Improving Neural Abstractive Summarization with Reliable Sentence Sampling
Communications in Computer and Information Science Data Analytics and Management in Data Intensive Domains(2022)
摘要
State-of-the-art abstractive summarization models are able to produce summaries for various types of sources with quality comparable to human written texts. However, despite the fluency, the generated summaries are often erroneous due to factual inconsistencies caused by neural hallucinations. In this work, we study possible ways of reducing the hallucination rate during abstractive summarization. We compare three different techniques aimed at improving the correctness of the training procedure: control tokens, truncated loss, and dataset cleaning. To control hallucination rate outside of the training, we propose an improved algorithm for summary sampling - reliable sentence sampling. The algorithm utilizes fact precision metrics to sample the most reliable sentences for an abstractive summary. By conducting the human evaluation, we demonstrate the algorithm’s efficiency in preserving summary factual consistency.
更多查看译文
关键词
neural abstractive summarization
AI 理解论文
溯源树
样例
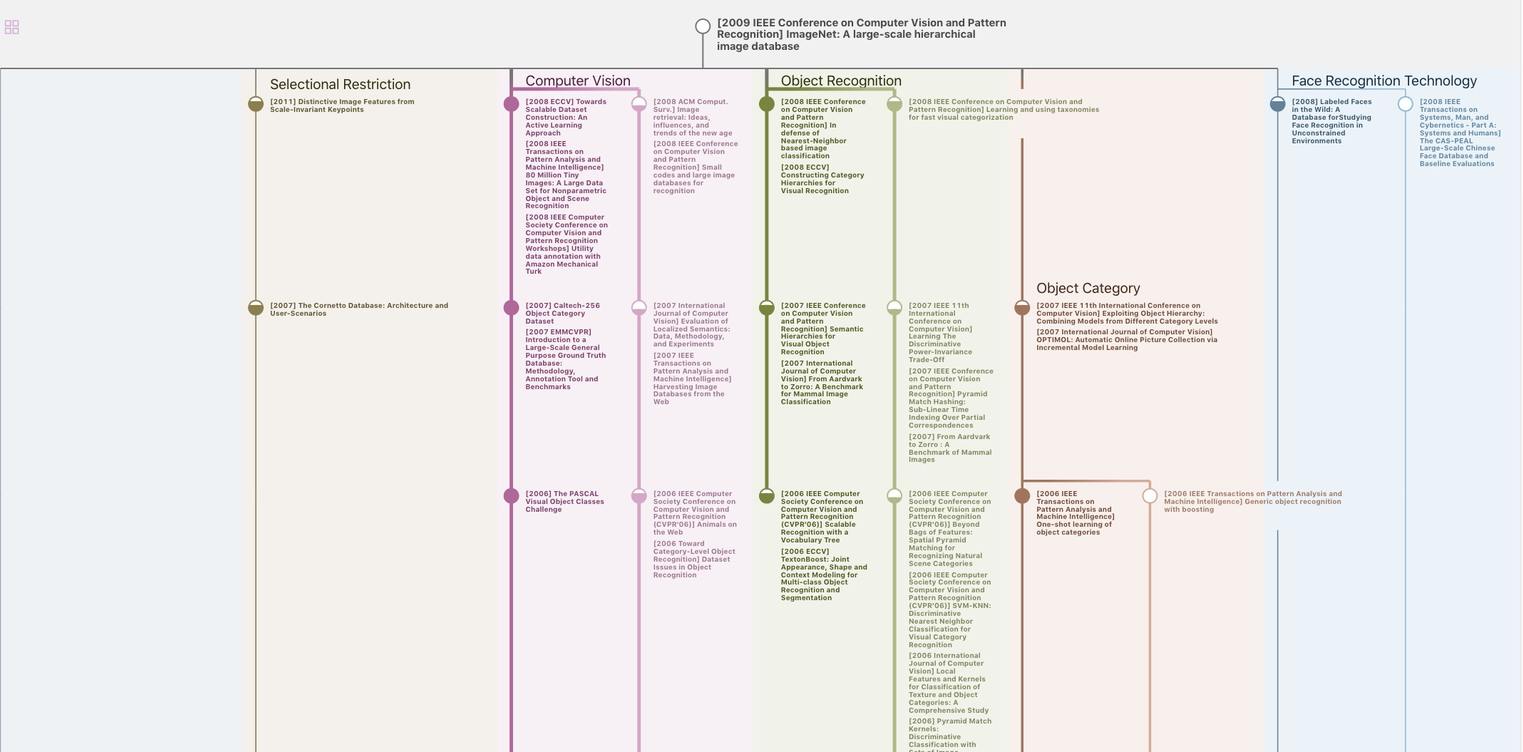
生成溯源树,研究论文发展脉络
Chat Paper
正在生成论文摘要