Dipole Fitting in Unit-Level Spacecraft Equipment with Deep Neural Networks
2022 ESA Workshop on Aerospace EMC (Aerospace EMC)(2022)
摘要
Magnetic Dipole Modeling (MDM) is a well- established method for fitting the spatial magnetic signature generated by magnetic sources into a model comprised of magnetic dipoles. In this paper, we study the dipole fitting problem using Machine Learning (ML) techniques. Simulated data were used to estimate the dipole parameters that can accurately reconstruct the measured magnetic field. We applied our methodology in two widely used measurement facilities, namely the Magnetic Coil Facility (MCF) and the Multi Magnetometer Facility (MMF). To solve this regression problem, Artificial Neural Networks (ANNs) were considered. Simulation results showed that ANNs significantly outperform other benchmark ML methods in terms of the achieved accuracy of the estimated dipole parameters. Finally, we observed that MMF model shows enhanced field reconstruction accuracy with reduced position estimation loss (~70% lower position error), magnetic moment estimation loss (~55% lower moment error) and model complexity (33.3% lower complexity) compared to the MCF model.
更多查看译文
关键词
ANN,magnetic dipole fitting problem,multimagnetometer facility,dipole parameter estimation,MCF model,reduced position estimation loss,field reconstruction accuracy,MMF model,benchmark ML methods,artificial neural networks,regression problem,magnetic coil facility,magnetic field measurement,machine learning techniques,magnetic sources,spatial magnetic signature,deep neural networks,unit-level spacecraft equipment
AI 理解论文
溯源树
样例
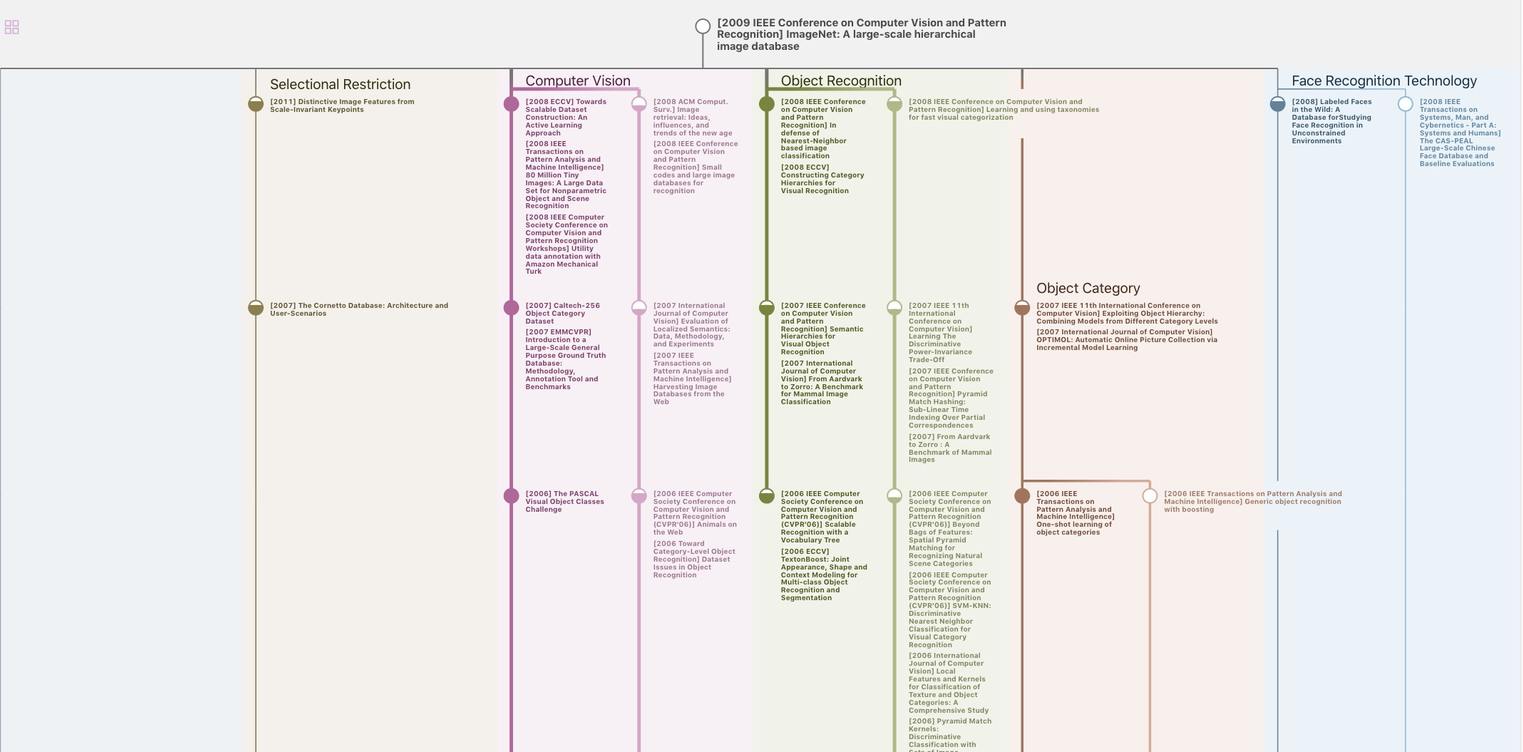
生成溯源树,研究论文发展脉络
Chat Paper
正在生成论文摘要