LKAU-Net: 3D Large-Kernel Attention-Based U-Net for Automatic MRI Brain Tumor Segmentation
MEDICAL IMAGE UNDERSTANDING AND ANALYSIS, MIUA 2022(2022)
摘要
Automatic segmentation of brain tumors from multi-modal magnetic resonance images (MRI) using deep learning methods has emerged in assisting the diagnosis and treatment of brain tumors. However, the diversity in location, shape, and appearance of brain tumors and the dominance of background voxels in MRI images make accurate brain tumor segmentation difficult. In this paper, a novel 3D large-kernel attention-based U-Net (LKAU-Net) is proposed to address these problems for accurate brain tumor segmentation. Advantages of convolution and self-attention are combined in the proposed 3D large-kernel (LK) attention module, including local contextual information, long-range dependence, and channel adaptability simultaneously. The network was trained and evaluated on the multi-modal Brain Tumor Segmentation Challenge (BraTS) 2020 dataset. The effectiveness of the proposed 3D LK attention module has been proved by both five-fold cross-validation and official online evaluation from the organizers. The results show that our LKAU-Net outperformed state-of-the-art performance in delineating all three tumor sub-regions, with average Dice scores of 79.01%, 91.31%, and 85.75%, as well as 95% Hausdorff distances of 26.27, 4.56, and 5.87 for the enhancing tumor, whole tumor, and tumor core, respectively.
更多查看译文
关键词
Brain tumor segmentation,Attention,Deep learning,MRI
AI 理解论文
溯源树
样例
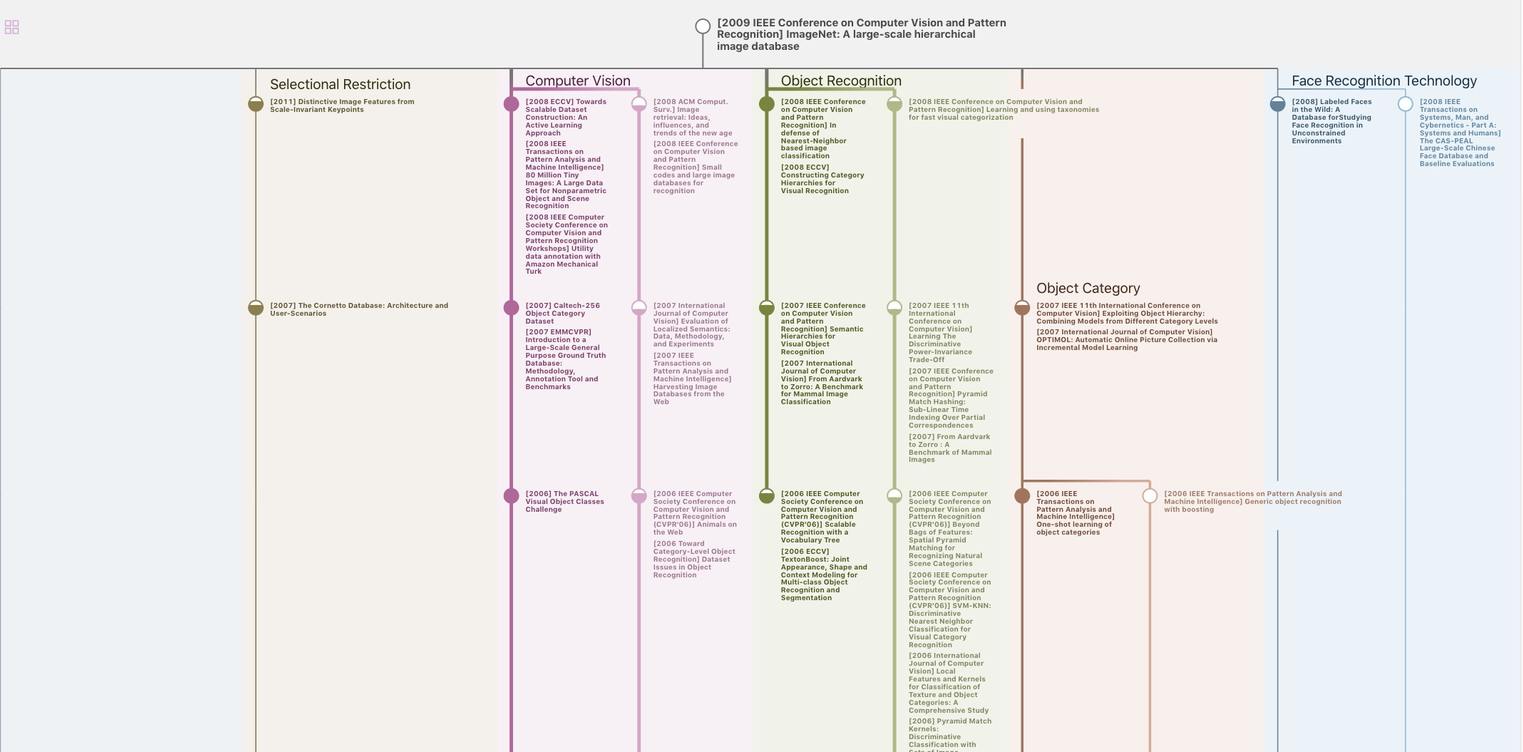
生成溯源树,研究论文发展脉络
Chat Paper
正在生成论文摘要