A Hybrid-Order Spectral-Spatial Feature Network for Hyperspectral Image Classification
REMOTE SENSING(2022)
摘要
Convolutional neural networks are widely applied in hyperspectral image (HSI) classification and show excellent performance. However, there are two challenges: the first is that fine features are generally lost in the process of depth transfer; the second is that most existing studies usually restore to first-order features, whereas they rarely consider second-order representations. To tackle the above two problems, this article proposes a hybrid-order spectral-spatial feature network (HS(2)FNet) for hyperspectral image classification. This framework consists of a precedent feature extraction module (PFEM) and a feature rethinking module (FRM). The former is constructed to capture multiscale spectral-spatial features and focus on adaptively recalibrate channel-wise and spatial-wise feature responses to achieve first-order spectral-spatial feature distillation. The latter is devised to heighten the representative ability of HSI by capturing the importance of feature cross-dimension, while learning more discriminative representations by exploiting the second-order statistics of HSI, thereby improving the classification performance. Massive experiments demonstrate that the proposed network achieves plausible results compared with the state-of-the-art classification methods.
更多查看译文
关键词
hyperspectral image classification,first-order feature,second-order representation,spectral-spatial feature
AI 理解论文
溯源树
样例
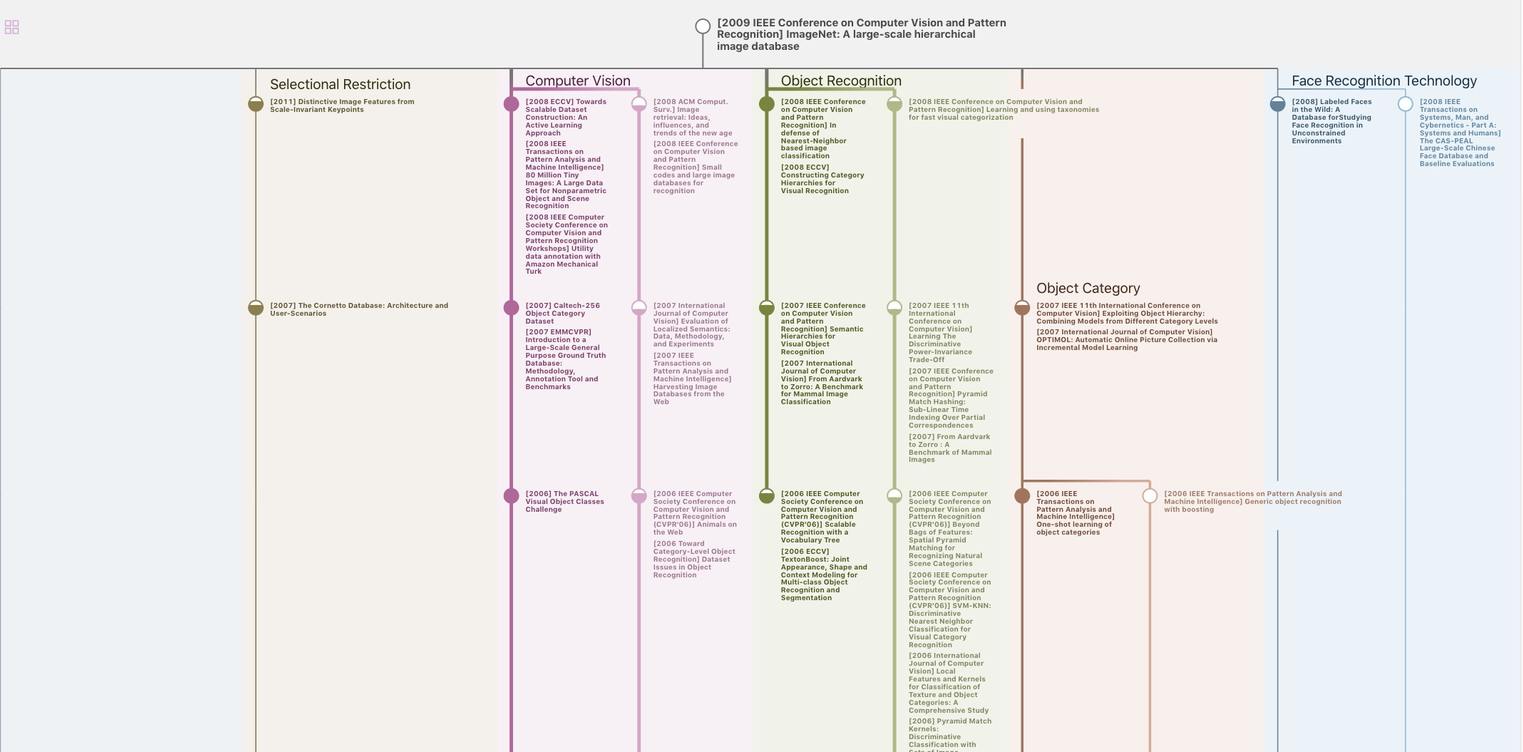
生成溯源树,研究论文发展脉络
Chat Paper
正在生成论文摘要