Genetic programming, standardisation, and stochastic gradient descent revisited
Proceedings of the Genetic and Evolutionary Computation Conference Companion(2022)
摘要
The use of Z-score standardisation to improve the performance of genetic programming is well known within symbolic regression. Additionally, Z-score standardisation is known to be a key element in the effective application of stochastic gradient descent. However, a thorough treatment of genetic programming with stochastic gradient descent (GP(ZGD)) does not exist in the literature. This paper introduces a recalibrated variant of GP(ZGD) and tests its performance within the recently-proposed SRBench framework: the resulting variant of GP(ZGD) demonstrates excellent performance relative to existing symbolic regression methods. Additionally, this paper provides some exploration of SRBench itself and suggests areas of potential improvement to increase the utility of the SRBench framework.
更多查看译文
关键词
genetic programming, symbolic regression, stochastic gradient descent, benchmarking
AI 理解论文
溯源树
样例
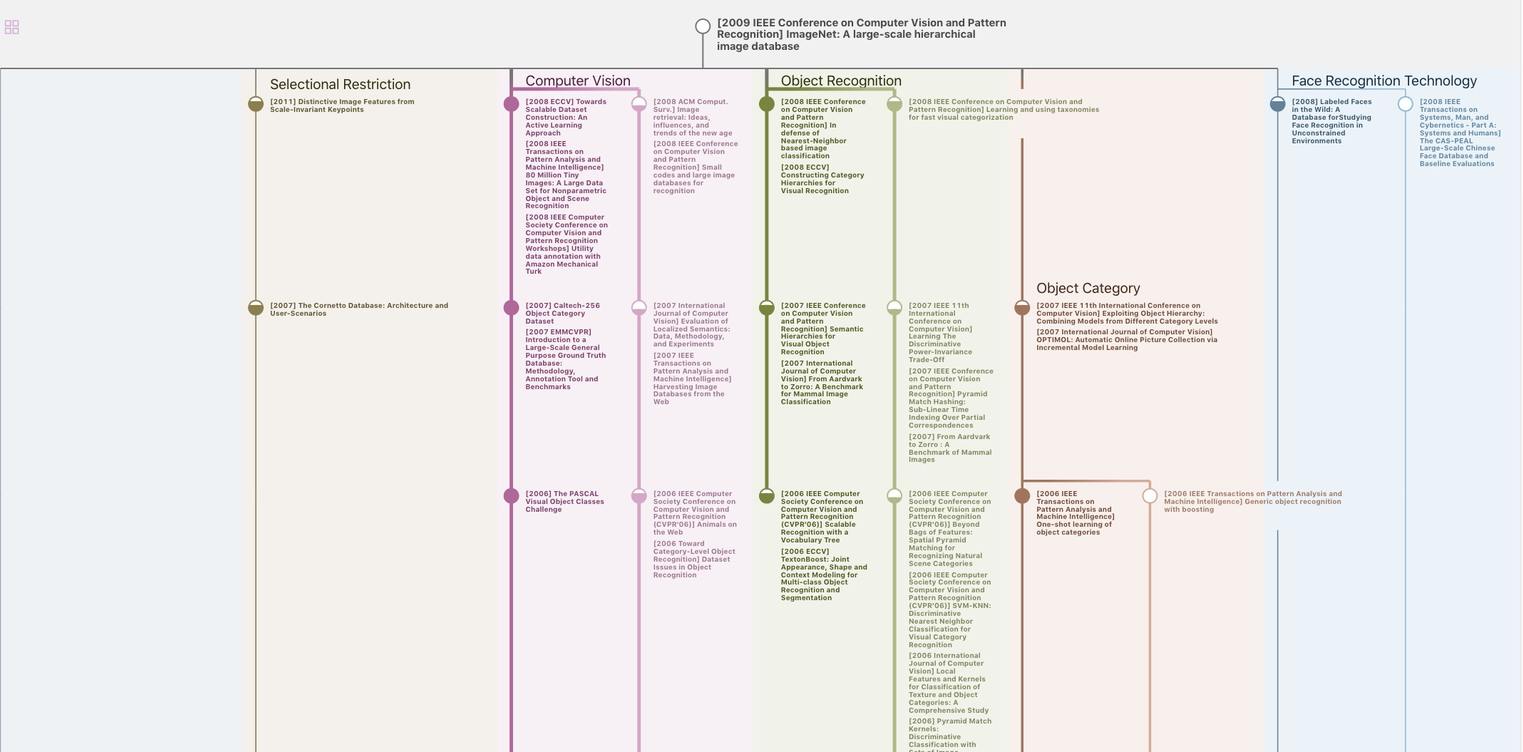
生成溯源树,研究论文发展脉络
Chat Paper
正在生成论文摘要