nLMVS-Net: Deep Non-Lambertian Multi-View Stereo
WACV(2023)
摘要
We introduce a novel multi-view stereo (MVS) method that can simultaneously recover not just per-pixel depth but also surface normals, together with the reflectance of textureless, complex non-Lambertian surfaces captured under known but natural illumination. Our key idea is to formulate MVS as an end-to-end learnable network, which we refer to as nLMVS-Net, that seamlessly integrates radiometric cues to leverage surface normals as view-independent surface features for learned cost volume construction and filtering. It first estimates surface normals as pixel-wise probability densities for each view with a novel shape-from-shading network. These per-pixel surface normal densities and the input multi-view images are then input to a novel cost volume filtering network that learns to recover per-pixel depth and surface normal. The reflectance is also explicitly estimated by alternating with geometry reconstruction. Extensive quantitative evaluations on newly established synthetic and real-world datasets show that nLMVS-Net can robustly and accurately recover the shape and reflectance of complex objects in natural settings.
更多查看译文
关键词
stereo,nlmvs-net,non-lambertian,multi-view
AI 理解论文
溯源树
样例
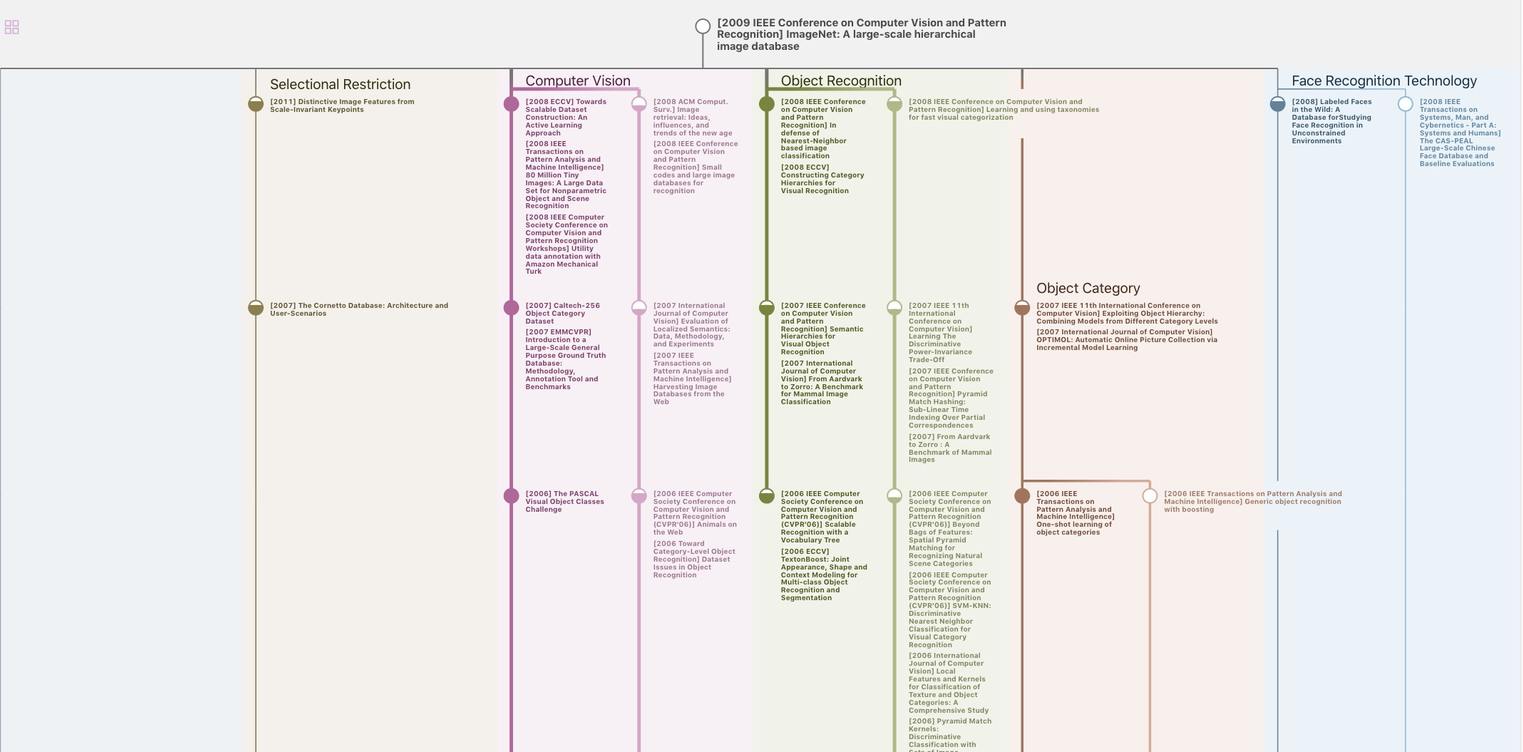
生成溯源树,研究论文发展脉络
Chat Paper
正在生成论文摘要