Sobolev Training for Implicit Neural Representations with Approximated Image Derivatives.
European Conference on Computer Vision(2022)
摘要
Recently, Implicit Neural Representations (INRs) parameterized by neural networks have emerged as a powerful and promising tool to represent different kinds of signals due to its continuous, differentiable properties, showing superiorities to classical discretized representations. However, the training of neural networks for INRs only utilizes input-output pairs, and the derivatives of the target output with respect to the input, which can be accessed in some cases, are usually ignored. In this paper, we propose a training paradigm for INRs whose target output is image pixels, to encode image derivatives in addition to image values in the neural network. Specifically, we use finite differences to approximate image derivatives. We show how the training paradigm can be leveraged to solve typical INRs problems, i.e., image regression and inverse rendering, and demonstrate this training paradigm can improve the data-efficiency and generalization capabilities of INRs. The code of our method is available at https://github.com/megvii-research/Sobolev_INRs.
更多查看译文
关键词
Implicit neural representations,Finite differences,Sobolev training
AI 理解论文
溯源树
样例
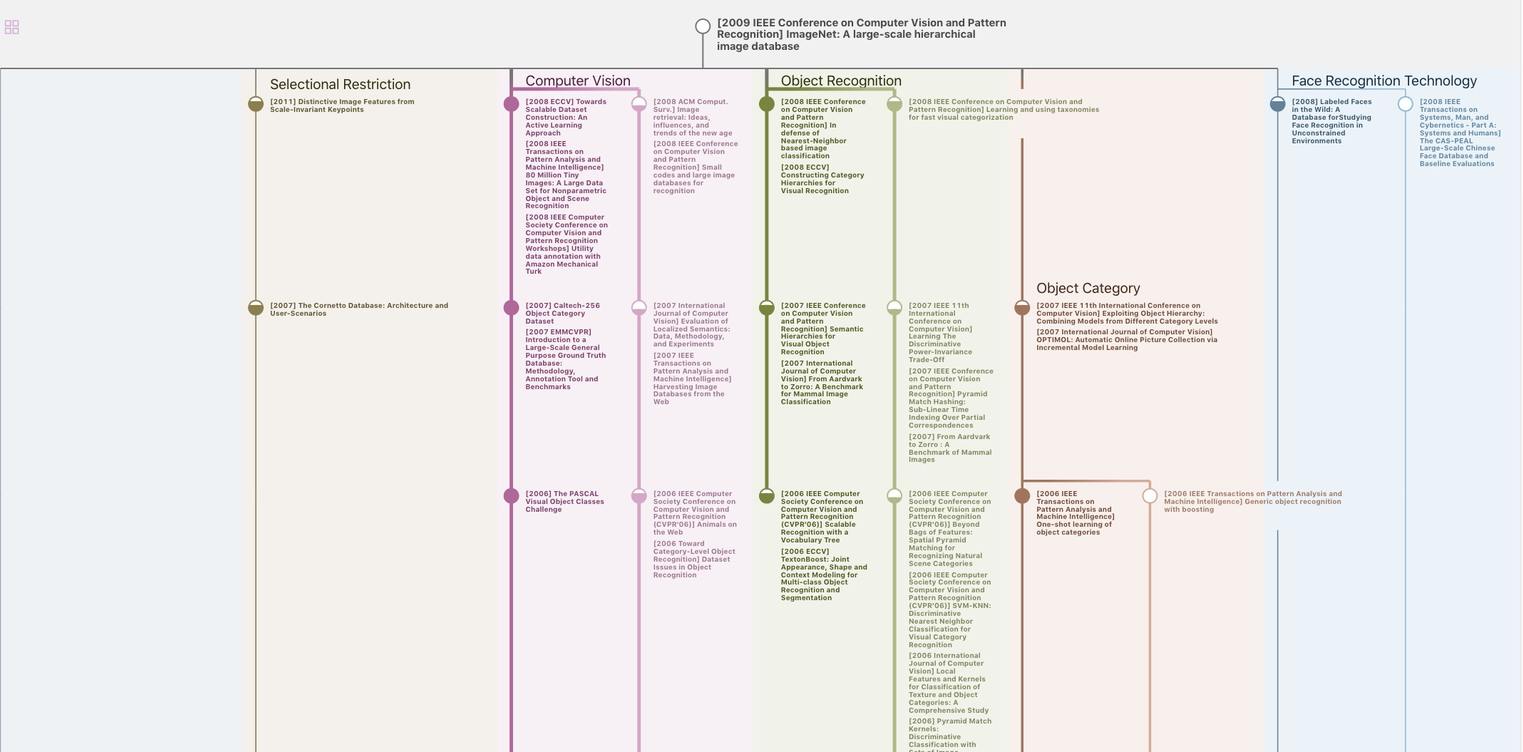
生成溯源树,研究论文发展脉络
Chat Paper
正在生成论文摘要