Optimal data generation for machine learned interatomic potentials
arxiv(2022)
摘要
Machine learning interatomic potentials (MLIPs) are routinely used atomic simulations, but generating databases of atomic configurations used in fitting these models is a laborious process, requiring significant computational and human effort. A computationally efficient method is presented to generate databases of atomic configurations that contain optimal information on the small-displacement regime of the potential energy surface of bulk crystalline matter. Utilising non-diagonal supercell (NDSC), an automatic process is suggested for ab initio data generation. MLIPs were fitted for Al, W, Mg and Si, which very closely reproduce the ab initio phonon and elastic properties. The protocol can be easily adapted to other materials and can be inserted in the workflow of any flavour of MLIP generation.
更多查看译文
关键词
database generation,interatomic potential fitting,phonon dispersion
AI 理解论文
溯源树
样例
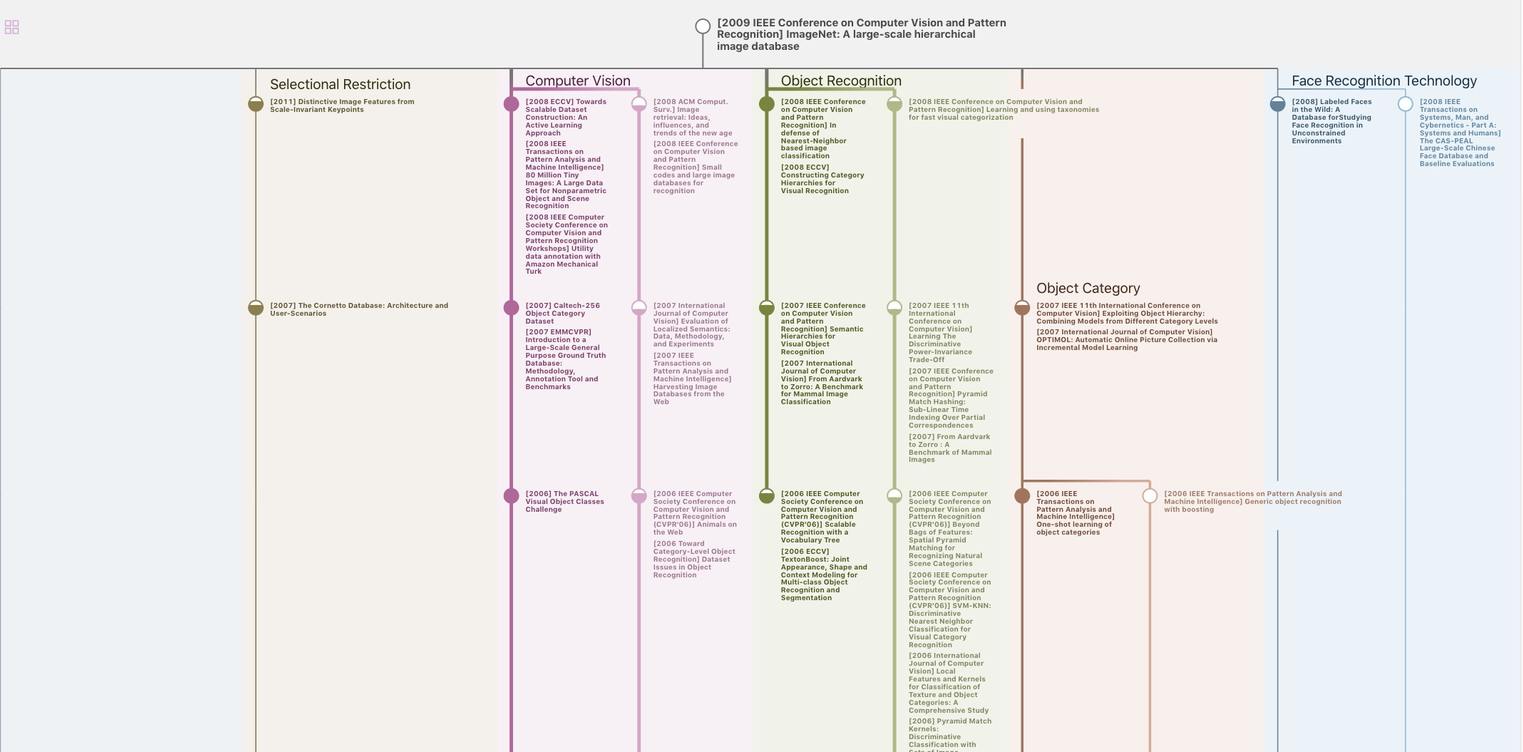
生成溯源树,研究论文发展脉络
Chat Paper
正在生成论文摘要