Engagement-Based Player Typologies Describe Game-Based Learning Outcomes.
ARTIFICIAL INTELLIGENCE IN EDUCATION: POSTERS AND LATE BREAKING RESULTS, WORKSHOPS AND TUTORIALS, INDUSTRY AND INNOVATION TRACKS, PRACTITIONERS AND DOCTORAL CONSORTIUM, PT II(2022)
摘要
Engagement is a strong predictor of learning in educational contexts, but the definition of engagement can vary from study to study, with small differences in definition leading to substantial differences in findings. In addition, students frequently employ strategies in online learning systems that the system designers may not have expected, which can challenge the assumptions made in these definitions. Students playing educational games employ a particularly wide variety of strategies and behaviors, which can make measuring overall engagement with the game challenging. In this study we examine student engagement by describing players' profiles of behaviors and interactions with a physics-based simulation game, Physics Playground. To identify possible sub-groups of players we use Latent Profile Analysis (LPA), a type of person-centered mixture model that assigns individuals to a set of mutually exclusive classes based on patterns of variance in a set of response data. We found support for two classes of players - high engagement players and low engagement players - and we show that students' membership in these classes is predictive of their performance on a posttest assessment. We end by discussing the limitations of this method, as well as the potential for identification and analysis of these types of player profiles to be used in adaptive game mechanics and personalization of learning contexts.
更多查看译文
关键词
Game-Based Learning,Mixture modeling,Engagement profile,Player typology
AI 理解论文
溯源树
样例
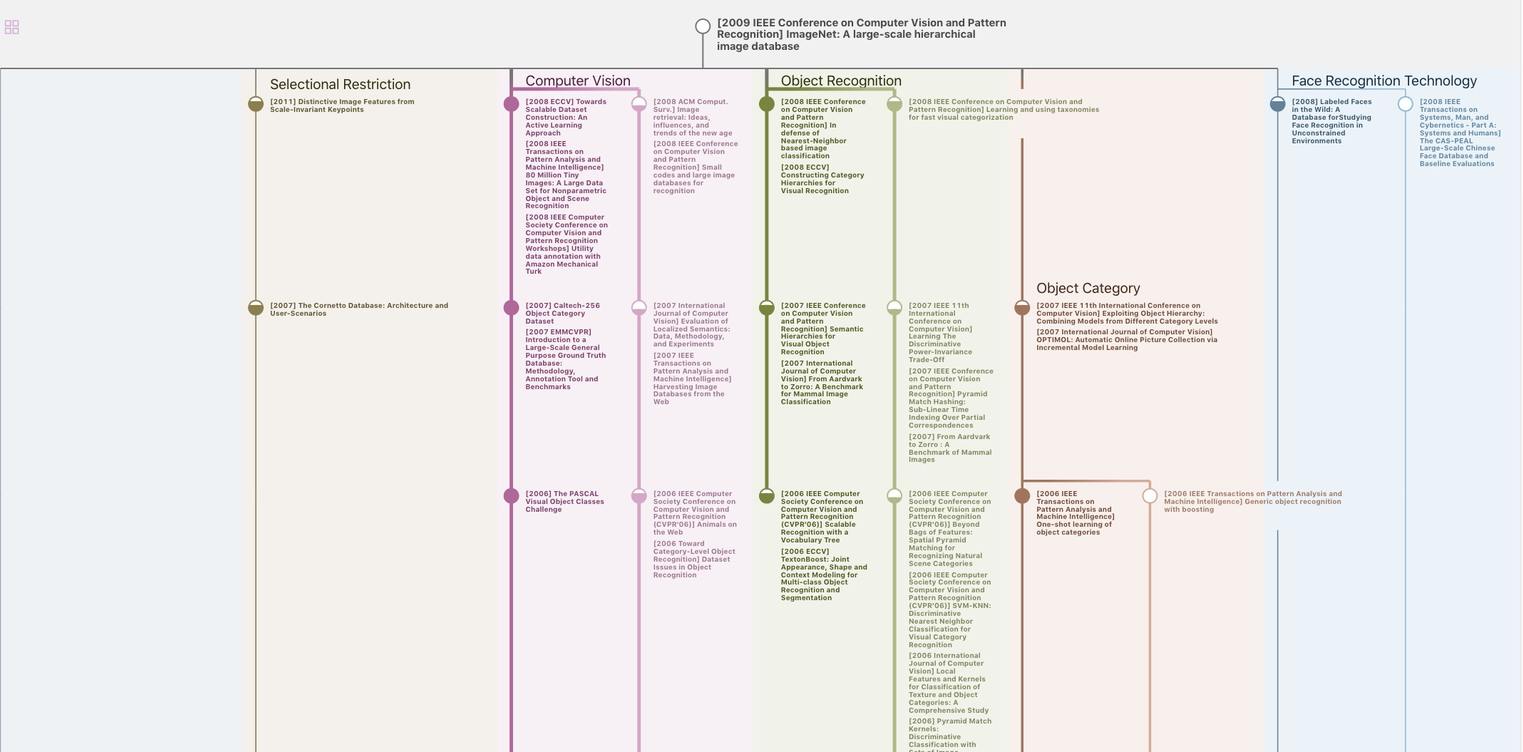
生成溯源树,研究论文发展脉络
Chat Paper
正在生成论文摘要