Co-clustering Interactions via Attentive Hypergraph Neural Network
SIGIR '22: Proceedings of the 45th International ACM SIGIR Conference on Research and Development in Information Retrieval(2022)
摘要
With the rapid growth of interaction data, many clustering methods have been proposed to discover interaction patterns as prior knowledge beneficial to downstream tasks. Considering that an interaction can be seen as an action occurring among multiple objects, most existing methods model the objects and their pair-wise relations as nodes and links in graphs. However, they only model and leverage part of the information in real entire interactions, i.e., either decompose the entire interaction into several pair-wise sub-interactions for simplification, or only focus on clustering some specific types of objects, which limits the performance and explainability of clustering. To tackle this issue, we propose to Co-cluster the Interactions via Attentive Hypergraph neural network (CIAH). Particularly, with more comprehensive modeling of interactions by hypergraph, we propose an attentive hypergraph neural network to encode the entire interactions, where an attention mechanism is utilized to select important attributes for explanations. Then, we introduce a salient method to guide the attention to be more consistent with real importance of attributes, namely saliency-based consistency. Moreover, we propose a novel co-clustering method to perform a joint clustering for the representations of interactions and the corresponding distributions of attribute selection, namely cluster-based consistency. Extensive experiments demonstrate that our CIAH significantly outperforms state-of-the-art clustering methods on both public datasets and real industrial datasets.
更多查看译文
关键词
Graph Neural Networks, Hypergraph, Clustering, Interaction Data
AI 理解论文
溯源树
样例
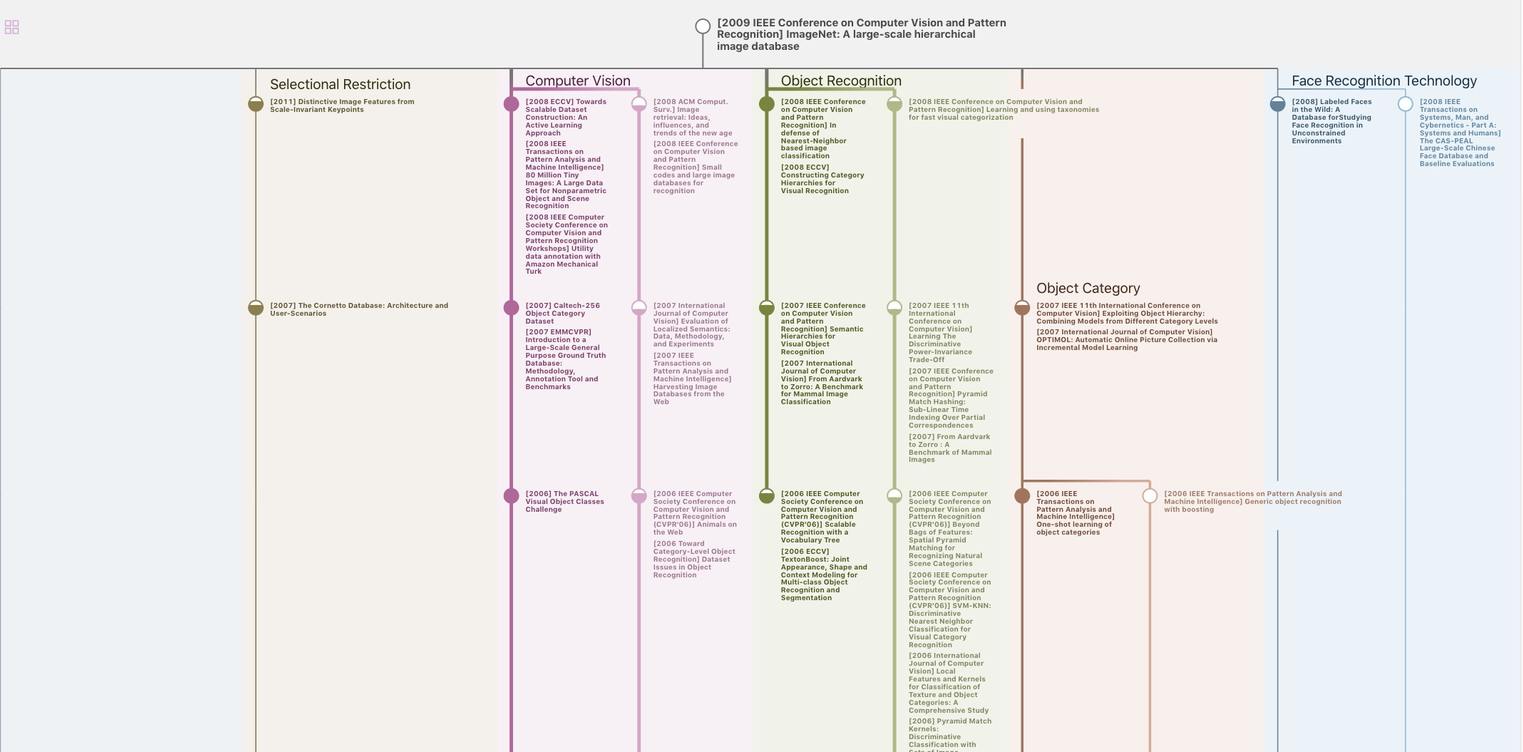
生成溯源树,研究论文发展脉络
Chat Paper
正在生成论文摘要