Lung cancer survival prediction and biomarker identification with an ensemble machine learning analysis of tumor core biopsy metabolomic data
Metabolomics(2022)
摘要
Introduction While prediction of short versus long term survival from lung cancer is clinically relevant in the context of patient management and therapy selection, it has proven difficult to identify reliable biomarkers of survival. Metabolomic markers from tumor core biopsies have been shown to reflect cancer metabolic dysregulation and hold prognostic value. Objectives Implement and validate a novel ensemble machine learning approach to evaluate survival based on metabolomic biomarkers from tumor core biopsies. Methods Data were obtained from tumor core biopsies evaluated with high-resolution 2DLC-MS/MS. Unlike biofluid samples, analysis of tumor tissue is expected to accurately reflect the cancer metabolism and its impact on patient survival. A comprehensive suite of machine learning algorithms were trained as base learners and then combined into a stacked-ensemble meta-learner for predicting “short” versus “long” survival on an external validation cohort. An ensemble method of feature selection was employed to find a reliable set of biomarkers with potential clinical utility. Results Overall survival (OS) is predicted in external validation cohort with AUROC TEST of 0.881 with support vector machine meta learner model, while progression-free survival (PFS) is predicted with AUROC TEST of 0.833 with boosted logistic regression meta learner model, outperforming a nomogram using covariate data (staging, age, sex, treatment vs. non-treatment) as predictors. Increased relative abundance of guanine, choline, and creatine corresponded with shorter OS, while increased leucine and tryptophan corresponded with shorter PFS. In patients that expired, N6,N6,N6-Trimethyl-L-lysine, L-pyrogluatmic acid, and benzoic acid were increased while cystine, methionine sulfoxide and histamine were decreased. In patients with progression, itaconic acid, pyruvate, and malonic acid were increased. Conclusion This study demonstrates the feasibility of an ensemble machine learning approach to accurately predict patient survival from tumor core biopsy metabolomic data.
更多查看译文
关键词
Metabolomics, Lung cancer, Survival prediction, Machine learning, Personalized medicine, Artificial intelligence
AI 理解论文
溯源树
样例
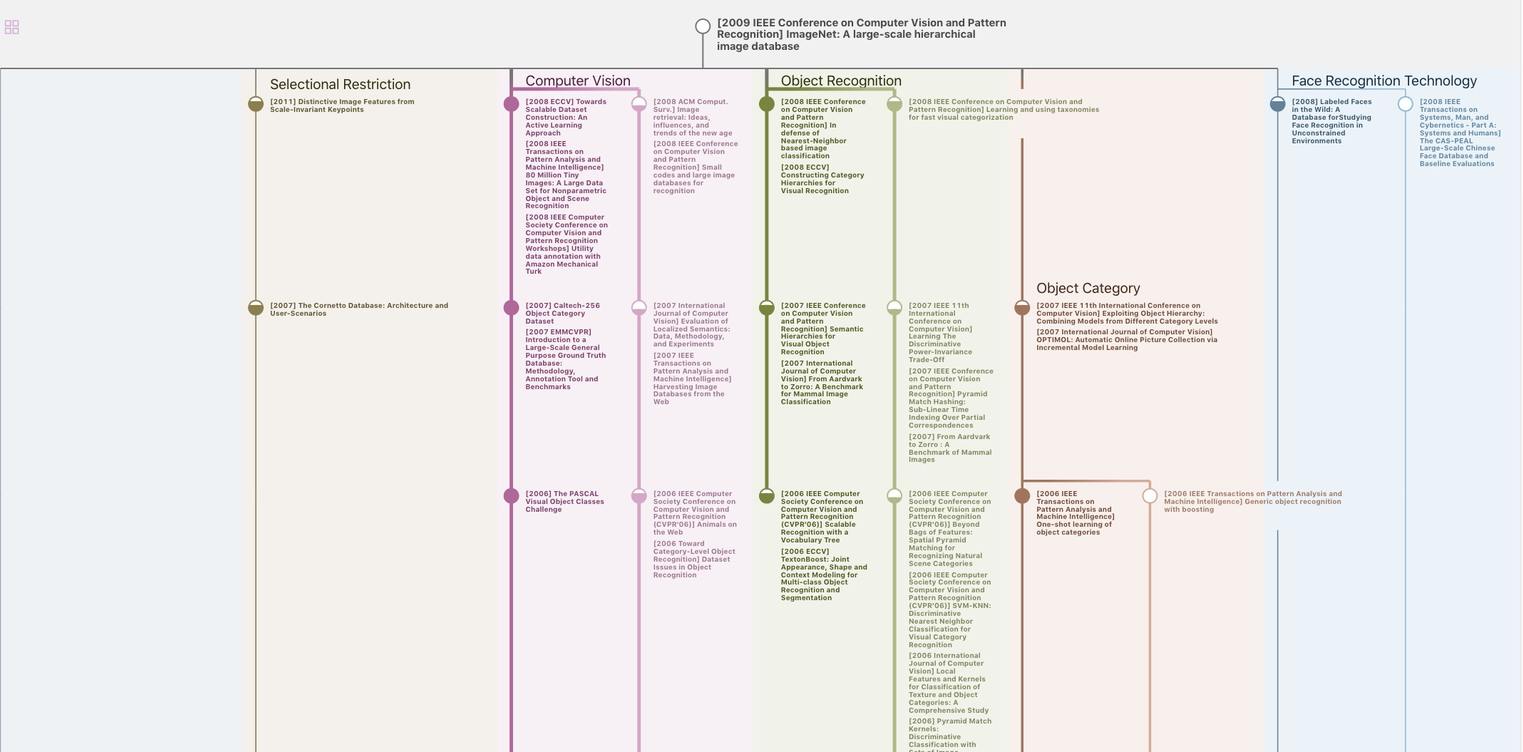
生成溯源树,研究论文发展脉络
Chat Paper
正在生成论文摘要