FAT: Frequency-Aware Transformation for Bridging Full-Precision and Low-Precision Deep Representations
IEEE TRANSACTIONS ON NEURAL NETWORKS AND LEARNING SYSTEMS(2024)
摘要
Learning low-bitwidth convolutional neural networks (CNNs) is challenging because performance may drop significantly after quantization. Prior arts often quantize the network weights by carefully tuning hyperparameters such as nonuniform stepsize and layerwise bitwidths, which are complicated since the full-and low-precision representations have large discrepancies. This work presents a novel quantization pipeline, named frequency-aware transformation (FAT), that features important benefits: 1) instead of designing complicated quantizers, FAT learns to transform network weights in the frequency domain to remove redundant information before quantization, making them amenable to training in low bitwidth with simple quantizers; 2) FAT readily embeds CNNs in low bitwidths using standard quantizers without tedious hyperparameter tuning and theoretical analyses show that FAT minimizes the quantization errors in both uniform and nonuniform quantizations; and 3) FAT can be easily plugged into various CNN architectures. Using FAT with a simple uniform/logarithmic quantizer can achieve the state-of-the-art performance in different bitwidths on various model architectures. Consequently, FAT serves to provide a novel frequency-based perspective for model quantization.
更多查看译文
关键词
Quantization (signal),Fats,Transforms,Training,Adaptation models,Standards,Frequency-domain analysis,Efficient neural network,model compression,quantization,representation learning
AI 理解论文
溯源树
样例
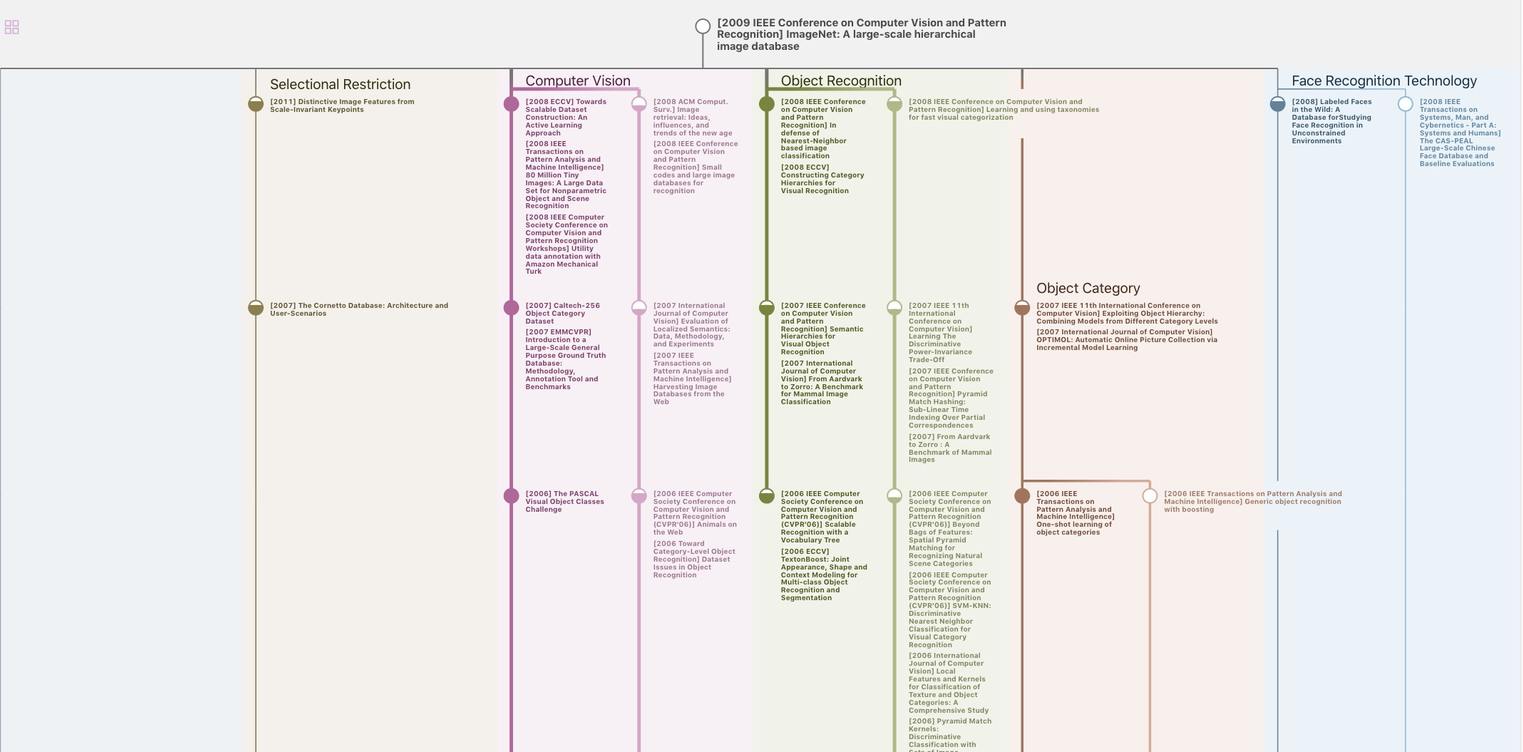
生成溯源树,研究论文发展脉络
Chat Paper
正在生成论文摘要