Prospective, multi-site study of patient outcomes after implementation of the TREWS machine learning-based early warning system for sepsis
NATURE MEDICINE(2022)
摘要
Early recognition and treatment of sepsis are linked to improved patient outcomes. Machine learning-based early warning systems may reduce the time to recognition, but few systems have undergone clinical evaluation. In this prospective, multi-site cohort study, we examined the association between patient outcomes and provider interaction with a deployed sepsis alert system called the Targeted Real-time Early Warning System (TREWS). During the study, 590,736 patients were monitored by TREWS across five hospitals. We focused our analysis on 6,877 patients with sepsis who were identified by the alert before initiation of antibiotic therapy. Adjusting for patient presentation and severity, patients in this group whose alert was confirmed by a provider within 3 h of the alert had a reduced in-hospital mortality rate (3.3%, confidence interval (CI) 1.7, 5.1%, adjusted absolute reduction, and 18.7%, CI 9.4, 27.0%, adjusted relative reduction), organ failure and length of stay compared with patients whose alert was not confirmed by a provider within 3 h. Improvements in mortality rate (4.5%, CI 0.8, 8.3%, adjusted absolute reduction) and organ failure were larger among those patients who were additionally flagged as high risk. Our findings indicate that early warning systems have the potential to identify sepsis patients early and improve patient outcomes and that sepsis patients who would benefit the most from early treatment can be identified and prioritized at the time of the alert
更多查看译文
关键词
Bacterial infection,Machine learning,Biomedicine,general,Cancer Research,Metabolic Diseases,Infectious Diseases,Molecular Medicine,Neurosciences
AI 理解论文
溯源树
样例
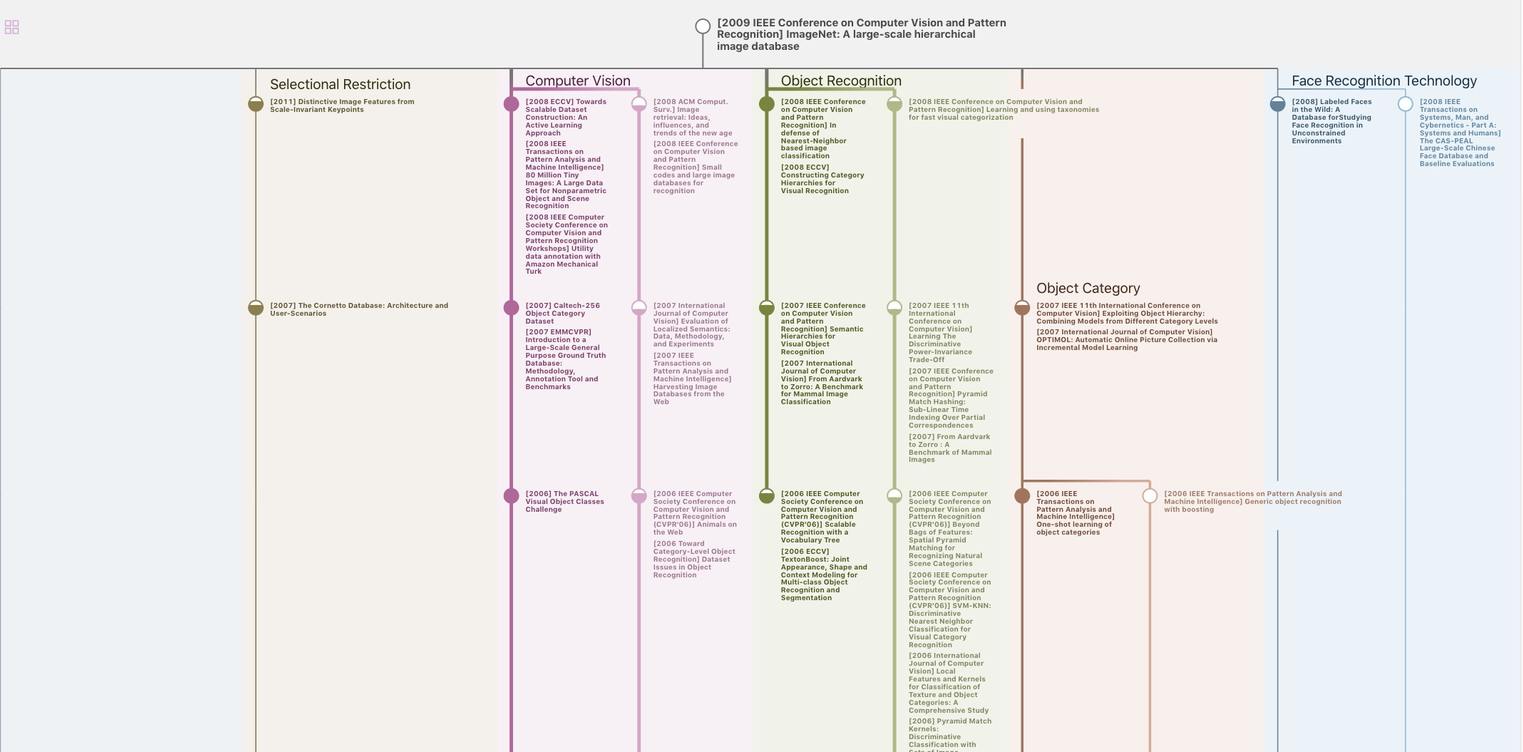
生成溯源树,研究论文发展脉络
Chat Paper
正在生成论文摘要