EventHD: Robust and efficient hyperdimensional learning with neuromorphic sensor
FRONTIERS IN NEUROSCIENCE(2022)
摘要
Brain-inspired computing models have shown great potential to outperform today's deep learning solutions in terms of robustness and energy efficiency. Particularly, Hyper-Dimensional Computing (HDC) has shown promising results in enabling efficient and robust cognitive learning. In this study, we exploit HDC as an alternative computational model that mimics important brain functionalities toward high-efficiency and noise-tolerant neuromorphic computing. We present EventHD, an end-to-end learning framework based on HDC for robust, efficient learning from neuromorphic sensors. We first introduce a spatial and temporal encoding scheme to map event-based neuromorphic data into high-dimensional space. Then, we leverage HDC mathematics to support learning and cognitive tasks over encoded data, such as information association and memorization. EventHD also provides a notion of confidence for each prediction, thus enabling self-learning from unlabeled data. We evaluate EventHD efficiency over data collected from Dynamic Vision Sensor (DVS) sensors. Our results indicate that EventHD can provide online learning and cognitive support while operating over raw DVS data without using the costly preprocessing step. In terms of efficiency, EventHD provides 14.2x faster and 19.8x higher energy efficiency than state-of-the-art learning algorithms while improving the computational robustness by 5.9x.
更多查看译文
关键词
hyperdimensional computing, neuromorphic sensor, brain-inspired computing, Dynamic Vision Sensor, machine learning
AI 理解论文
溯源树
样例
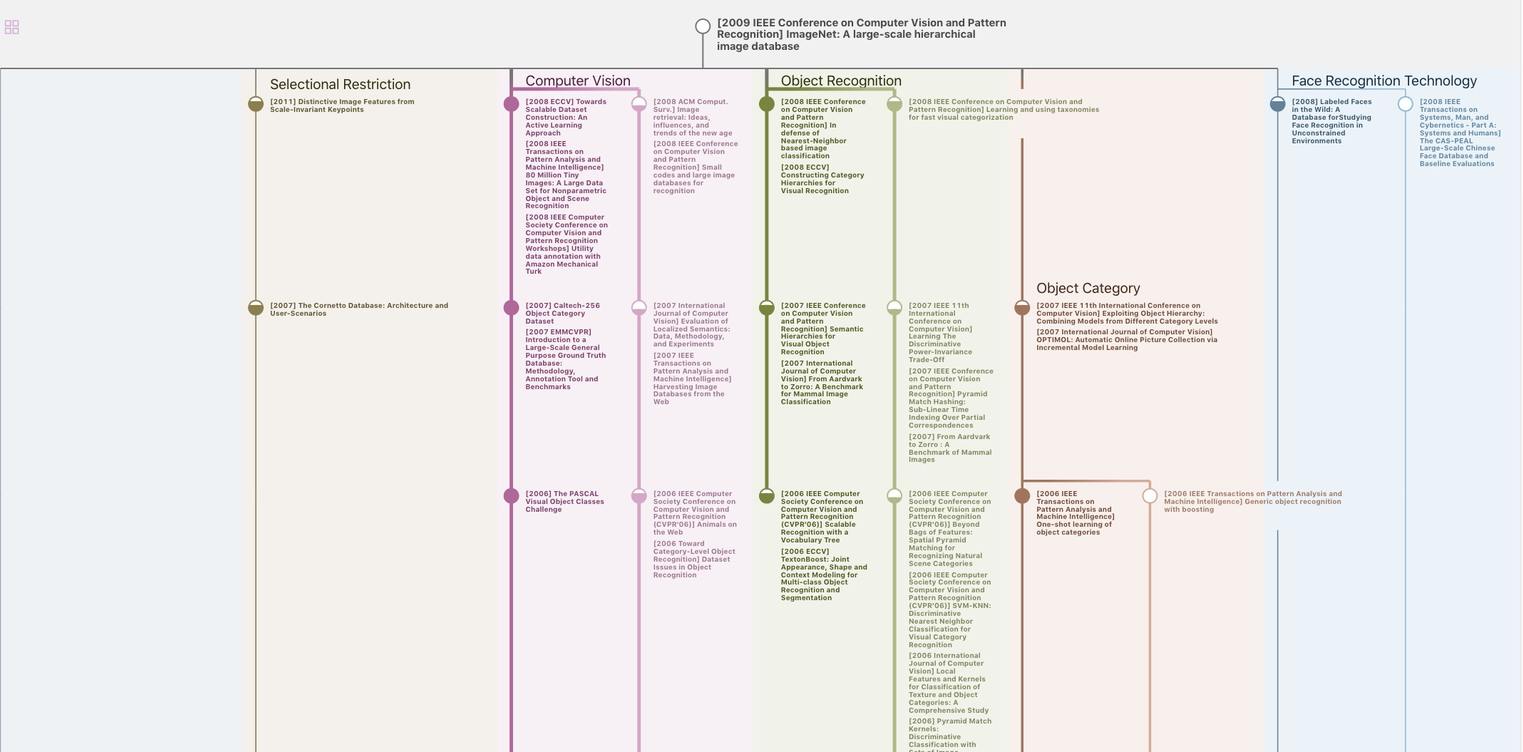
生成溯源树,研究论文发展脉络
Chat Paper
正在生成论文摘要