Prediction of biomarker-disease associations based on graph attention network and text representation
BRIEFINGS IN BIOINFORMATICS(2022)
摘要
Motivation: The associations between biomarkers and human diseases play a key role in understanding complex pathology and developing targeted therapies. Wet lab experiments for biomarker discovery are costly, laborious and time-consuming. Computational prediction methods can be used to greatly expedite the identification of candidate biomarkers. Results: Here, we present a novel computational model named GTGenie for predicting the biomarker-disease associations based on graph and text features. In GTGenie, a graph attention network is utilized to characterize diverse similarities of biomarkers and diseases from heterogeneous information resources. Meanwhile, a pretrained BERT-based model is applied to learn the text-based representation of biomarker-disease relation from biomedical literature. The captured graph and text features are then integrated in a bimodal fusion network to model the hybrid entity representation. Finally, inductive matrix completion is adopted to infer the missing entries for reconstructing relation matrix, with which the unknown biomarker-disease associations are predicted. Experimental results on HMDD, HMDAD and LncRNADisease data sets showed that GTGenie can obtain competitive prediction performance with other state-of-the-art methods. Availability: The source code of GTGenie and the test data are available at: https://github.com/Wolverinerine/GTGenie.
更多查看译文
关键词
miRNA-disease associations,microbe-disease associations,lncRNA-disease associations,graph attention network,text-based relation representation,bimodal fusion network
AI 理解论文
溯源树
样例
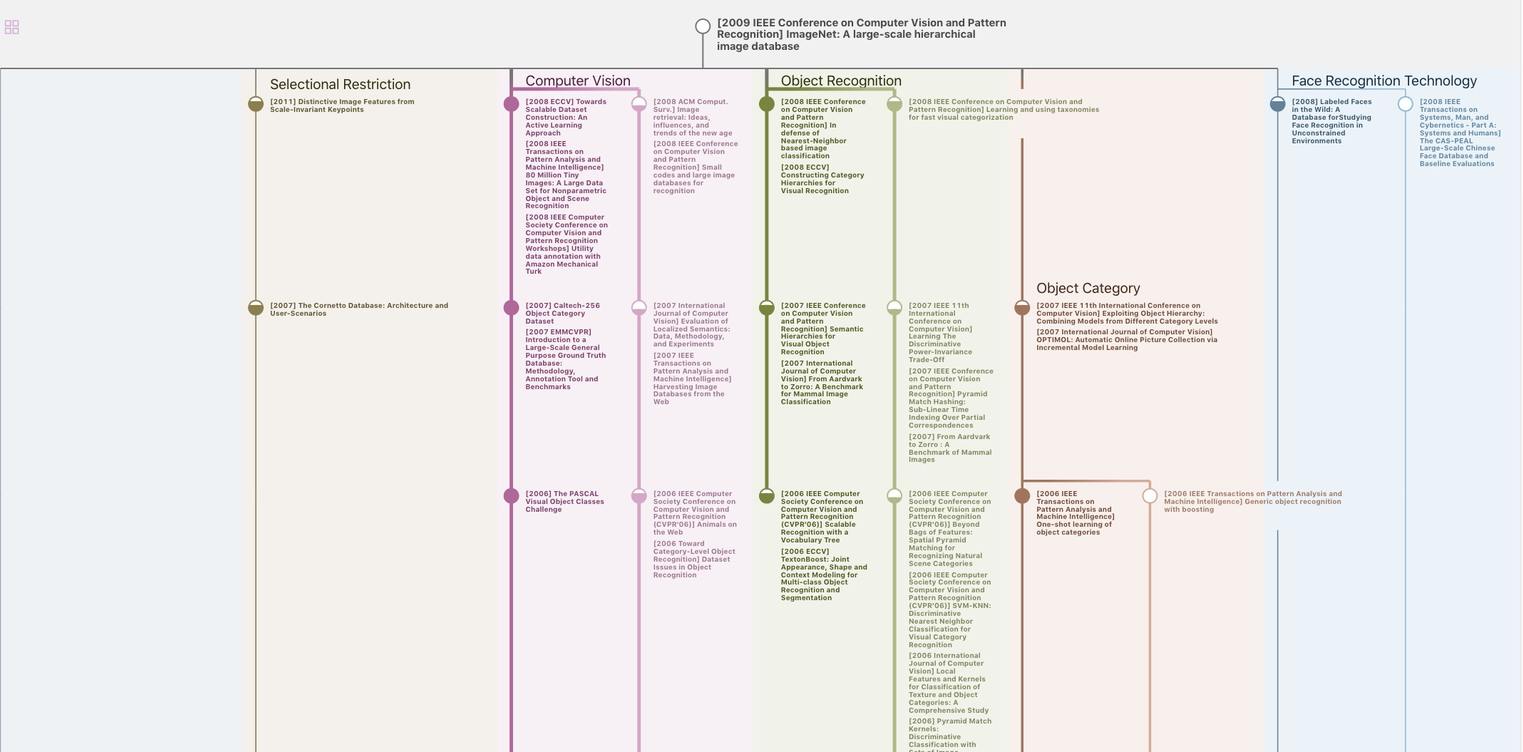
生成溯源树,研究论文发展脉络
Chat Paper
正在生成论文摘要