Testing a filtering strategy for systematic reviews: evaluating work savings and recall.
AMIA ... Annual Symposium proceedings. AMIA Symposium(2022)
摘要
Systematic reviews are extremely time-consuming. The goal of this work is to assess work savings and recall for a publication type filtering strategy that uses the output of two machine learning models, Multi-Tagger and web RCT Tagger, applied retrospectively to 10 systematic reviews on drug effectiveness. Our filtering strategy resulted in mean work savings of 33.6% and recall of 98.3%. Of 363 articles finally included in any of the systematic reviews, 7 were filtered out by our strategy, but 1 "error" was actually an article using a publication type that the SR team had not pre-specified as relevant for inclusion. Our analysis suggests that automated publication type filtering can potentially provide substantial work savings with minimal loss of included articles. Publication type filtering should be personalized for each systematic review and might be combined with other filtering or ranking methods to provide additional work savings for manual triage.
更多查看译文
AI 理解论文
溯源树
样例
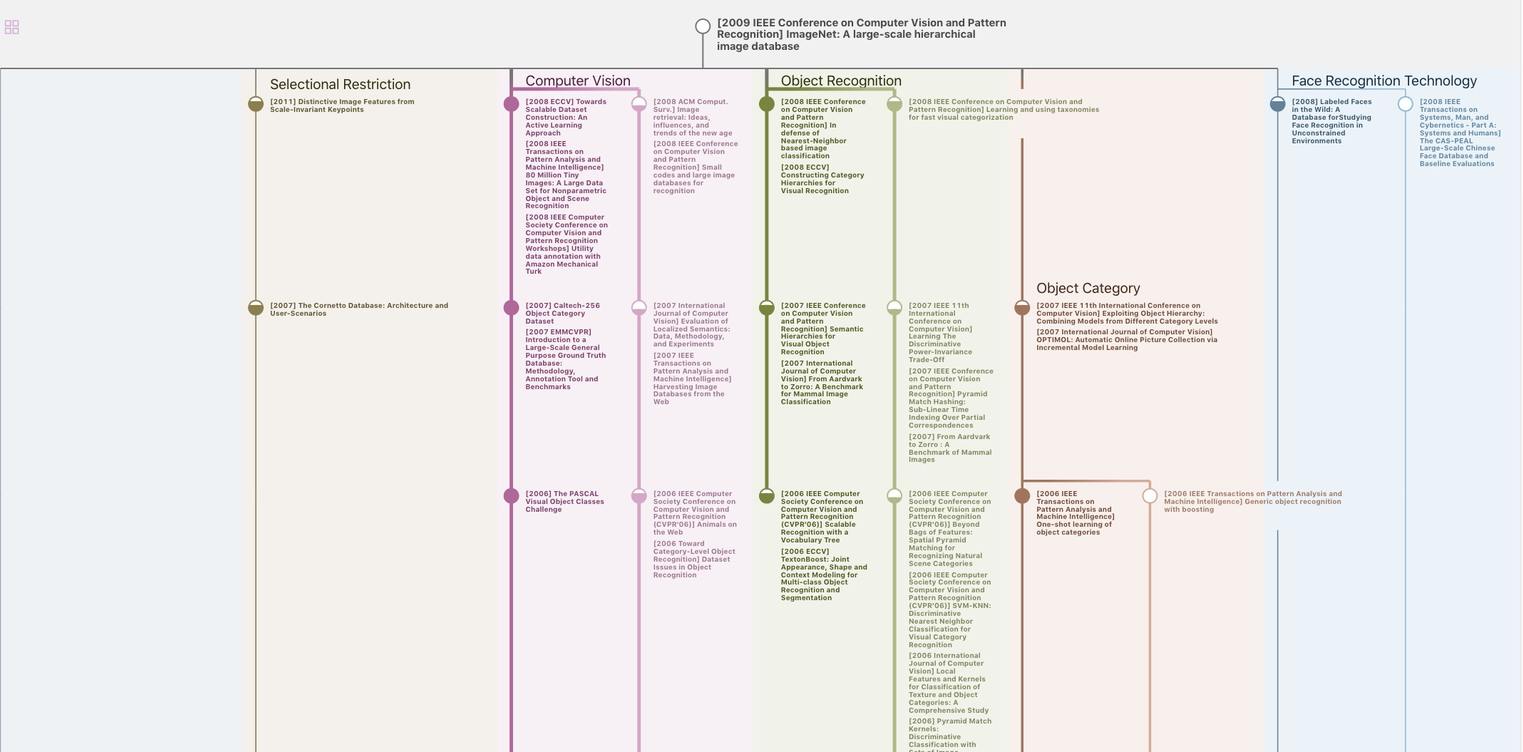
生成溯源树,研究论文发展脉络
Chat Paper
正在生成论文摘要