Diversity Vs Relevance: A Practical Multi-objective Study in Luxury Fashion Recommendations
SIGIR '22: Proceedings of the 45th International ACM SIGIR Conference on Research and Development in Information Retrieval(2022)
摘要
Personalized algorithms focusing uniquely on accuracy might provide highly relevant recommendations, but the recommended items could be too similar to current users' preferences. Therefore, recommenders might prevent users from exploring new products and brands (filter bubbles). This is especially critical for luxury fashion recommendations because luxury shoppers expect to discover exclusive and rare items. Thus, recommender systems for fashion need to consider diversity and elevate the shopping experience by recommending new brands and products from the catalog. In this work, we explored a handful of diversification strategies to rerank the output of a relevance-focused recommender system. Subsequently, we conducted a multi-objective offline experiment optimizing for relevance and diversity simultaneously. We measured diversity with commonly used metrics such as coverage, serendipity, and neighborhood distance, whereas, for relevance, we selected ranking metrics such as recall. The best diversification strategy offline improved user engagement by 2% in click-through rate and presented an uplift of 46% in distinct brands recommended when AB tested against real users. These results reinforced the importance of considering accuracy and diversity metrics when developing a recommender system.
更多查看译文
关键词
diversity, recommender systems, multi-objective optimization, offline evaluation, fashion, e-commerce
AI 理解论文
溯源树
样例
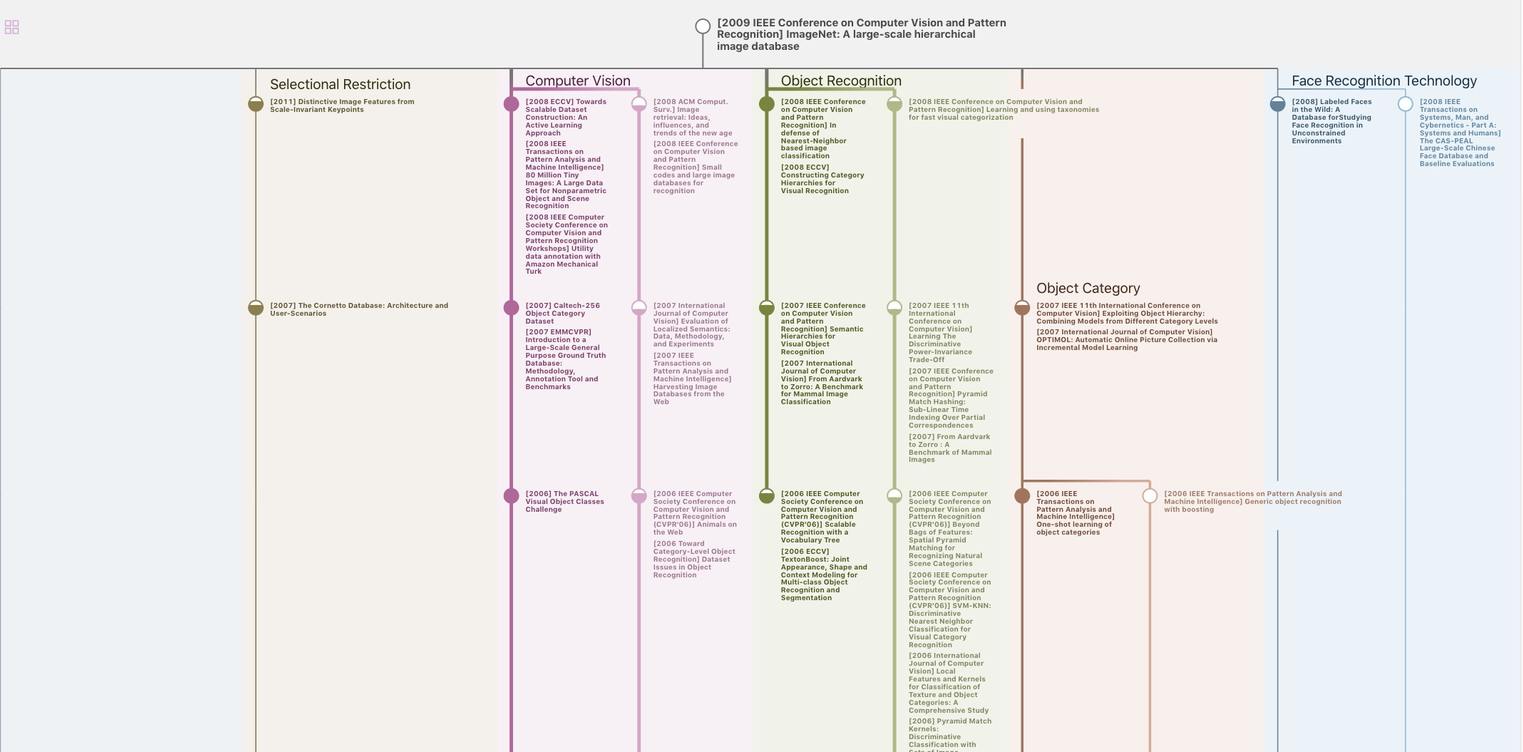
生成溯源树,研究论文发展脉络
Chat Paper
正在生成论文摘要