Bayesian dynamic network modelling: an application to metabolic associations in cardiovascular diseases
JOURNAL OF APPLIED STATISTICS(2024)
摘要
We propose a novel approach to the estimation of multiple Graphical Models to analyse temporal patterns of association among a set of metabolites over different groups of patients. Our motivating application is the Southall And Brent REvisited (SABRE) study, a tri-ethnic cohort study conducted in the UK. We are interested in identifying potential ethnic differences in metabolite levels and associations as well as their evolution over time, with the aim of gaining a better understanding of different risk of cardio-metabolic disorders across ethnicities. Within a Bayesian framework, we employ a nodewise regression approach to infer the structure of the graphs, borrowing information across time as well as across ethnicities. The response variables of interest are metabolite levels measured at two time points and for two ethnic groups, Europeans and South-Asians. We use nodewise regression to estimate the high-dimensional precision matrices of the metabolites, imposing sparsity on the regression coefficients through the dynamic horseshoe prior, thus favouring sparser graphs. We provide the code to fit the proposed model using the software Stan, which performs posterior inference using Hamiltonian Monte Carlo sampling, as well as a detailed description of a block Gibbs sampling scheme.
更多查看译文
关键词
Dynamic shrinkage priors,Gibbs sampling,graphical models,metabolomics,nodewise regression
AI 理解论文
溯源树
样例
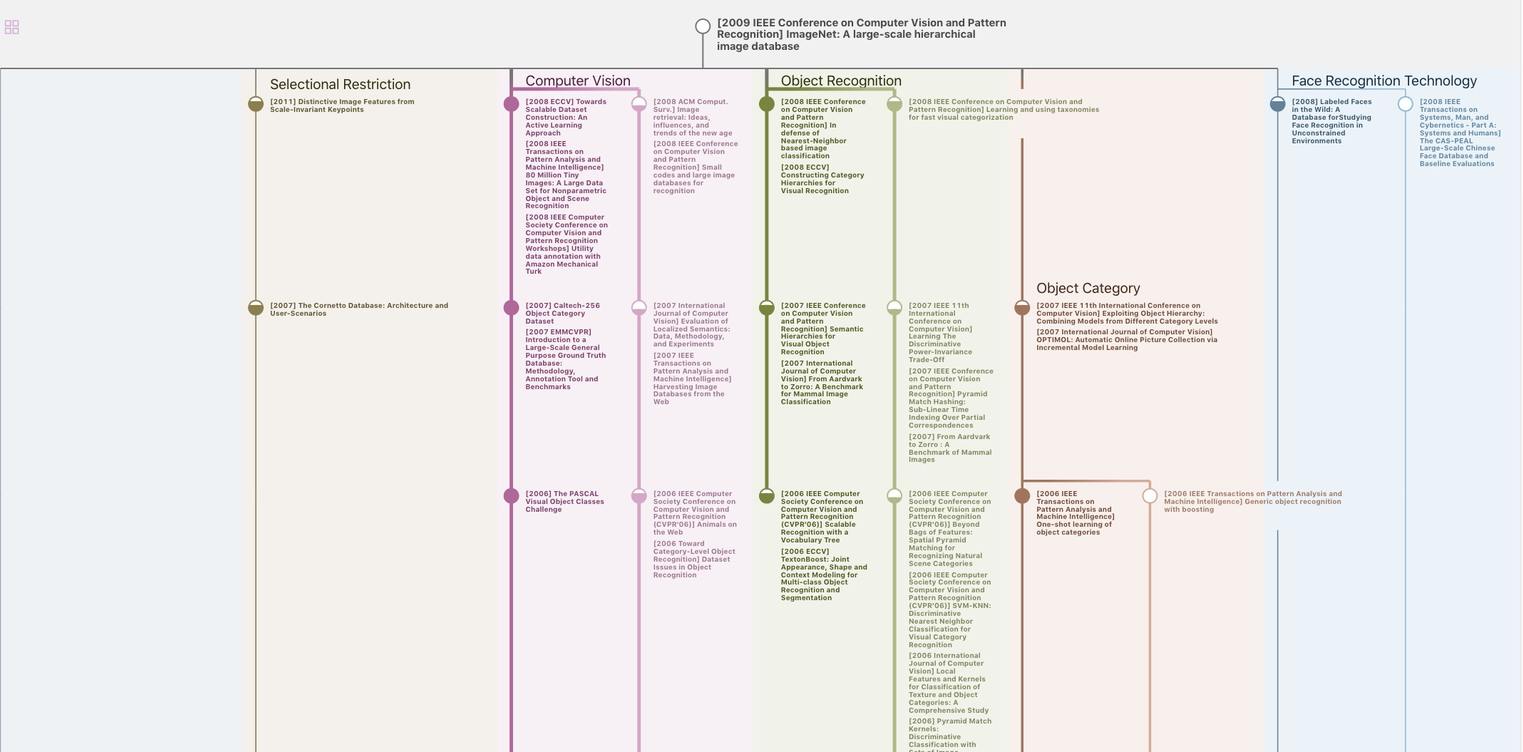
生成溯源树,研究论文发展脉络
Chat Paper
正在生成论文摘要