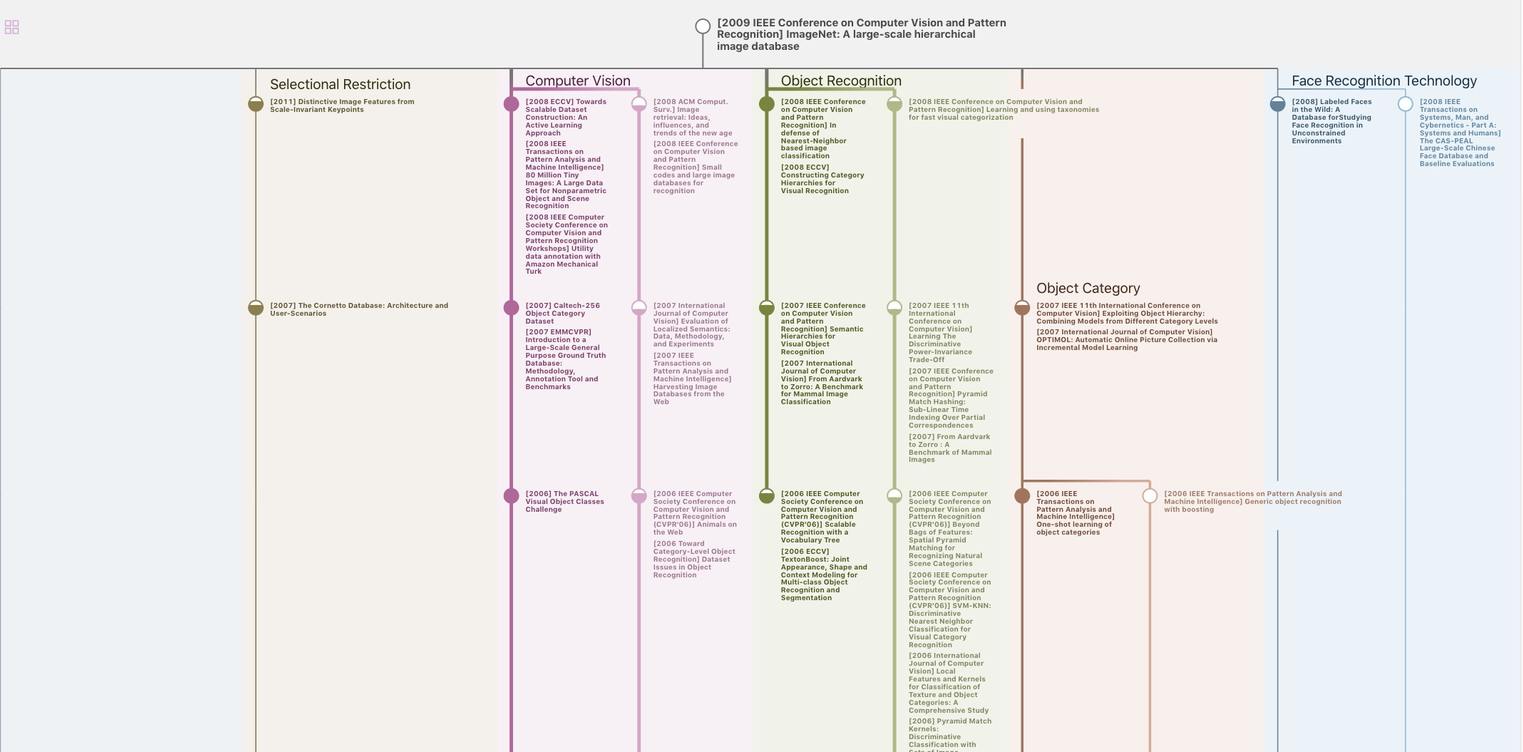
生成溯源树,研究论文发展脉络
Chat Paper
正在生成论文摘要