ADGAN: Attribute-Driven Generative Adversarial Network for Synthesis and Multiclass Classification of Pulmonary Nodules
IEEE TRANSACTIONS ON NEURAL NETWORKS AND LEARNING SYSTEMS(2024)
摘要
Lung cancer is the leading cause of cancer-related deaths worldwide. According to the American Cancer Society, early diagnosis of pulmonary nodules in computed tomography (CT) scans can improve the five-year survival rate up to 70% with proper treatment planning. In this article, we propose an attribute-driven Generative Adversarial Network (ADGAN) for synthesis and multiclass classification of Pulmonary Nodules. A self-attention U-Net (SaUN) architecture is proposed to improve the generation mechanism of the network. The generator is designed with two modules, namely, self-attention attribute module (SaAM) and a self-attention spatial module (SaSM). SaAM generates a nodule image based on given attributes whereas SaSM specifies the nodule region of the input image to be altered. A reconstruction loss along with an attention localization loss (AL) is used to produce an attention map prioritizing the nodule regions. To avoid resemblance between a generated image and a real image, we further introduce an adversarial loss containing a regularization term based on KL divergence. The discriminator part of the proposed model is designed to achieve the multiclass nodule classification task. Our proposed approach is validated over two challenging publicly available datasets, namely LIDC-IDRI and LUNGX. Exhaustive experimentation on these two datasets clearly indicate that we have achieved promising classification accuracy as compared to other state-of-the-art methods.
更多查看译文
关键词
Adversarial network,multiclass nodule classification,nodule synthesis,self-attention U-Net (SaUN)
AI 理解论文
溯源树
样例
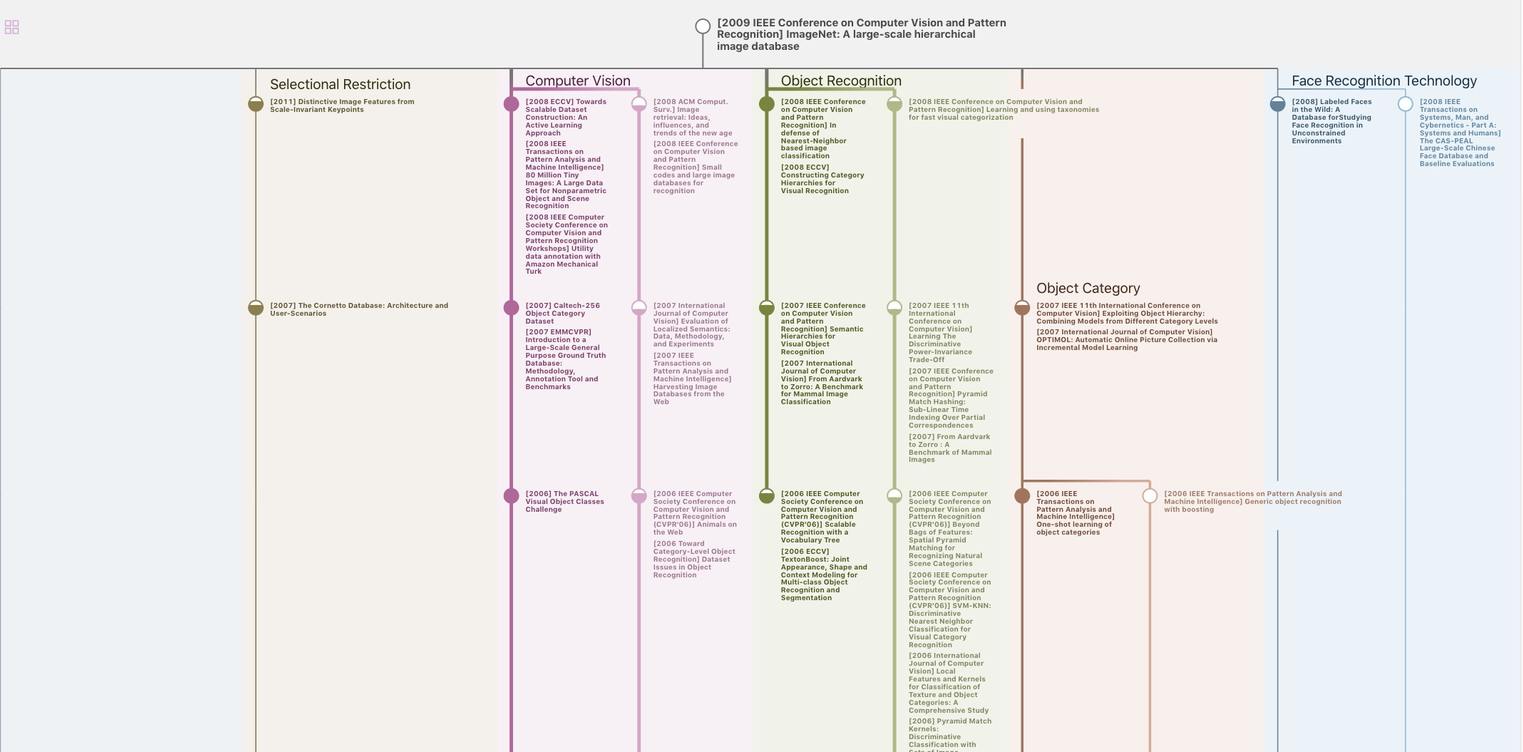
生成溯源树,研究论文发展脉络
Chat Paper
正在生成论文摘要