A Deep-Learning Lesion Segmentation Model that Addresses Class Imbalance and Expected Low Probability Tissue Abnormalities in Pre and Postoperative Liver MRI
MEDICAL IMAGE UNDERSTANDING AND ANALYSIS, MIUA 2022(2022)
摘要
Class imbalance in various forms is a common challenge in machine learning (ML) applied to medical imaging. One of these forms is the presence of low probability, but unsurprising, tissue abnormalities as a result of e.g. implants and surgery. Assessments from automated methods can be impeded if the ML system cannot address these abnormalities. A context where this issue arises is segmentation of lesions within the liver when postoperative scans are possible inputs to the model, since surgical clips and postoperative seromas can distort measures such as future liver remnant volume if they are not correctly identified. To this end, we developed a deep learning segmentation model with classes that expliciltly include surgery-related structures: liver parenchyma, lesions, surgical clips, and postoperative seromas. Given a heavy class imbalance in this task, we deployed an asymmetric focal loss function and hysteresis thresholding post-processing. We applied our model to T1-weighted MRI data, reporting average Dice scores of 0.96, 0.57, 0.71, and 0.84 for the four classes, respectively. Finally, we tested the model's potential in a semi-automatic workflow, finding a user-interaction speedup and an increased inter-rater agreement compared to fully manual delineations. To our knowledge, this is the first study to investigate an automated lesion segmentation model for postoperative MRI with both surgical clips and seromas as explicit classes, and the first work to explore an asymmetric focal loss function for segmentation in liver cancer.
更多查看译文
关键词
Liver cancer,Deep learning segmentation,Hepatectomy,Asymmetric focal loss,Hysteresis thresholding,Semi-automatic workflows
AI 理解论文
溯源树
样例
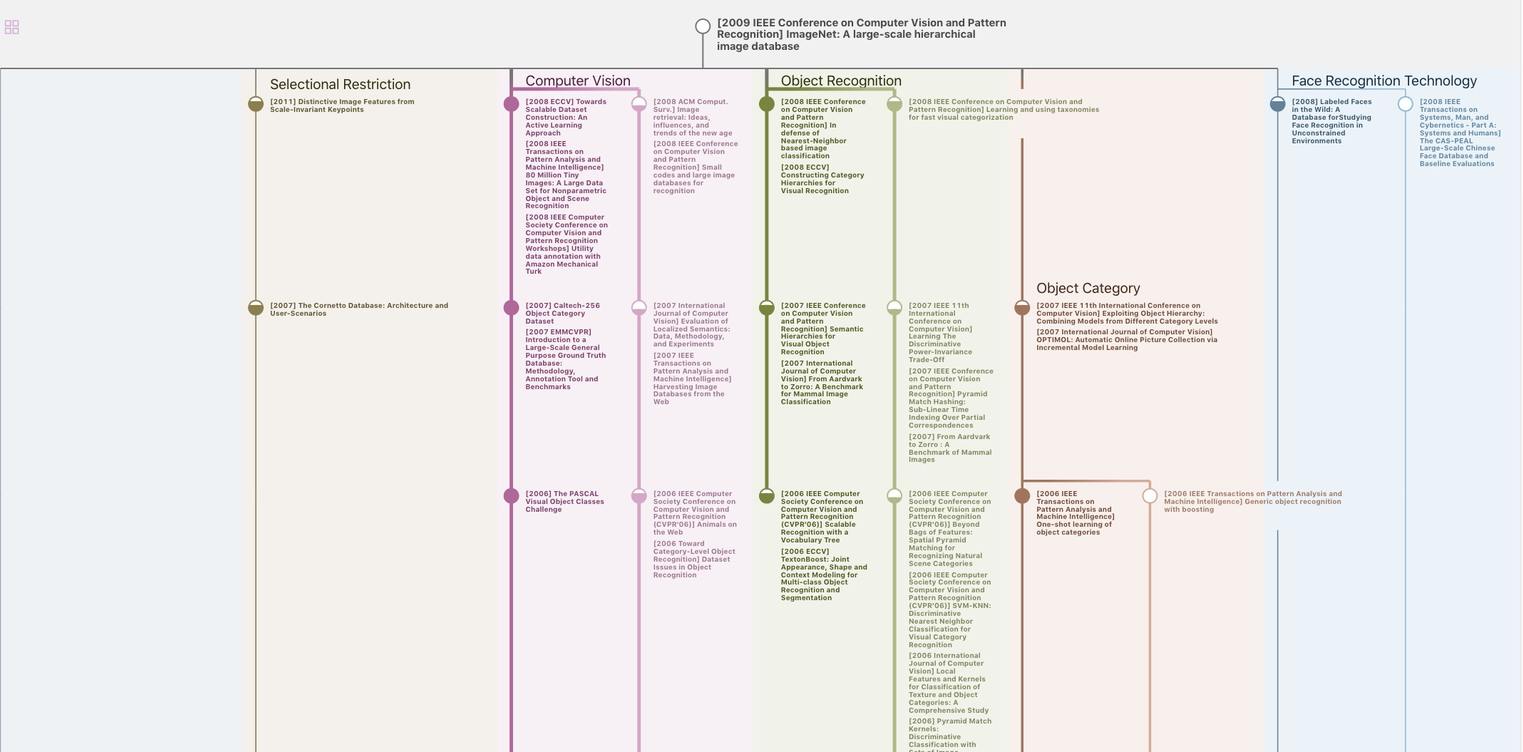
生成溯源树,研究论文发展脉络
Chat Paper
正在生成论文摘要