Knowing Where and What: Unified Word Block Pretraining for Document Understanding
arxiv(2022)
摘要
Due to the complex layouts of documents, it is challenging to extract information for documents. Most previous studies develop multimodal pre-trained models in a self-supervised way. In this paper, we focus on the embedding learning of word blocks containing text and layout information, and propose UTel, a language model with Unified TExt and Layout pre-training. Specifically, we propose two pre-training tasks: Surrounding Word Prediction (SWP) for the layout learning, and Contrastive learning of Word Embeddings (CWE) for identifying different word blocks. Moreover, we replace the commonly used 1D position embedding with a 1D clipped relative position embedding. In this way, the joint training of Masked Layout-Language Modeling (MLLM) and two newly proposed tasks enables the interaction between semantic and spatial features in a unified way. Additionally, the proposed UTel can process arbitrary-length sequences by removing the 1D position embedding, while maintaining competitive performance. Extensive experimental results show UTel learns better joint representations and achieves superior performance than previous methods on various downstream tasks, though requiring no image modality. Code is available at \url{https://github.com/taosong2019/UTel}.
更多查看译文
关键词
unified word block pretraining,document
AI 理解论文
溯源树
样例
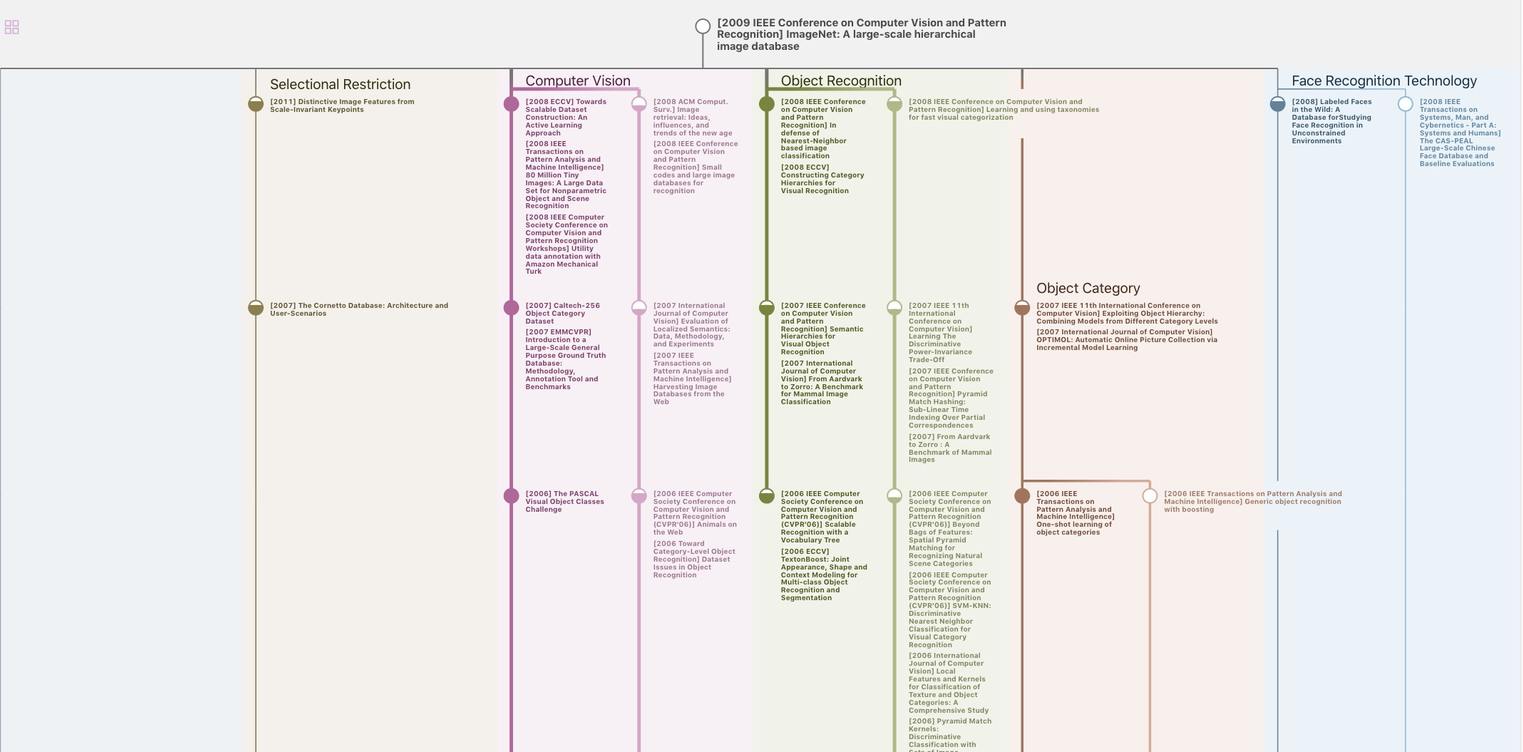
生成溯源树,研究论文发展脉络
Chat Paper
正在生成论文摘要