Multiobjective Differential Evolution With Speciation for Constrained Multimodal Multiobjective Optimization
IEEE Transactions on Evolutionary Computation(2023)
摘要
This article proposes a novel differential evolution algorithm for solving constrained multimodal multiobjective optimization problems (CMMOPs), which may have multiple feasible Pareto-optimal solutions with identical objective vectors. In CMMOPs, due to the coexistence of multimodality and constraints, it is difficult for current algorithms to perform well in both objective and decision spaces. The proposed algorithm uses the speciation mechanism to induce niches preserving more feasible Pareto-optimal solutions and adopts an improved environment selection criterion to enhance diversity. The algorithm can not only obtain feasible solutions but also retain more well-distributed feasible Pareto-optimal solutions. Moreover, a set of constrained multimodal multiobjective test functions is developed. All these test functions have multimodal characteristics and contain multiple constraints. Meanwhile, this article proposes a new indicator, which comprehensively considers the feasibility, convergence, and diversity of a solution set. The effectiveness of the proposed method is verified by comparing with the state-of-the-art algorithms on both test functions and real-world location-selection problem.
更多查看译文
关键词
multiobjective differential evolution,constrained multimodal multiobjective optimization,speciation
AI 理解论文
溯源树
样例
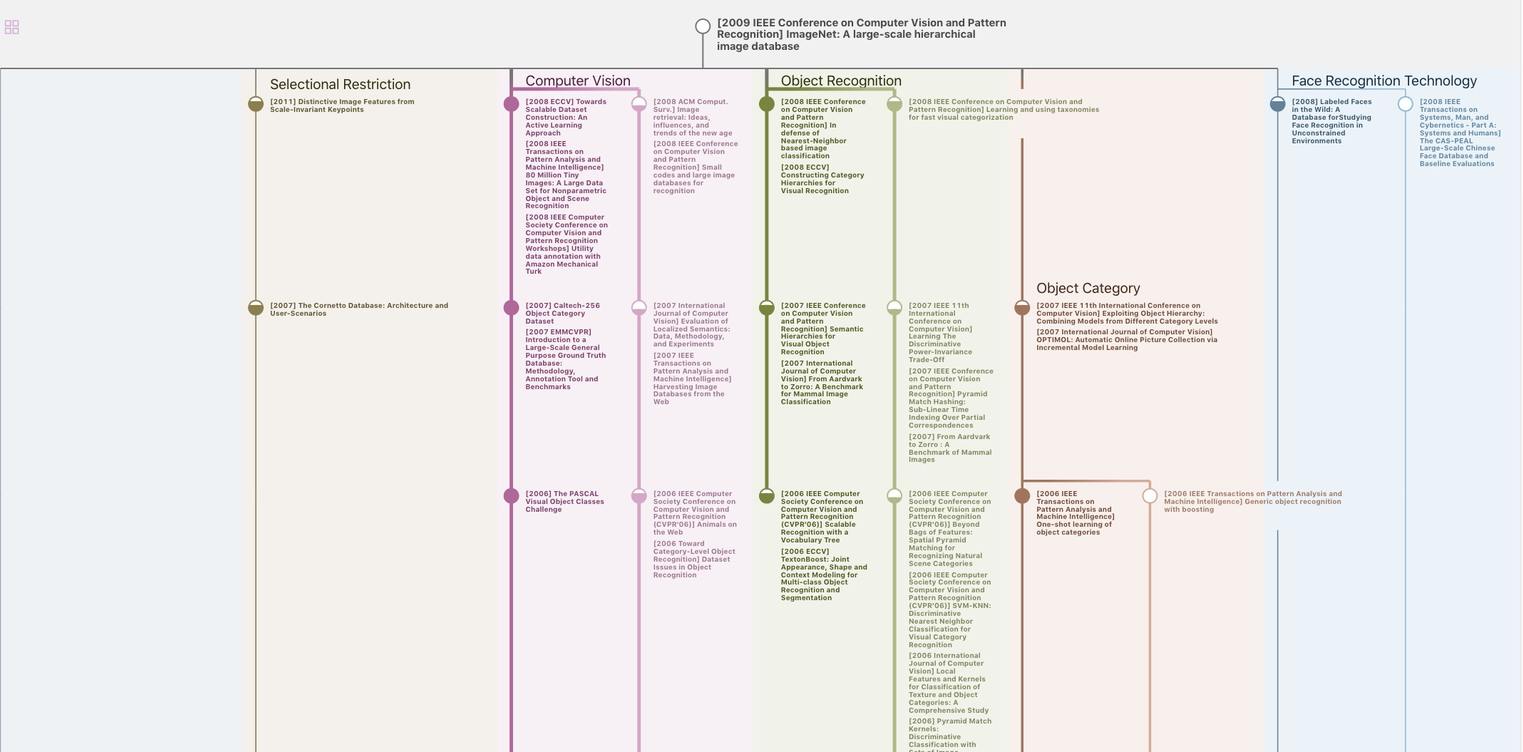
生成溯源树,研究论文发展脉络
Chat Paper
正在生成论文摘要