A Taxonomy of Recurrent Learning Rules
ARTIFICIAL NEURAL NETWORKS AND MACHINE LEARNING - ICANN 2022, PT I(2022)
摘要
Backpropagation through time (BPTT) is the de facto standard for training recurrent neural networks (RNNs), but it is non-causal and non-local. Real-time recurrent learning is a causal alternative, but it is highly inefficient. Recently, e-prop was proposed as a causal, local, and efficient practical alternative to these algorithms, providing an approximation of the exact gradient by radically pruning the recurrent dependencies carried over time. Here, we derive RTRL from BPTT using a detailed notation bringing intuition and clarification to how they are connected. Furthermore, we frame e-prop within in the picture, formalising what it approximates. Finally, we derive a family of algorithms of which e-prop is a special case.
更多查看译文
关键词
Recurrent neural networks, Backpropagation through time, Real-time recurrent learning, Forward propagation, E-prop
AI 理解论文
溯源树
样例
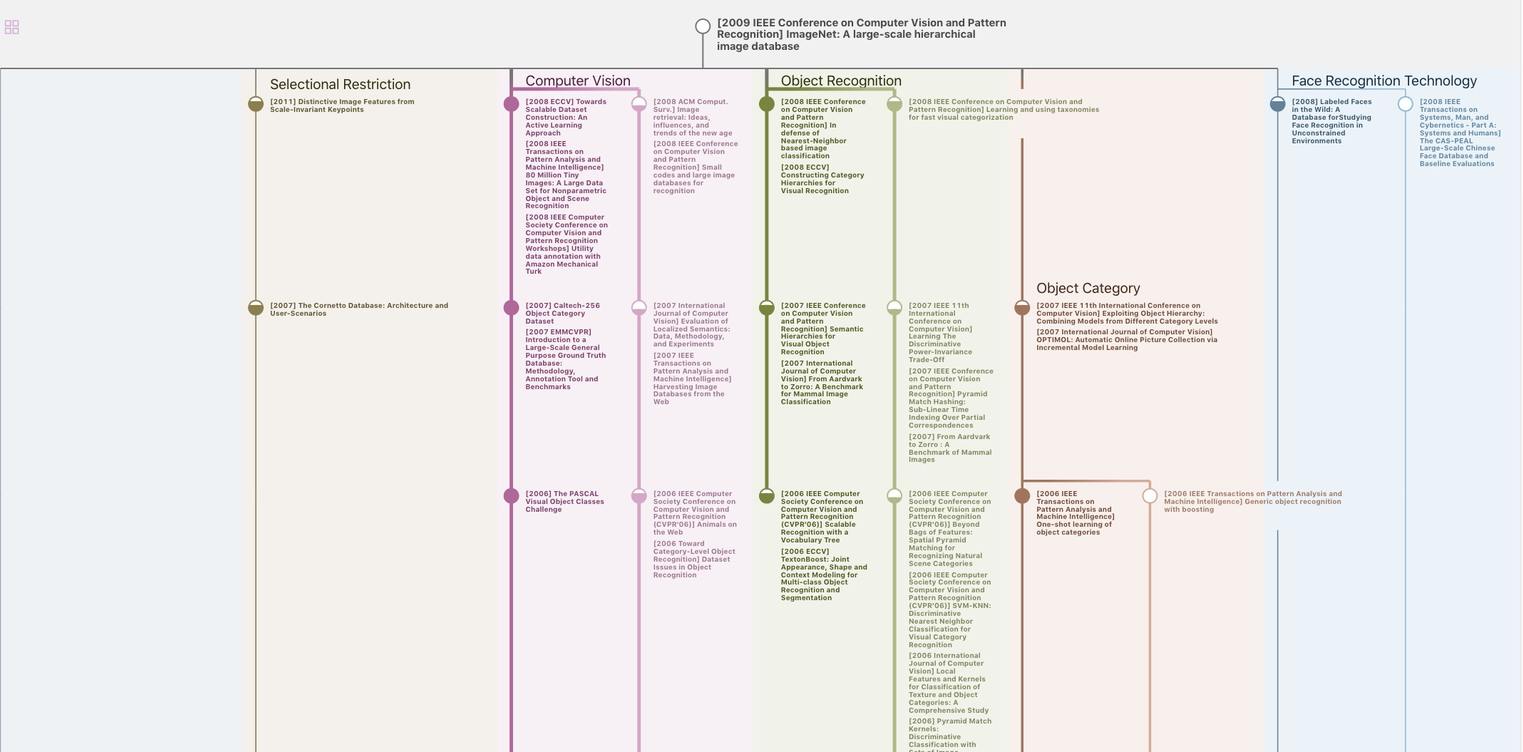
生成溯源树,研究论文发展脉络
Chat Paper
正在生成论文摘要