Spatio-Temporal Deformable Attention Network for Video Deblurring.
European Conference on Computer Vision(2022)
摘要
The key success factor of the video deblurring methods is to compensate for the blurry pixels of the mid-frame with the sharp pixels of the adjacent video frames. Therefore, mainstream methods align the adjacent frames based on the estimated optical flows and fuse the alignment frames for restoration. However, these methods sometimes generate unsatisfactory results because they rarely consider the blur levels of pixels, which may introduce blurry pixels from video frames. Actually, not all the pixels in the video frames are sharp and beneficial for deblurring. To address this problem, we propose the spatio-temporal deformable attention network (STDANet) for video delurring, which extracts the information of sharp pixels by considering the pixel-wise blur levels of the video frames. Specifically, STDANet is an encoder-decoder network combined with the motion estimator and spatio-temporal deformable attention (STDA) module, where motion estimator predicts coarse optical flows that are used as base offsets to find the corresponding sharp pixels in STDA module. Experimental results indicate that the proposed STDANet performs favorably against state-of-the-art methods on the GoPro, DVD, and BSD datasets.
更多查看译文
关键词
Video deblurring, Pixel-wise blur levels, Spatio-temporal deformable attention
AI 理解论文
溯源树
样例
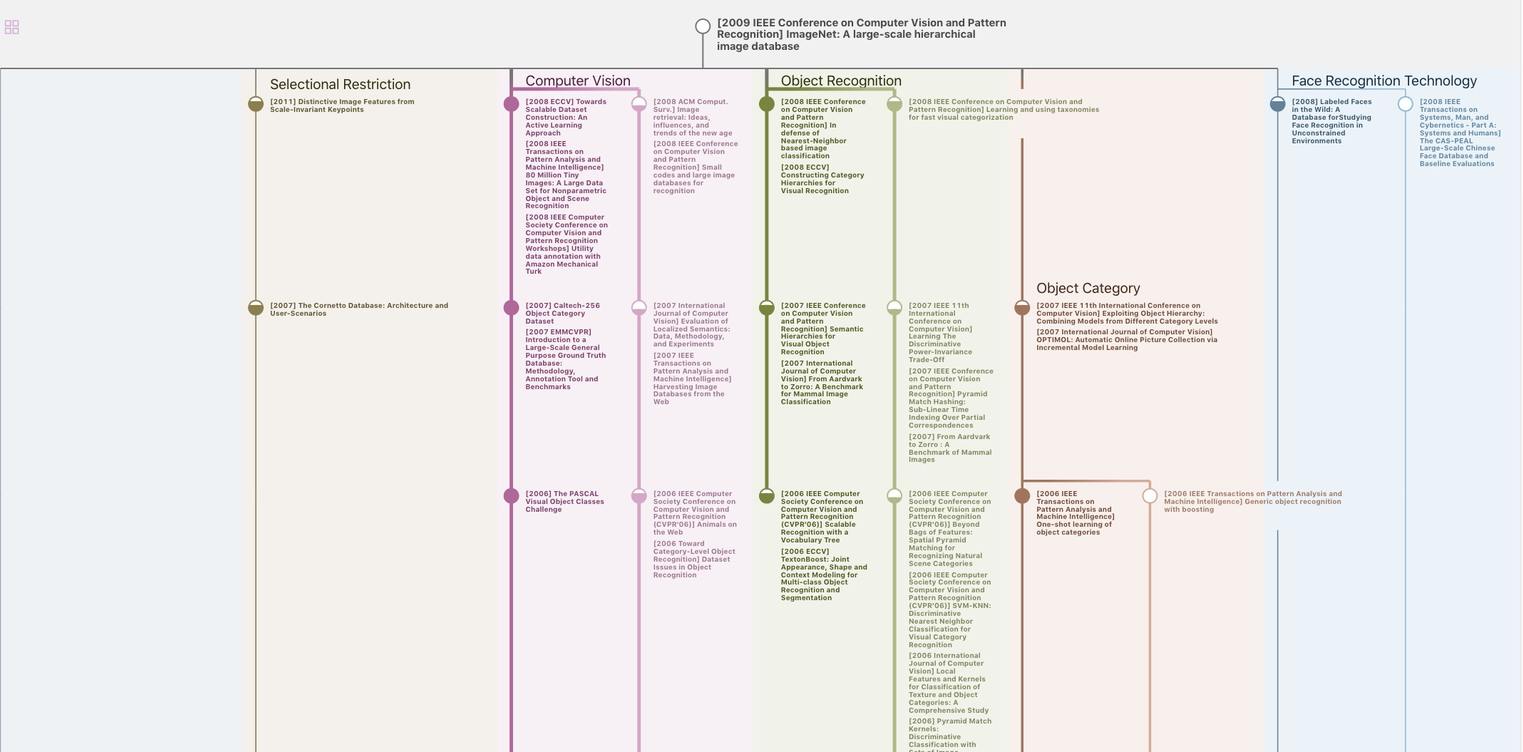
生成溯源树,研究论文发展脉络
Chat Paper
正在生成论文摘要