The MABe22 Benchmarks for Representation Learning of Multi-Agent Behavior
arxiv(2022)
摘要
Real-world behavior is often shaped by complex interactions between multiple agents. To scalably study multi-agent behavior, advances in unsupervised and self-supervised learning have enabled a variety of different behavioral representations to be learned from trajectory data. To date, there does not exist a unified set of benchmarks that can enable comparing methods quantitatively and systematically across a broad set of behavior analysis settings. We aim to address this by introducing a large-scale, multi-agent trajectory dataset from real-world behavioral neuroscience experiments that covers a range of behavior analysis tasks. Our dataset consists of trajectory data from common model organisms, with 9.6 million frames of mouse data and 4.4 million frames of fly data, in a variety of experimental settings, such as different strains, lengths of interaction, and optogenetic stimulation. A subset of the frames also consist of expert-annotated behavior labels. Improvements on our dataset corresponds to behavioral representations that work across multiple organisms and is able to capture differences for common behavior analysis tasks.
更多查看译文
AI 理解论文
溯源树
样例
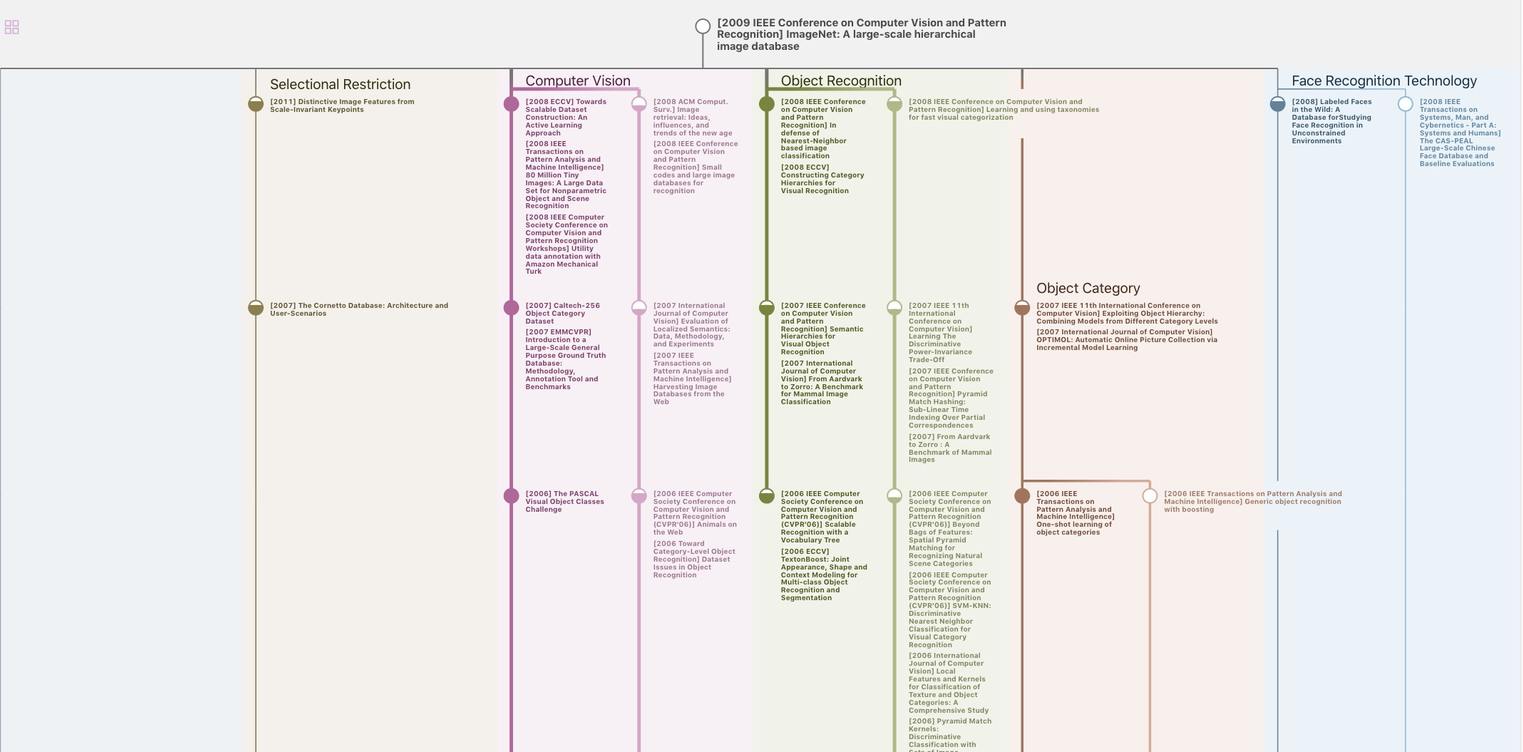
生成溯源树,研究论文发展脉络
Chat Paper
正在生成论文摘要