Neutralizing Popularity Bias in Recommendation Models
SIGIR '22: Proceedings of the 45th International ACM SIGIR Conference on Research and Development in Information Retrieval(2022)
摘要
Most existing recommendation models learn vectorized representations for items, i.e., item embeddings to make predictions. Item embeddings inherit popularity bias from the data, which leads to biased recommendations. We use this observation to design two simple and effective strategies, which can be flexibly plugged into different backbone recommendation models, to learn popularity neutral item representations. One strategy isolates popularity bias in one embedding direction and neutralizes the popularity direction post-training. The other strategy encourages all embedding directions to be disentangled and popularity neutral. We demonstrate that the proposed strategies outperform state-of-the-art debiasing methods on various real-world datasets, and improve recommendation quality of shallow and deep backbone models.
更多查看译文
关键词
recommender systems, popularity bias, disentangled representation
AI 理解论文
溯源树
样例
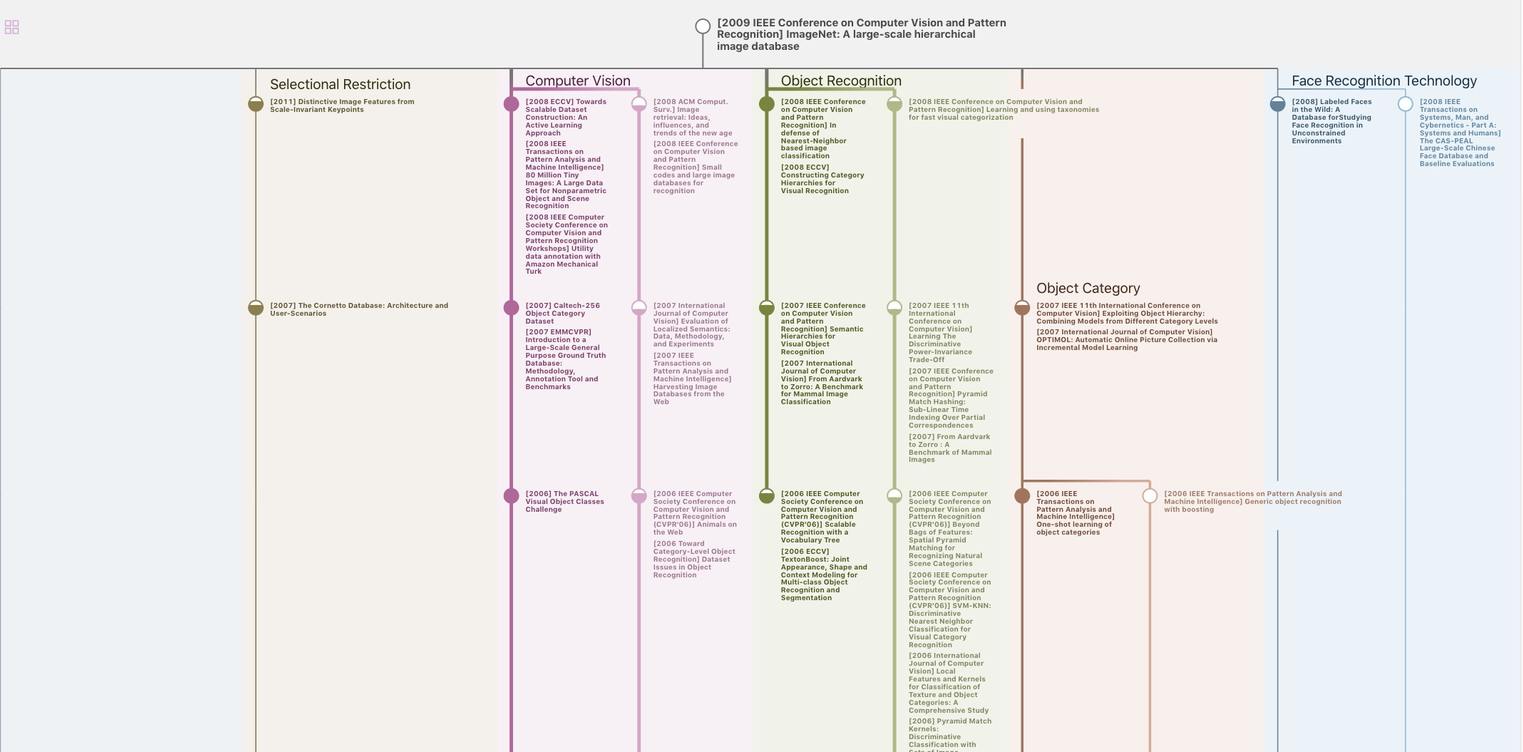
生成溯源树,研究论文发展脉络
Chat Paper
正在生成论文摘要