Attention-Fused CNN Model Compression with Knowledge Distillation for Brain Tumor Segmentation
MEDICAL IMAGE UNDERSTANDING AND ANALYSIS, MIUA 2022(2022)
摘要
3D brain tumor segmentation is an indispensable part of computer-aided detection systems, which is a very time-consuming and error-prone task. In recent years, many deep learning methods, such as U-net and DeepMedic, have been developed to reduce the burden on physicians and improve segmentation accuracy. Particularly with the transformer, an attention-based approach, the segmentation accuracy has been improved. However, transformer-type methods are computationally heavy and require workstations accelerated with graphic process units for fast inference. In this paper, we fuse attention block and convolutional neural network (CNN) with transformer connection to improve segmentation. Meantime, we compress the model and preserve the accuracy with the knowledge distillation technique. We investigated the knowledge distilling efficiency between different architectures. We found the knowledge from the attention-CNN fused model could improve the accuracy of the compressed U-net model. With the knowledge distillation, the compressed U-net architecture could achieve comparable accuracy with around only 11% parameters of the original U-net architecture. As for the compressed attention-CNN fused model, the knowledge from the feature attention combining the knowledge from soft target work better than soft target only. This work would help researchers to compress their model architecture properly.
更多查看译文
关键词
Knowledge distillation,Transformer connection,Brain tumor segmentation
AI 理解论文
溯源树
样例
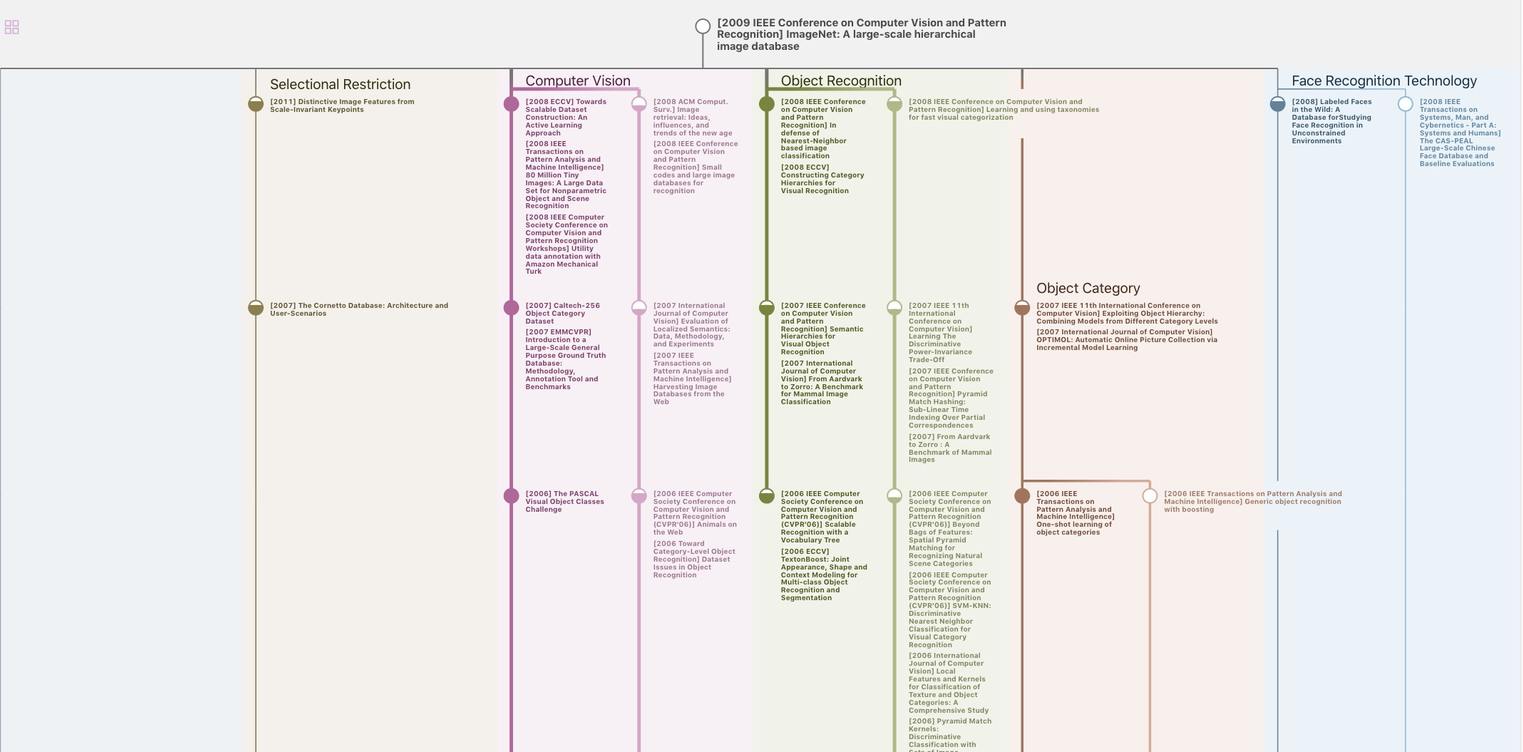
生成溯源树,研究论文发展脉络
Chat Paper
正在生成论文摘要