Learning a Dual-Mode Speech Recognition Model VIA Self-Pruning
2022 IEEE Spoken Language Technology Workshop (SLT)(2023)
摘要
There is growing interest in unifying the streaming and full-context automatic speech recognition (ASR) networks into a single end-to-end ASR model to simplify the model training and deployment for both use cases. While in real-world ASR applications, the streaming ASR models typically operate under more storage and computational constraints - e.g., on embedded devices - than any server-side full-context models. Motivated by the recent progress in Omni-sparsity supernet training, where multiple subnetworks are jointly optimized in one single model, this work aims to jointly learn a compact sparse on-device streaming ASR model, and a large dense server non-streaming model, in a single supernet. Next, we present that, performing supernet training on both wav2vec 2.0 self-supervised learning and supervised ASR fine-tuning can not only substantially improve the large non-streaming model as shown in prior works, and also be able to improve the compact sparse streaming model.
更多查看译文
关键词
Neural network pruning,sparsity optimization,supernet,recurrent neural network transducer
AI 理解论文
溯源树
样例
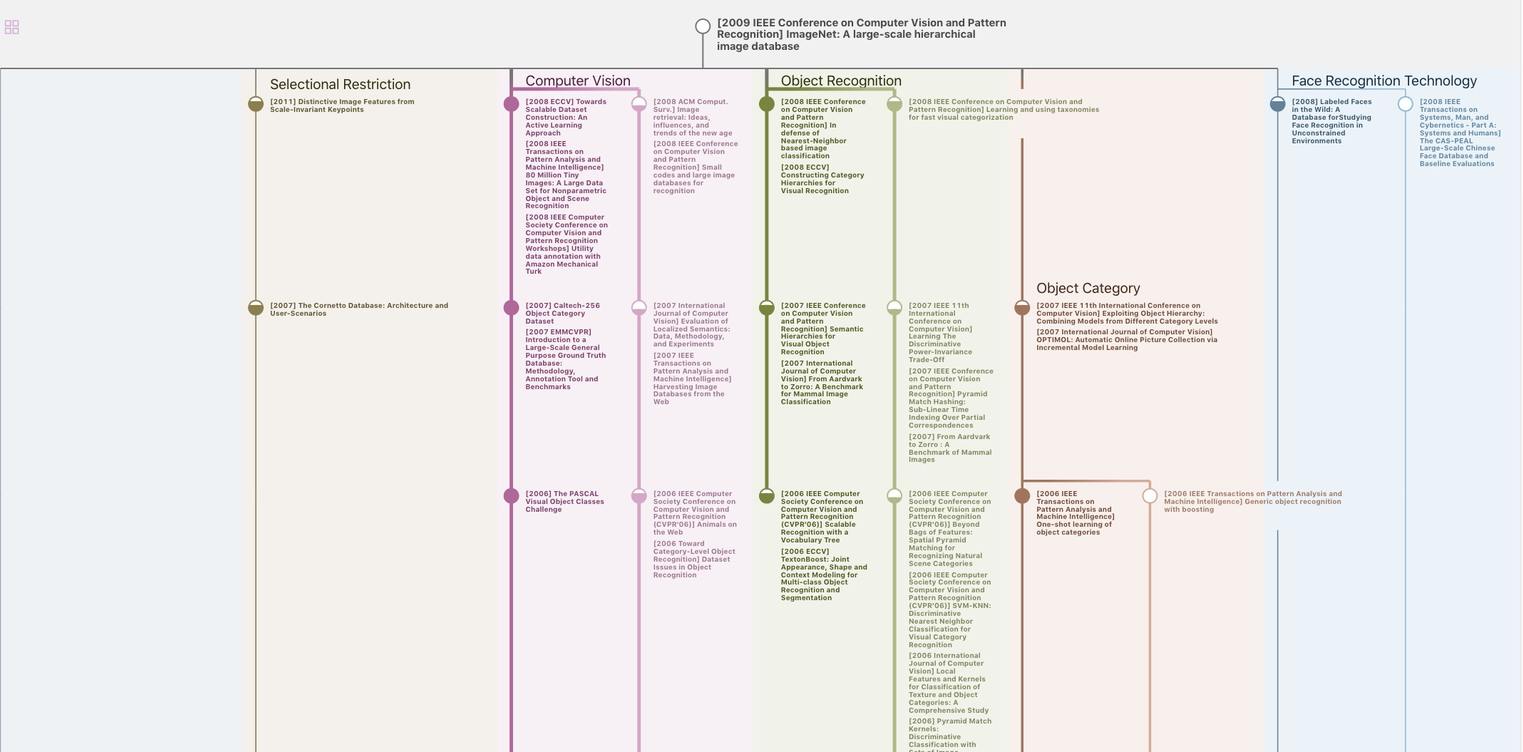
生成溯源树,研究论文发展脉络
Chat Paper
正在生成论文摘要