An Integrative Heterogeneous Graph Neural Network-Based Method for Multi-Labeled Drug Repurposing
FRONTIERS IN PHARMACOLOGY(2022)
摘要
Drug repurposing is the process of discovering new indications (i.e., diseases or conditions) for already approved drugs. Many computational methods have been proposed for predicting new associations between drugs and diseases. In this article, we proposed a new method, called DR-HGNN, an integrative heterogeneous graph neural network-based method for multi-labeled drug repurposing, to discover new indications for existing drugs. For this purpose, we first used the DTINet dataset to construct a heterogeneous drug-protein-disease (DPD) network, which is a graph composed of four types of nodes (drugs, proteins, diseases, and drug side effects) and eight types of edges. Second, we labeled each drug-protein edge, dp(i,j) = (d(i), p(j)), of the DPD network with a set of diseases, {delta(i,j,1), horizontal ellipsis , delta(i,j,k)} associated with both d(i) and p(j) and then devised multi-label ranking approaches which incorporate neural network architecture that operates on the heterogeneous graph-structured data and which leverages both the interaction patterns and the features of drug and protein nodes. We used a derivative of the GraphSAGE algorithm, HinSAGE, on the heterogeneous DPD network to learn low-dimensional vector representation of features of drugs and proteins. Finally, we used the drug-protein network to learn the embeddings of the drug-protein edges and then predict the disease labels that act as bridges between drugs and proteins. The proposed method shows better results than existing methods applied to the DTINet dataset, with an AUC of 0.964.
更多查看译文
关键词
computational drug repurposing, graph embedding, graphsage, data integration, link prediction, graph neural network
AI 理解论文
溯源树
样例
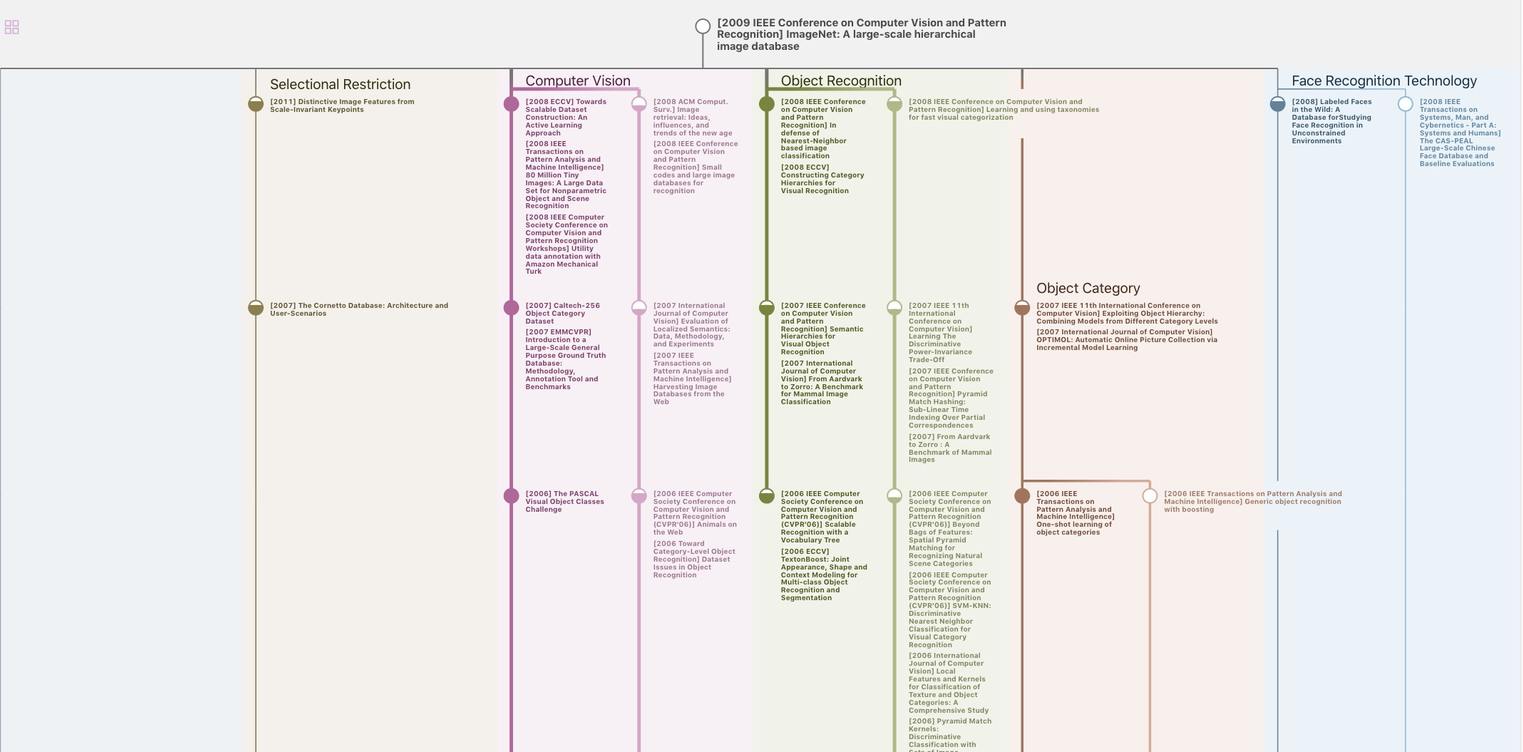
生成溯源树,研究论文发展脉络
Chat Paper
正在生成论文摘要