Co-training Disentangled Domain Adaptation Network for Leveraging Popularity Bias in Recommenders
SIGIR '22: Proceedings of the 45th International ACM SIGIR Conference on Research and Development in Information Retrieval(2022)
摘要
Recommender system usually faces popularity bias. From the popularity distribution shift perspective, the normal paradigm trained on exposed items (most are hot items) identifies that recommending popular items more frequently can achieve lower loss, thus injecting popularity information into item property embedding, e.g., id embedding. From the long-tail distribution shift perspective, the sparse interactions of long-tail items lead to insufficient learning of them. The resultant distribution discrepancy between hot and long-tail items would not only inherit the bias, but also amplify the bias. Existing work addresses this issue with inverse propensity scoring (IPS) or causal embeddings. However, we argue that not all popularity biases mean bad effects, i.e., some items show higher popularity due to better quality or conform to current trends, which deserve more recommendations. Blindly seeking unbiased learning may inhibit high-quality or fashionable items. To make better use of the popularity bias, we propose a co-training disentangled domain adaptation network (CD$^2$AN), which can co-train both biased and unbiased models. Specifically, for popularity distribution shift, CD$^2$AN disentangles item property representation and popularity representation from item property embedding. For long-tail distribution shift, we introduce additional unexposed items (most are long-tail items) to align the distribution of hot and long-tail item property representations. Further, from the instances perspective, we carefully design the item similarity regularization to learn comprehensive item representation, which encourages item pairs with more effective co-occurrences patterns to have more similar item property representations. Based on offline evaluations and online A/B tests, we show that CD$^2$AN outperforms the existing debiased solutions. Currently, CD$^2$AN has been successfully deployed at Mobile Taobao App and handling major online traffic.
更多查看译文
关键词
Recommender Systems, Popularity Bias, Domain Adaptation
AI 理解论文
溯源树
样例
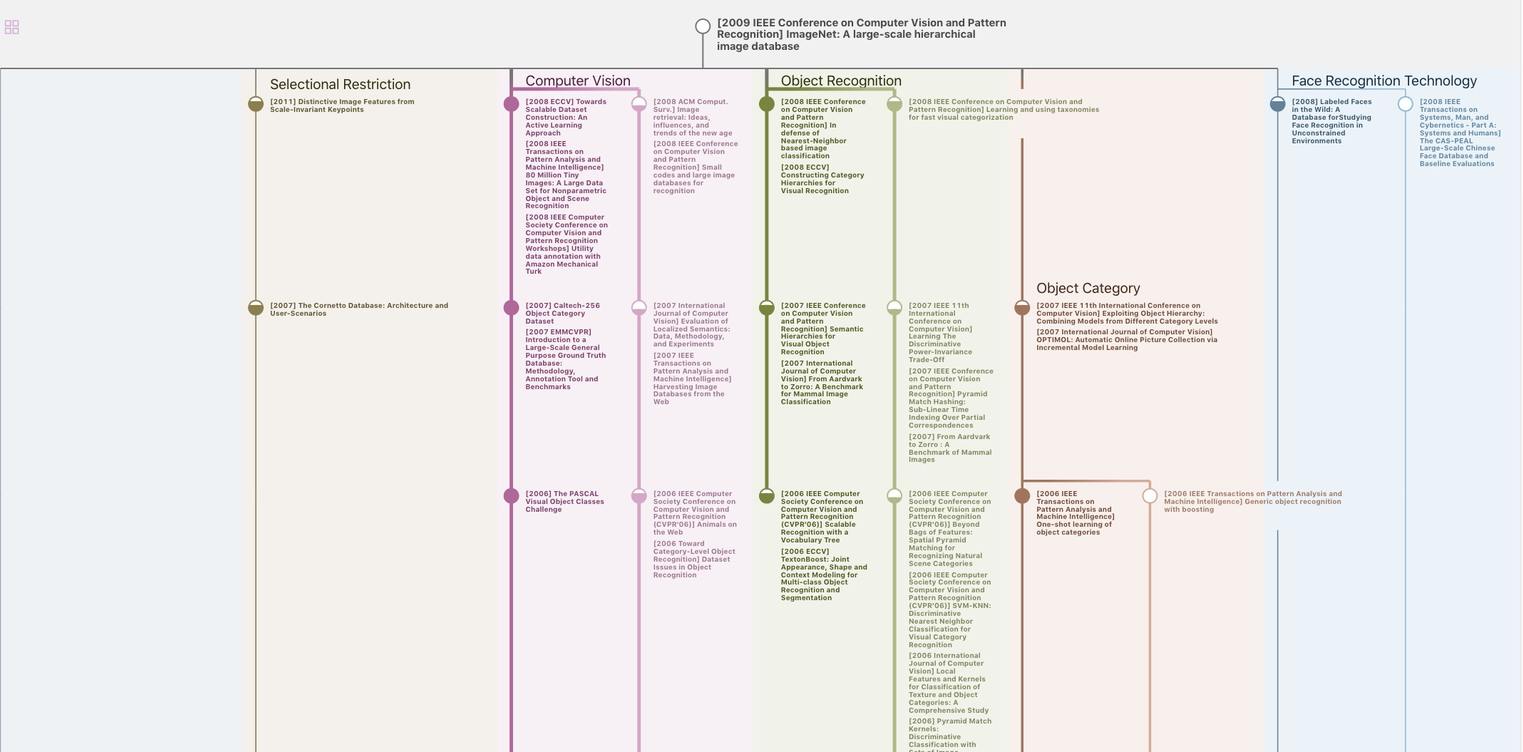
生成溯源树,研究论文发展脉络
Chat Paper
正在生成论文摘要