Learning Hyper Label Model for Programmatic Weak Supervision
arxiv(2023)
摘要
To reduce the human annotation efforts, the programmatic weak supervision (PWS) paradigm abstracts weak supervision sources as labeling functions (LFs) and involves a label model to aggregate the output of multiple LFs to produce training labels. Most existing label models require a parameter learning step for each dataset. In this work, we present a hyper label model that (once learned) infers the ground-truth labels for each dataset in a single forward pass without dataset-specific parameter learning. The hyper label model approximates an optimal analytical (yet computationally intractable) solution of the ground-truth labels. We train the model on synthetic data generated in the way that ensures the model approximates the analytical optimal solution, and build the model upon Graph Neural Network (GNN) to ensure the model prediction being invariant (or equivariant) to the permutation of LFs (or data points). On 14 real-world datasets, our hyper label model outperforms the best existing methods in both accuracy (by 1.4 points on average) and efficiency (by six times on average). Our code is available at https://github.com/wurenzhi/hyper_label_model
更多查看译文
关键词
Programmatic Weak Supervision,Data Programming,Label Model
AI 理解论文
溯源树
样例
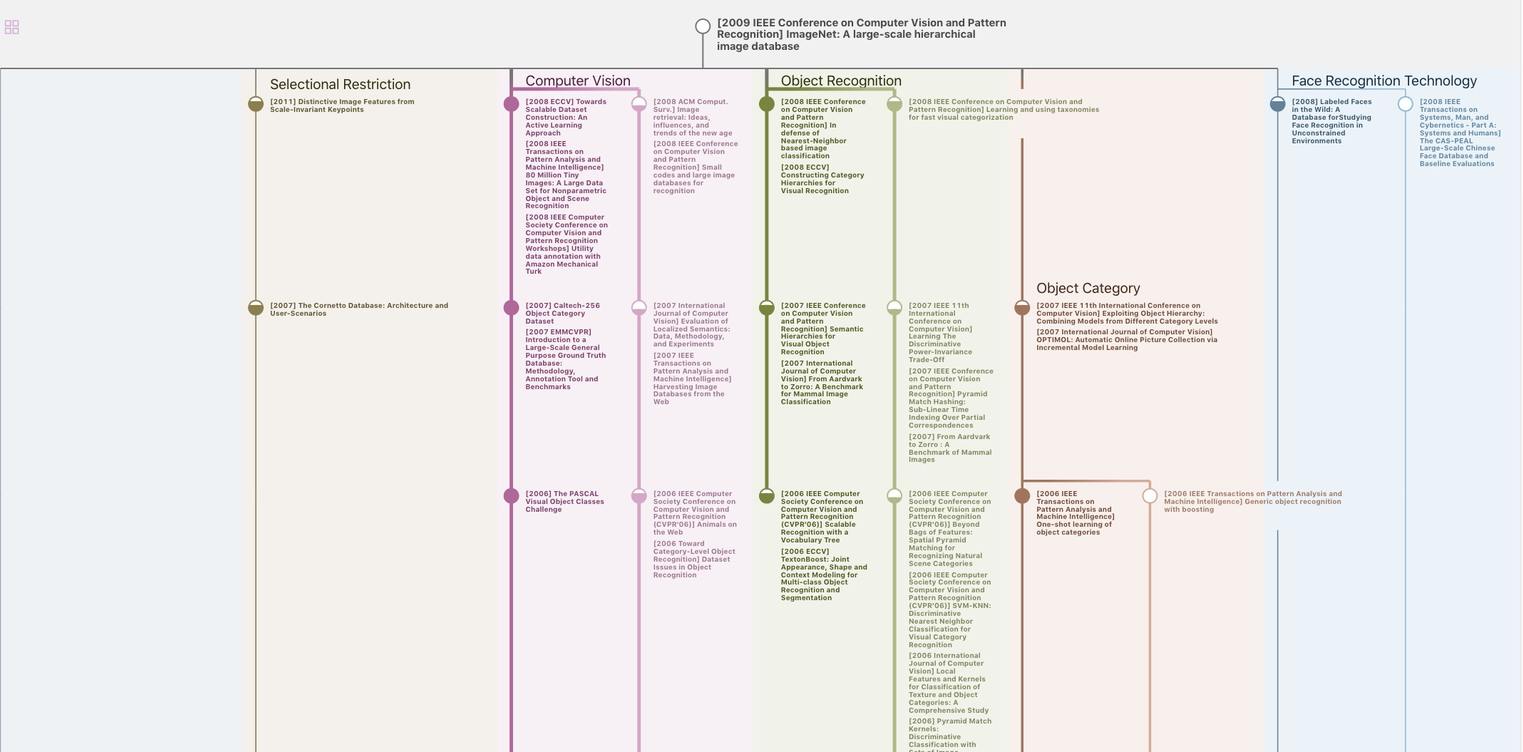
生成溯源树,研究论文发展脉络
Chat Paper
正在生成论文摘要