FastATDC: Fast Anomalous Trajectory Detection and Classification.
IEEE Conference on Automation Science and Engineering (CASE)(2022)
摘要
Automated detection of anomalous trajectories is an important problem with considerable applications in intelligent transportation systems. Many existing studies have focused on distinguishing anomalous trajectories from normal trajectories, ignoring the large differences between anomalous trajectories. A recent study has made great progress in identifying abnormal trajectory patterns and proposed a two-stage algorithm for anomalous trajectory detection and classification (ATDC). This algorithm has excellent performance but suffers from a few limitations, such as high time complexity and poor interpretation. Here, we present a careful theoretical and empirical analysis of the ATDC algorithm, showing that the calculation of anomaly scores in both stages can be simplified, and that the second stage of the algorithm is much more important than the first stage. Hence, we develop a FastATDC algorithm that introduces a random sampling strategy in both stages. Experimental results show that FastATDC is 10 to 20 times faster than ATDC on real datasets. Moreover, FastATDC outperforms the baseline algorithms and is comparable to the ATDC algorithm.
更多查看译文
关键词
fast anomalous trajectory detection,automated detection,intelligent transportation systems,distinguishing anomalous trajectories,normal trajectories,abnormal trajectory patterns,two-stage algorithm,ATDC algorithm,FastATDC algorithm,baseline algorithms
AI 理解论文
溯源树
样例
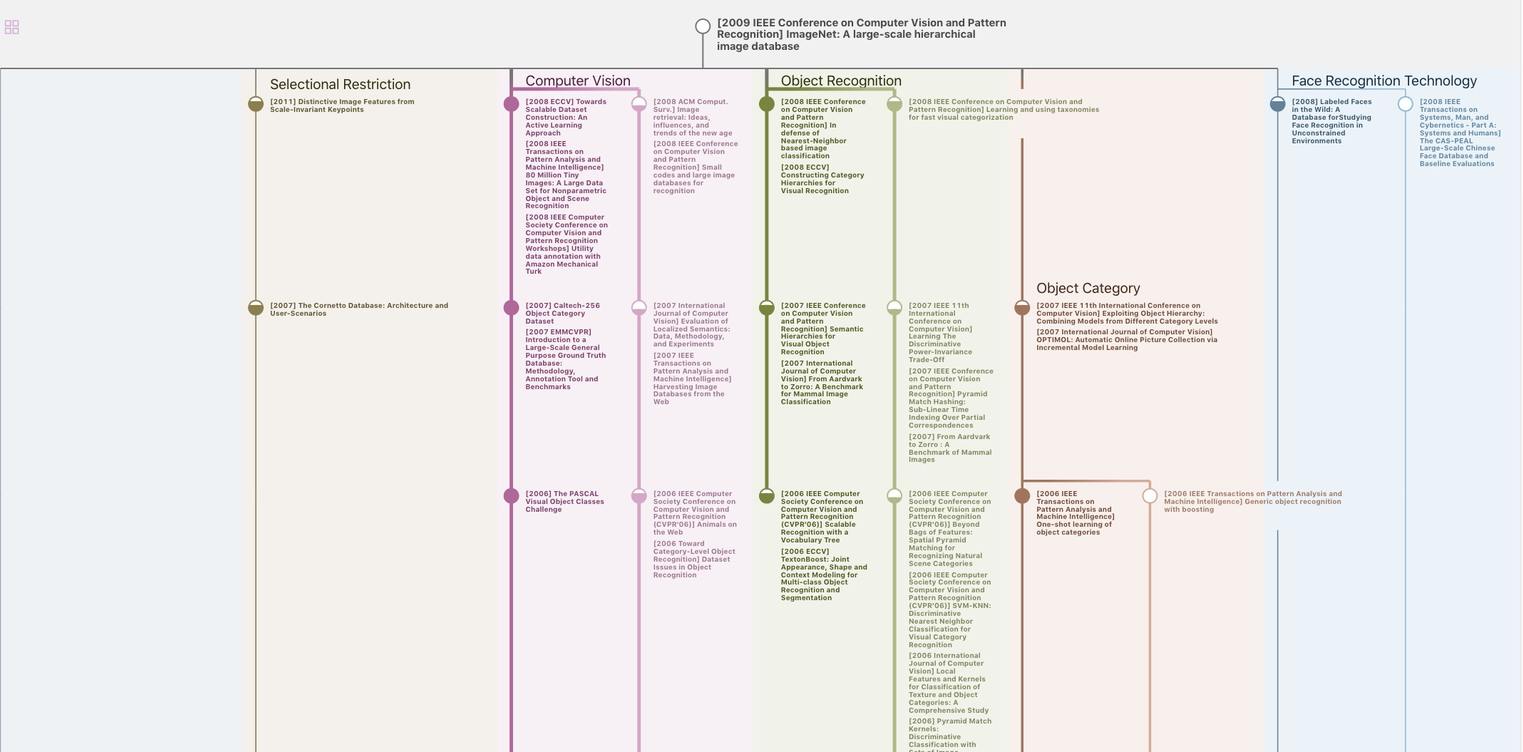
生成溯源树,研究论文发展脉络
Chat Paper
正在生成论文摘要