Contrastive Knowledge-Augmented Meta-Learning for Few-Shot Classification
WACV(2023)
摘要
Model agnostic meta-learning algorithms aim to infer priors from several observed tasks that can then be used to adapt to a new task with few examples. Given the inherent diversity of tasks arising in existing benchmarks, recent methods have resorted to task-specific adaptation of the prior. Our goal is to improve generalization of meta learners when the task distribution contains challenging distribution shifts and semantic disparities. To this end, we introduce CAML (Contrastive Knowledge-Augmented Meta Learning), a knowledge-enhanced few-shot learning approach that evolves a knowledge graph to encode historical experience, and employs a contrastive distillation strategy to leverage the encoded knowledge for task-aware modulation of the base learner. In addition to the standard fewshot task adaptation, we also consider the more challenging multi-domain task adaptation and few-shot dataset generalization settings in our evaluation with standard benchmarks. Our empirical study shows that CAML (i) enables simple task encoding schemes; (ii) eliminates the need for knowledge extraction at inference time; and most importantly, (iii) effectively aggregates historical experience thus leading to improved performance in both multi-domain adaptation and dataset generalization.
更多查看译文
关键词
Algorithms: Machine learning architectures,formulations,and algorithms (including transfer),Image recognition and understanding (object detection,categorization,segmentation,scene modeling,visual reasoning)
AI 理解论文
溯源树
样例
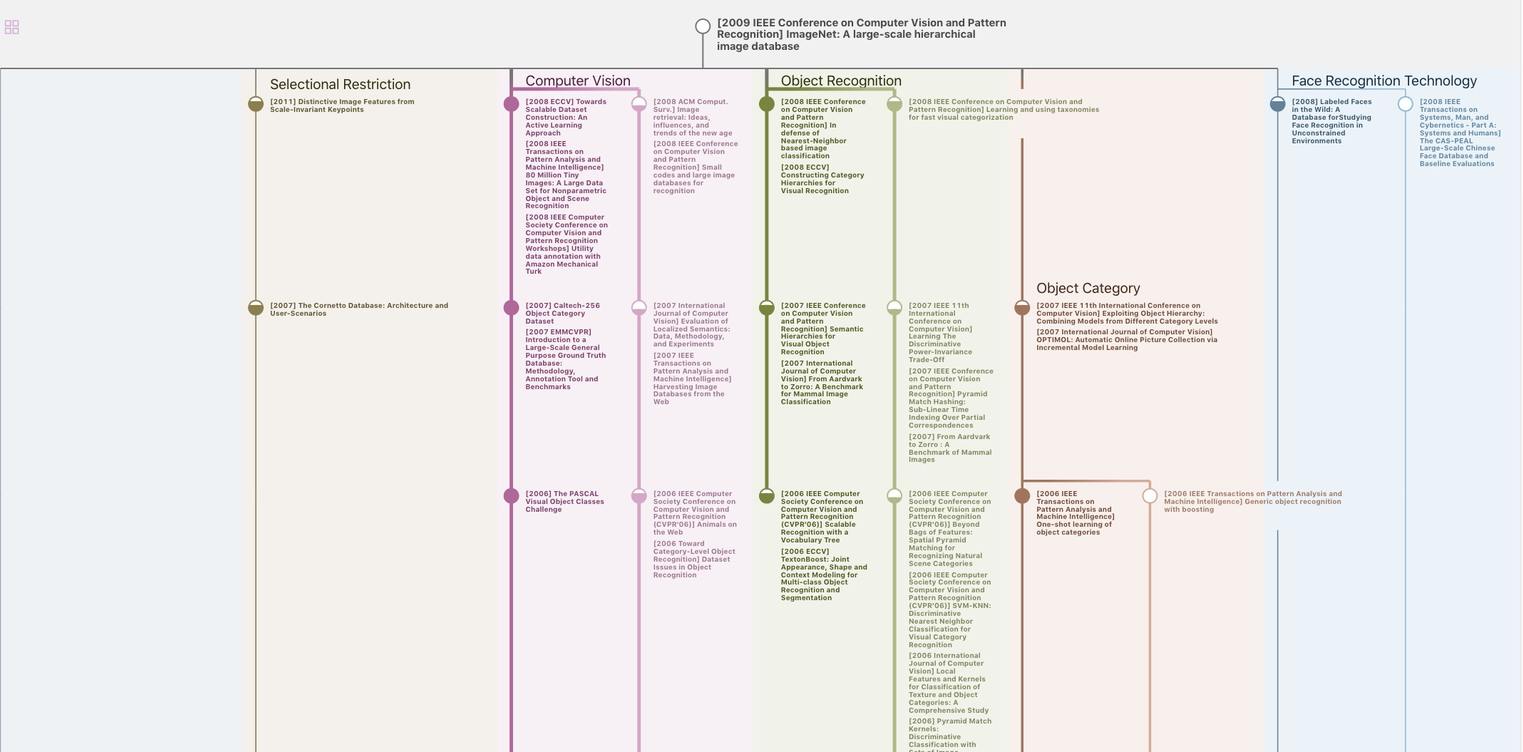
生成溯源树,研究论文发展脉络
Chat Paper
正在生成论文摘要