On Extractive Summarization for Profile-centric Neural Expert Search in Academia
SIGIR '22: Proceedings of the 45th International ACM SIGIR Conference on Research and Development in Information Retrieval(2022)
摘要
Identifying academic experts is crucial for the progress of science, enabling researchers to connect, form networks, and collaborate on the most pressing research problems. A key challenge for ranking experts in response to a query is how to infer their expertise from the publications they coauthored. Profile-centric approaches represent candidate experts by concatenating all their publications into a text-based profile. Despite offering a complete picture of each candidate's scientific output, such lengthy profiles make it inefficient to leverage state-of-the-art neural architectures for inferring expertise. To overcome this limitation, we investigate the suitability of extractive summarization as a mechanism to reduce candidate profiles for semantic encoding using Transformers. Our thorough experiments with a representative academic search test collection demonstrate the benefits of encoding summarized profiles for an improved expertise inference.
更多查看译文
关键词
Expert Search, Deep Learning, Information Retrieval, Extractive Summarization
AI 理解论文
溯源树
样例
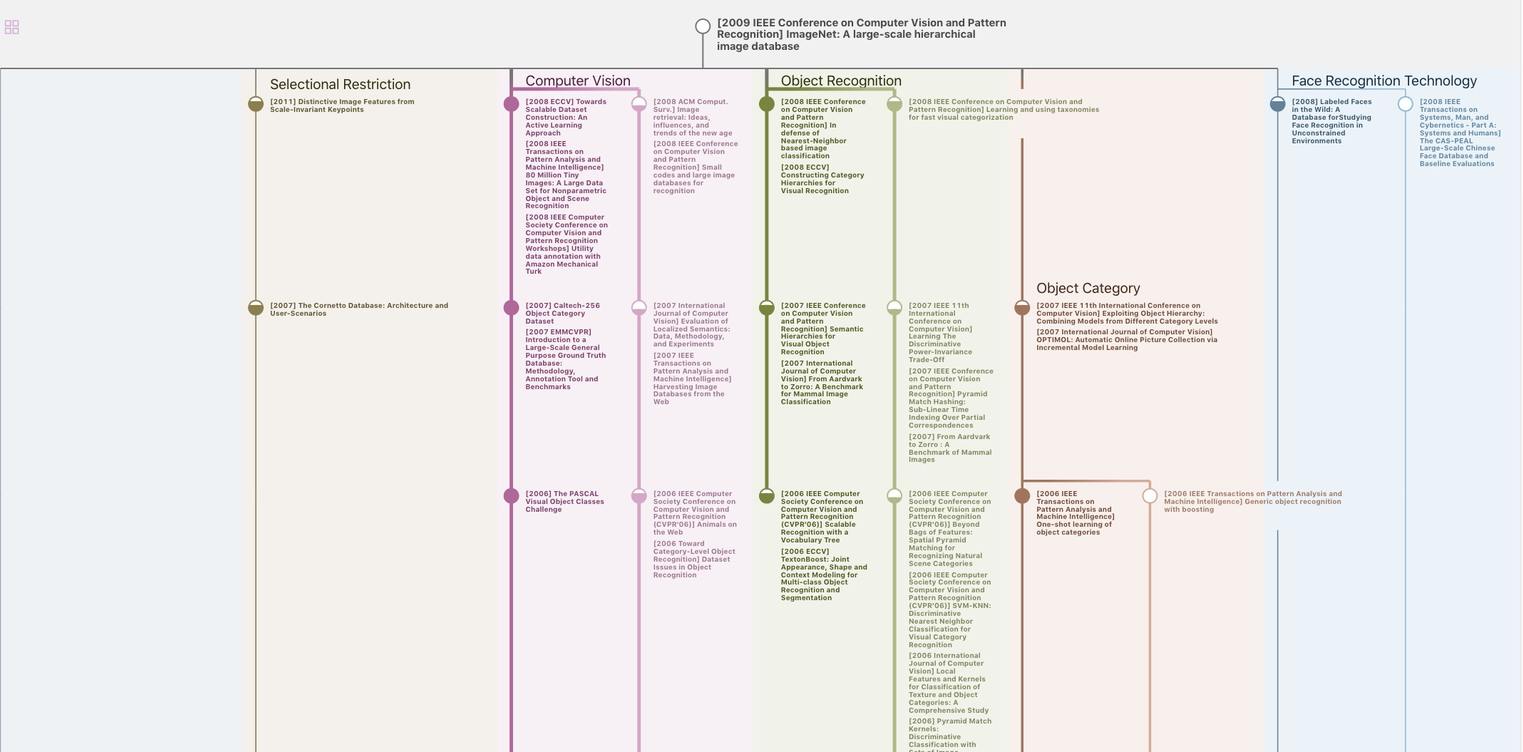
生成溯源树,研究论文发展脉络
Chat Paper
正在生成论文摘要