BRAM-ED: Vehicle Trajectory Prediction Considering the Change of Driving Behavior
IEEE/ASME Transactions on Mechatronics(2022)
摘要
Trajectory prediction plays a key role in the decision-making system of autonomous vehicles. The existing trajectory prediction models have the problem of accuracy deterioration when driving behavior change. To solve this problem, a novel BRAM-ED trajectory prediction framework is proposed, which mainly consists of driving behavior recognition model, behavior attention mechanism (BAM) trajectory encoder, and behavior adaptive future trajectory decoder. First, a Bi-GLSTM network is designed to recognize real-time driving behavior. The graph structure is used to describe the complex dependencies among vehicles and recurrent cell is to capture temporal correlation. Subsequently, in contrast to traditional models, directly concatenate historical trajectories and interactive information as the input of prediction model, BAM is proposed to integrated trajectory and interaction features in this article. The BAM is designed to generate attention weights according to the change of driving behavior, and guide trajectory decoder to predict the future trajectories. Then, the proposed model is trained and validated on various public datasets, including HighD and NGSIM. Compared with existing optimal models, our prediction error has been reduced 44.66% at most. Furthermore, a typical cut-in scenario is designed based on hardware-in-loop platform. The experiment results show that the proposed model could recognize the change of driving behavior timely, and predict accurate trajectory.
更多查看译文
关键词
Behavior attention mechanism (BAM),deep neural network,driving behavior,trajectory prediction
AI 理解论文
溯源树
样例
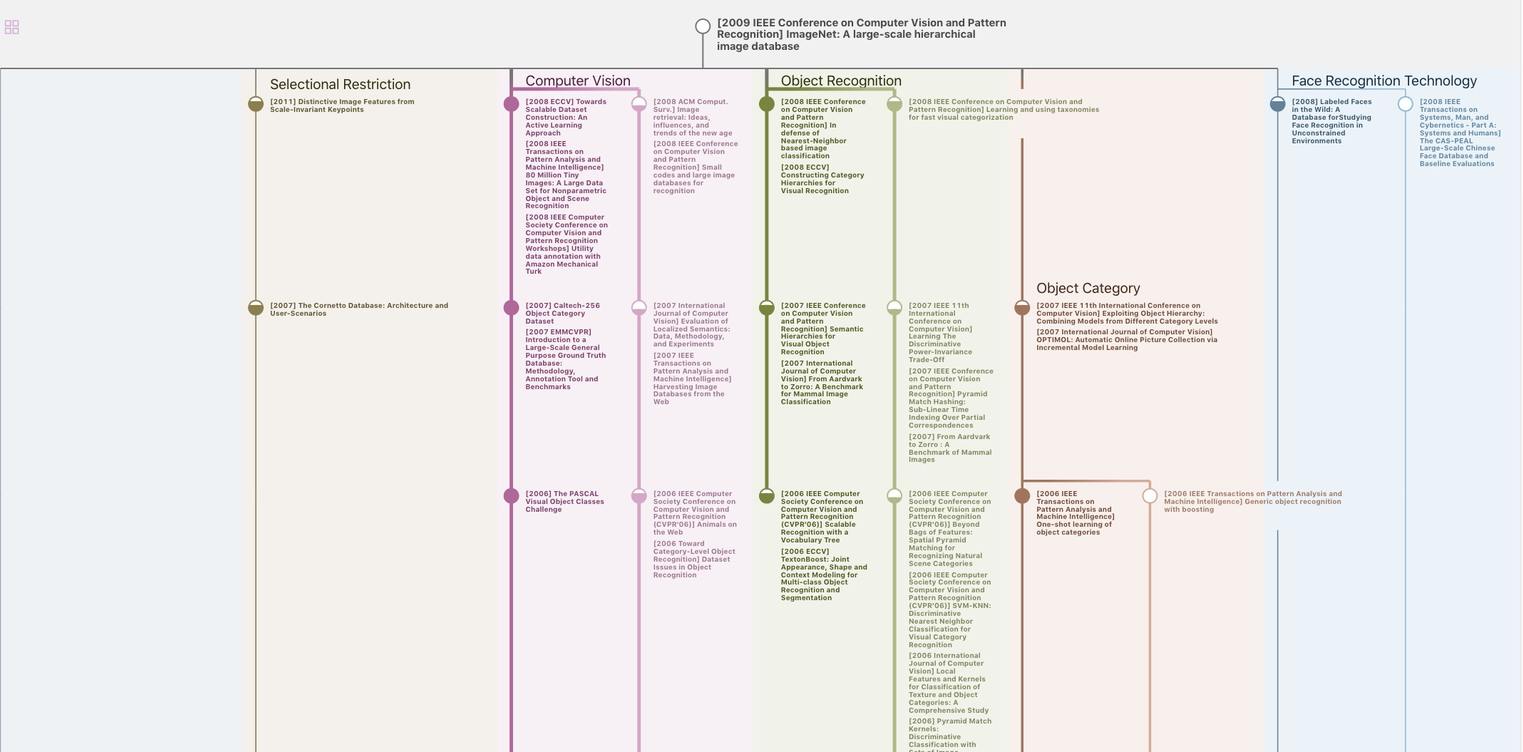
生成溯源树,研究论文发展脉络
Chat Paper
正在生成论文摘要