Estimating the Individual Treatment Effect on Survival Time Based on Prior Knowledge and Counterfactual Prediction
ENTROPY(2022)
摘要
The estimation of the Individual Treatment Effect (ITE) on survival time is an important research topic in clinics-based causal inference. Various representation learning methods have been proposed to deal with its three key problems, i.e., reducing selection bias, handling censored survival data, and avoiding balancing non-confounders. However, none of them consider all three problems in a single method. In this study, by combining the Counterfactual Survival Analysis (CSA) model and Dragonnet from the literature, we first propose a CSA-Dragonnet to deal with the three problems simultaneously. Moreover, we found that conclusions from traditional Randomized Controlled Trials (RCTs) or Retrospective Cohort Studies (RCSs) can offer valuable bound information to the counterfactual learning of ITE, which has never been used by existing ITE estimation methods. Hence, we further propose a CSA-Dragonnet with Embedded Prior Knowledge (CDNEPK) by formulating a unified expression of the prior knowledge given by RCTs or RCSs, inserting counterfactual prediction nets into CSA-Dragonnet and defining loss items based on the bounds for the ITE extracted from prior knowledge. Semi-synthetic data experiments showed that CDNEPK has superior performance. Real-world experiments indicated that CDNEPK can offer meaningful treatment advice.
更多查看译文
关键词
individual treatment effect, survival data, counterfactual prediction, prior knowledge
AI 理解论文
溯源树
样例
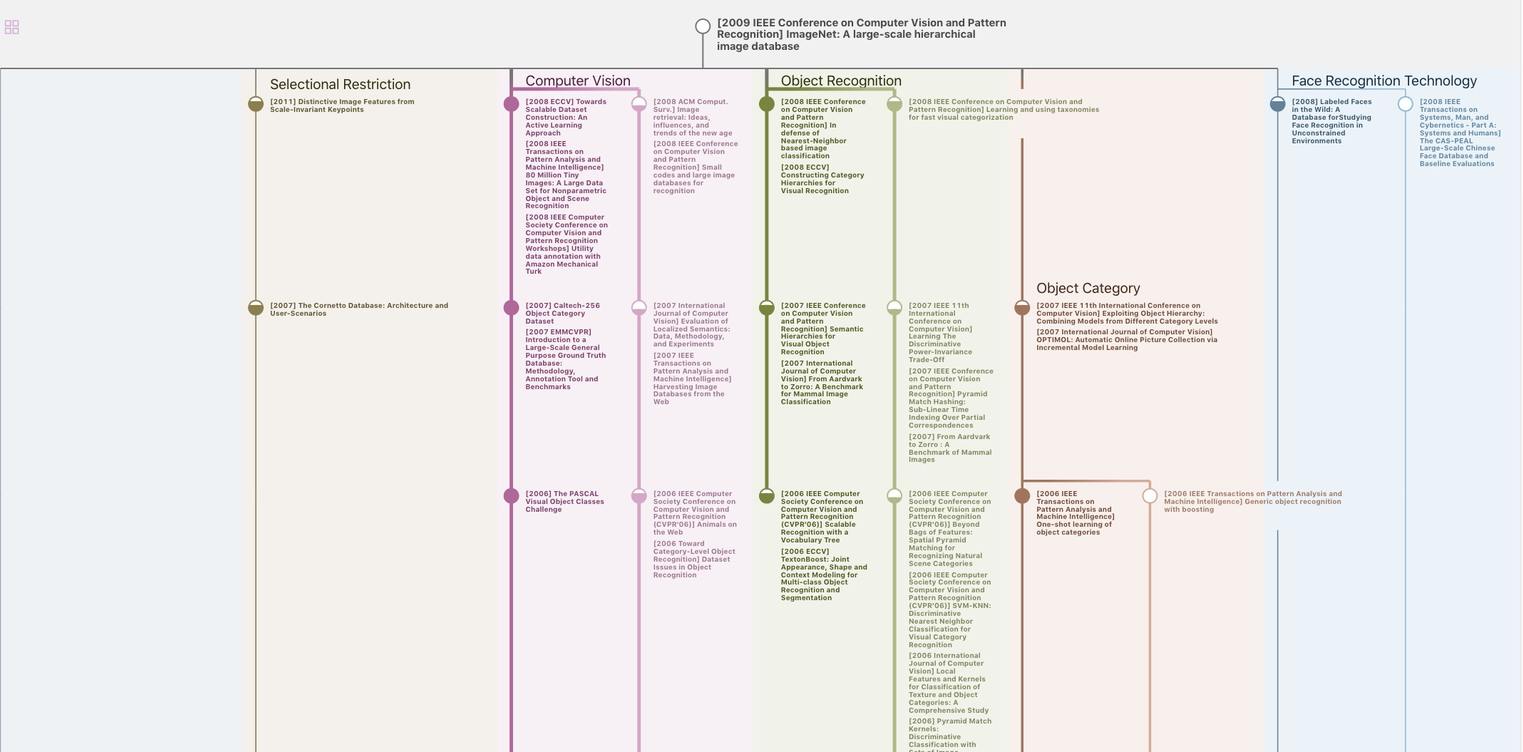
生成溯源树,研究论文发展脉络
Chat Paper
正在生成论文摘要